Chapter 1. Introduction
“Decision-makers only use 22% of the jumble of data-driven insights they receive,” Erik Larson writes in Forbes. What’s going on? This is an especially important question when we think about senior leaders, whose choices are critical to the success of their organizations, and sometimes, the world.
It’s sad to think that this powerful technology is having such limited impact. Technologies define an age: the printing press brought about the Renaissance, the steam engine was core to the Industrial Revolution, and computers created the “Information Age.” We thought that analytics and data would have a similar impact for the better. But, as this book goes to press, the jury is still out.
There was a widespread belief for a while that “more data = better decisions,” but, sadly, we’re not seeing organizations—public or private—that we think are doing better than ever. Some would say things are worse.
What’s going on? Part of the answer is that many organizations are facing unprecedented complexity and volatility from external factors. Globalization means that the corner store has given way to the big-box store, the pandemic has changed how we work and collaborate, and changes in media and climate mean that we’re all getting used to a “new normal.”
In response, many leaders and data scientists have embarked on a digital transformation journey, going from paper-based records to digitized information, then using that information to drive business intelligence (BI) and to train artificial intelligence (AI).
But data, AI, and BI aren’t enough. Leading organizations are recognizing the importance of both collaborative intelligence (CI) and decision intelligence (DI): to integrate the expertise of multiple perspectives to make better decisions, leading to better outcomes. AI/BI/CI/DI: all are needed to reap the benefits of the new age. DI, from this point of view, then, is the “last mile” to realize the transformation vision.
Indeed, you can think of modern organizations as decision factories, using meetings, emails, and more to churn out one decision after another. As Dominik Dellermann, CEO of Vencortex, writes: “Ultimately, the value of your company is just the sum of decisions made and executed.... [The] ability to make faster, more consistent, more adaptable and higher-quality decisions at scale defines the performance of your entire business.”
But the journey to better decision making, as you might expect, includes bumps and obstacles along the road. One common roadblock is that, despite these widespread digital transformations, many organizations are still making decisions without the benefit of evidence and data that are clearly connected to business outcomes—even as the decisions they make are more important than ever.
Core to overcoming this roadblock is a more professional approach to managing the ingredients in a typical decision. How do you integrate them into a well-justified set of actions that (you have good reason to believe) will lead to the outcomes you desire? Within the volatile, complex, and uncertain environment of organizations (commercial and public), this challenge—however promising—can seem overwhelming. As decision-making expert Dr. Roger Moser told us, what’s important is that “people start to focus on designing more effective and efficient decision-making processes, and new technological advancements along the data value chain (including AI/ML, data storage, data lakes, etc.) allow for a new level of professionalism in decision process design.”
The good news is that a little bit goes a long way. In most organizations, people still make important decisions using 20th-century processes and “technology.” Most people don’t see effective and efficient decision-making processes as critical to managing decisions. They don’t think of “a decision” as something that they can create collaboratively, extend, check for “bugs,” reuse, and improve over time.
Indeed, a decision is a bit like a spaceship: it’s something that you create with other experts from different parts of your organization, using advanced technology to reach lofty goals. Just this simple mental shift toward realizing that decisions are like other things we can build and extend can be a powerful step toward solving complex problems.
Do You Need Decision Intelligence?
Perhaps you’ve heard one or more of these questions within your organization:
“I think that our organization’s decisions should be more evidence-based. What’s the best way to do that?”
“I’ve heard that data and AI are transforming organizations, and I don’t want to miss the boat. But where should I start?”
“I feel that we could do better when decision teams get together to make strategy decisions that impact us for many months or years. How can we effectively manage assumptions, uncertainty, and risk?”
“We track certain key performance indicators (KPIs) in our company, and that’s a good start, but it leaves an open question: can’t technology help us decide what to do when the KPIs show we’re in trouble?”
If so, then you need DI. (We’ll have more to say about assessing your particular decision for its fitness to DI in Chapter 2.)
What DI Does for You and Your Organization
In many ways, DI is like other new disciplines that have changed how we work together, like project management, business process re-engineering, or data governance. We know that you’re probably overwhelmed with new technologies and methods, and we don’t want to make your life even harder! We are, however, asking you to join us on a journey—and on a learning curve.
If you’ve been working within a medium-sized or large organization for a while, you might already have started to think about how to fix organizations at a systems level, rather than adding yet another technical silver bullet. DI is that systemic fix: not another gee-whiz, overhyped method, but rather a solidly designed discipline that builds on—instead of replacing—over a century of management innovation.
Most of this book walks you through the nine DI processes that we mentioned briefly in the Preface. Have no fear: they’re not hard! You can learn them one step at a time, and by the end of the book you’ll have one of the most powerful disciplines of the 21st century at your command.
Before we get to the processes, though, this chapter sets the table with some context. We’ll give you an easy on-ramp into DI, describe how to motivate your organization to use DI, and give you a brief glimpse of the discipline’s history and current status. It’s no problem if you choose to skip this chapter and go straight into Chapter 2, where you can roll up your sleeves to get started.
From Data to Decisions
You’ve probably run into a “data-driven” or digital transformation initiative at some point in your career. At the very least, your organization has probably asked, “What can we do with all this data? Can it help us to reach our goals?” Most organizations don’t fully understand how data fits into decision making. Often, data analysts make guesses about the decisions they’ll be supporting without any clear understanding. They apply sophisticated mathematical and AI techniques to gain insights and find patterns and trends, provide charts and graphs that they think will be helpful, and then throw it all “over the wall” to nontechnologists.
Imagine you’re sitting at a restaurant and your waiter brings a bowl of water, a cup of flour, and a bowl of spices. This is how many nontechnologists feel when their data teams show them charts and graphs: this information is just not “cooked” into the form that is “digestible.” Data, insights, even information: that’s like those ingredients. To make the information “taste good,” it needs to show how your actions lead to the outcomes you want. Because that’s how nontechnical people think.
That’s why the core focus of DI is finding the data that helps decision makers to connect actions to outcomes: this is what it means for data to be “well cooked,” because action-to-outcome thinking is the “natural” thought process that decision makers use.
There are three specific gaps between data and decisions in most organizations.
First, we’ve found that many analysts and nontechnologists struggle to reach a shared understanding of how data will be used. What are you trying to achieve: sales, profit, customer retention, population health? What actions do you have available to reach your objective(s)? What can you measure that will help you understand the path from actions to outcomes?
Second, it’s often hard to know where to begin with a new decision-making initiative. The decision context, available actions, and required outcomes are in the hands of the decision maker, who is often not a technical person. The data and models that inform the decision are in the hands of the analysts. How can nontechnologists communicate the decision’s context to the analysts effectively so that the analysts can provide data that connects actions to outcomes?
Third, even when you have accurate data, it can be hard to use it well. To make a solid evidence-based decision, you need to know how your desired outcomes depend on the actions you can take and your external environment. Despite the finest AI techniques, typically your data doesn’t answer the question, “If I take these actions, what will the outcomes be?”
DI is, then, the discipline of converting those “raw ingredients” into the right form. And this book is your recipe.
The Decision Complexity Ceiling
Organizational decision making has reached a complexity ceiling: the factors that come into play when making a major decision are so many and so complex that they exceed human decision makers’ capacity to make the right choices.1 Construction, software, finance, manufacturing, and many other disciplines have faced similar complexity ceilings as they’ve evolved: they all reached a point where the number of inputs, the interactions between them, and the time frames in which results had to be achieved overwhelmed the techniques of the day. Says DI entrepreneur Jazmine Cable-Whitehurst of CModel, “Maybe back in the day, we didn’t need to design decisions for a corner store. But now, businesses have a much greater reach, and they are affected in turn by global factors like climate change, diversity, race, planetary boundaries, and politics: all things that they need to be conscious of today that they didn’t have to think about 50 years ago.”
A 2021 Gartner survey, for instance, found that 65% of organizational decisions are more complex—often involving more stakeholders or choices—than they were only two years earlier. And complexity is exacerbated by volatility: decision making can’t keep up with a fast-changing context. This complexity might include:
- Causal chain length and complexity
The number of elements that make up the decisions, including choices of actions, desired results, dependencies between elements of the decision, peripheral (often unintended) consequences, and long cause-and-effect chains
- Time variation
Factors that change during the decision-making and execution process
- Data
Data that is only partially available, uncertain, incorrect, or difficult to obtain, manage, or interpret
- Human factors
Decision contributors’ differing viewpoints, their levels of skill and experience, and the effects of political and social relationships, to name a few
You must consider so much information, so many choices, so many potential interactions between the two, and such serious potential consequences for bad decisions, that it can be overwhelming. For this reason, organizations end up taking big, unnecessary risks based on justifications that are often far from sound.
In recent years, a knee-jerk answer to complexity has been to simply gather more data, create more models, hire statisticians, or ask the IT department to build an information architecture that allows greater sharing and collaboration. Although these approaches may sometimes be helpful, they are usually not enough, they often add complexity and cognitive overload, and they lack integration between people, processes, and technology. The result is that, in many organizations, systems, data, and human stakeholders are separated by culture, language, geographical distance, and time delays.
The stakes have become too high, and the game is now played too fast, for organizations to rely on intuition and luck. Decision makers need a system that gives them the best chance of winning. DI is a solution to this fundamental shift.
Fortunately, a very effective solution pattern has emerged. Disciplines like construction, software engineering, and systems engineering have overcome the complexity ceiling by developing and widely adopting a methodology that:
Systematizes the tasks required for successful completion and makes them objective (like framing a house)
Defines quality-control checks and balances for each set of tasks (like inspecting a house before occupancy)
Defines a common formal nomenclature that removes ambiguity and facilitates sharing information and knowledge among groups with widely different skills and backgrounds (like having a standard way to draw a picture of a door on a blueprint)
Is supported by tools (like blueprints and computer-assisted design tools)
DI applies these tried-and-true approaches to decision making.
Figure 1-1 shows a simple CDD for a decision: whether to buy regular coffee or bird-friendly, fair-trade coffee. As you can see there, the decision contains actions that lead to outcomes through a chain of events. Those events are defined by intermediates, the steps in the causal chain from actions to outcomes. They also depend on externals, or things beyond your control that affect the outcomes.
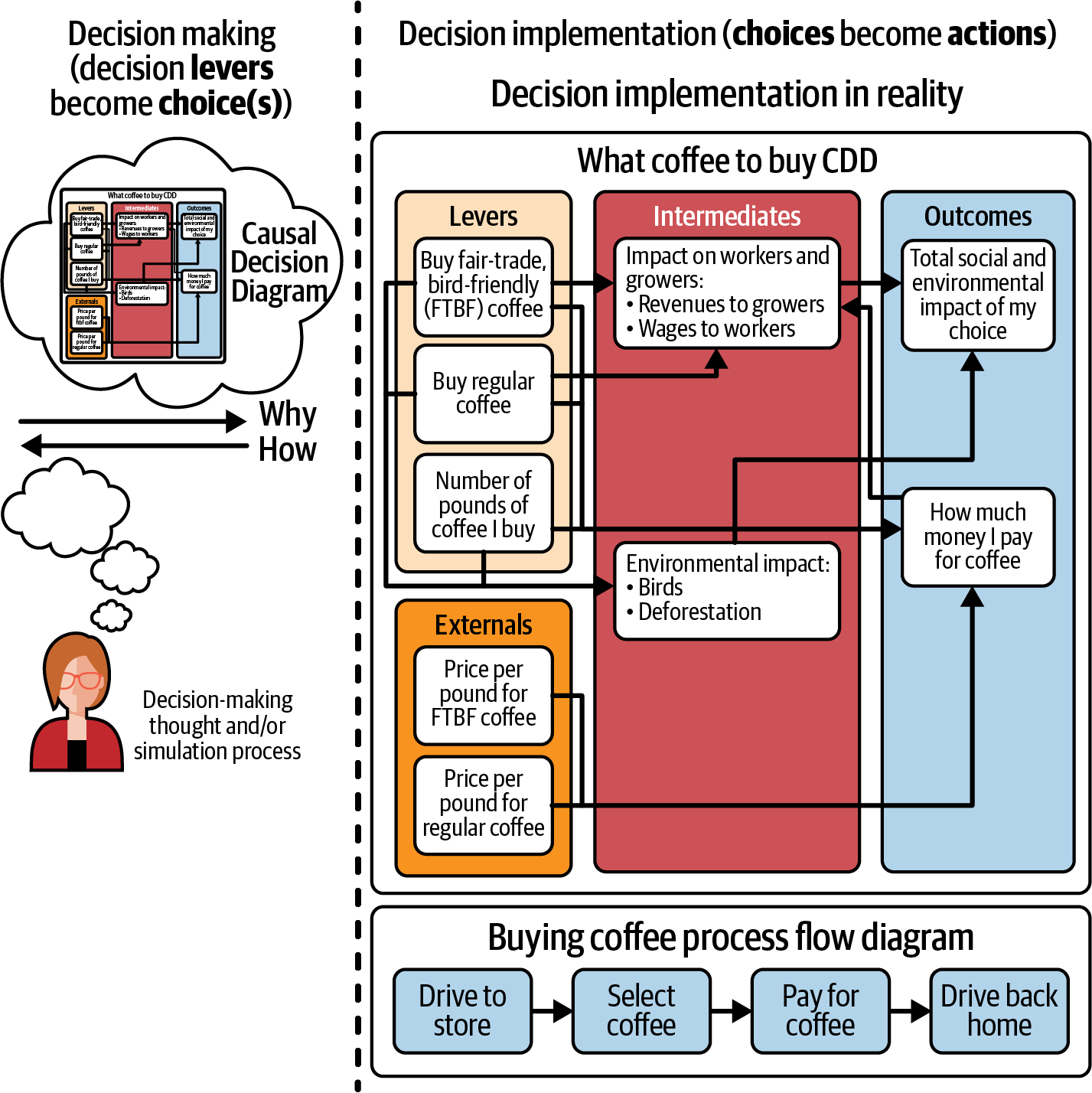
Figure 1-1. An overview of DI, from the point of view of a CDD, distinguishing the decision process from the action process, DI flows from process flows, and technology from nontechnology solutions.
There’s a difference between the process of thinking about a decision (on the left-hand side of Figure 1-1) and the process of actually taking an action based on a decision (on the right). This figure also shows the difference between a process in which a decision is made (drive to the store, select coffee, etc.) and the action-to-outcome CDD (which also uses boxes and arrows, but does not show a process). The right-hand side is an abstraction or representation of the left-hand side. The distinction here is similar to the philosophical argument that “the map is not the territory”.
This book is organized around starting with the left-hand side of Figure 1-1: designing a decision first, taking an action, and then using the decision model to track the action as it plays out over time. For a different approach, you could start on the right, with intermediates that you’re already monitoring: in the coffee example, maybe you have various measures of the deforestation caused by coffee plantations. Then you can structure those measures into a CDD with a focus on the outcomes and leading indicators, working back to the actions over time. This will all make more sense by the end of the book.
DI’s approach to evidence-based decision making starts with the decision, not with the evidence. First you need to understand your decision: the outcomes you want, the actions that you can take, the externals that can affect outcomes, and the causal chains from actions to outcomes. Then you can find evidence: the data, models, and human expertise that provide information about the boxes and arrows on the CDD.
A benefit of the decision-first approach is that you can now safely set aside all information that isn’t relevant to your decision. This is a huge win, because to make the decision at hand, you don’t need to cleanse, vet, or assess any data that doesn’t affect your outcomes.
Your CDD is a tool for integrating multiple pieces of evidence to help you understand how your actions lead to the outcomes you want. This integration is a key benefit of DI. With the addition of evidence, a decision model becomes a powerful tool for reasoning about or simulating your decision. Simply working through a few examples manually can sometimes be enough to show important patterns or illuminate the most effective actions. And when many dollars or lives are on the line and you and your stakeholders have difficulty understanding the decision, it may be worthwhile to develop in-depth software simulations and powerful visualizations to find the right balance between risks and rewards. (See Chapter 5 for more information.)
What Is DI?
In the Preface, we sketched how to design a decision, summarized the elements of the DI, and gave you a brief introduction to the nine DI processes. We’ll tell you a little more here, to set the stage for the remaining chapters, but we expect you’ll still have questions. Your understanding of what DI is and how to use it will deepen with each chapter.
DI is a methodology and set of processes and technologies for making better, more evidence-based decisions by helping decision makers understand how the actions they take today can affect their desired outcomes in the future.
The key concept of DI is the idea that you can design decisions (see Chapter 3). Importantly, a decision, as we treat it here, is a thought process about an action that, once made, leads to specific outcomes without any further intervention. (Contrast this to a process flow—if you’re familiar with those—where you have control over each step.)
Just as organizations design cars, buildings, and airplanes before they build them using drawings and tools, it turns out that you can also design decisions. Much like a blueprint, a decision design helps align everyone involved in that decision—including stakeholders—around its rationale. If you think of creating decisions as a design problem, you can also bring many design best practices to bear, such as ideation, documentation, rendering, refinement, quality assurance (QA), and design thinking. You’ll be documenting all this in a CDD, as we introduced in the Preface.
That might sound like a lot, but an important aspect of DI is that it’s easy to do, at least the early processes.
DI begins and ends with the group or person making the decision. Rather than asking you to think about decisions in a new way, DI simply asks that you document the way that you think about decisions today. You’ll find that just drawing that CDD picture of a decision—as we’ll teach you to do—goes a long way.
Build Your First CDD, Right Now!
This is a long book, and we hope it will be an enjoyable read for you. But, if you’re feeling impatient and want to get started doing DI right now, then you can focus on the simplified DI process shown here. This process is for building a CDD, like the ones in the Preface. We’ll break it down in a lot more detail and give many more examples in the following chapters. But we’ll be honest—and serious—if you just read and implement this one process, today, you’ll be well on your way to better decisions in your organization. (We were surprised, too, when we first started doing it.)
Work by yourself if you are the sole decision maker, or assemble a decision team—ideally a diverse one that includes experts, stakeholders, and the person who will be responsible for the decision outcome. Then follow these steps:
Facilitate a brainstorming session to write down goals/outcomes for a decision (such as, for example, “launch a new product that will be profitable within two years”). (See Chapter 3.)
Select about three outcomes for initial focus. (You can add more in step 8 if you need to.) (See Chapter 3 for more on steps 2 through 8.)
Discuss those outcomes and ensure that the team agrees to them and that they’re precise enough to measure.
Brainstorm actions that could lead to those outcomes (such as, for example, “launch a video course”).
Select about three of those actions for initial focus. (Again, you can add more in step 8.)
Discuss the chains of events that might lead from actions to outcomes. Document them as boxes and arrows, working from left to right on a whiteboard. (While this looks like a flowchart, data flow, or process diagram, the boxes and arrows mean something quite different. We’ll explain more in Chapter 3.)
Review the diagram to ensure everyone likes it.
Add more actions and outcomes to the original sets of three, one at a time, as you see fit. Stop when you think the diagram is complex enough to be valuable, but not overwhelming.
Clean up the diagram and publish it within your organization for review. (Maybe just hang it on the wall. You can even send us a picture if that’s allowed—we’d love to see what you come up with!) Consider writing an explanatory narrative document as well.
Use the diagram to help to support decision conversations.
Send the diagram to your analytics team and ask them how they might provide data, ML models, or other technologies to refine your understanding of how the outlined actions lead to the desired outcomes (see Chapter 4).
Revisit the diagram from time to time to extend it, update it, and modify it as circumstances change.
You would be surprised at the number of decisions that are made without even getting to step 4. Simply aligning around the outcomes you want from your decisions has tremendous power (because—let’s be clear—you make thousands of decisions a day, most of which will never be modeled, and a picture like this can help everyone to be better aligned).
The previously described process is a bridge to treating decisions as designed artifacts. Once you document decisions in this way, you can review, improve, and reuse them and treat them as a scaffold for integrating data, human knowledge, preexisting tools, and more. This process is a jumping-off point for the benefits you can achieve with a deeper understanding of DI, like the ability to make better evidence-based decisions, to better utilize your data stack and AI, and to understand how actions in one silo of your organization impact other silos. If you want practical steps to use this powerful new discipline to its maximum benefit, then this handbook is for you.
DI Is About Action-to-Outcome Decisions
If you’ve been involved in systems that involve decision making, especially if they’re technology systems, then you might be feeling a bit confused about what we mean by the word decision. Not surprising, because this word has overlapping meanings, some of which may be unfamiliar to nontechnical readers. If so, don’t worry about them. They are outside the scope of this book. For everyone else, it’s important to zero in on what we mean by “decision” within DI. Consider the decision types shown in Figure 1-2 and summarized as follows:
- Classification decisions
We sometimes call this “decisions that”: for example, deciding that a particular picture shows a cat.
- Regression decisions (predictions)
Another “decision that”: for example, that there is a 20% chance of rain tomorrow.
- Action-to-outcome decisions
Decisions to take one or more actions to achieve one or more outcomes.2 Here, the actions we’re choosing are irrevocable allocations of resources, as described by Google Chief Decision Scientist Dr. Cassie Kozyrkov.
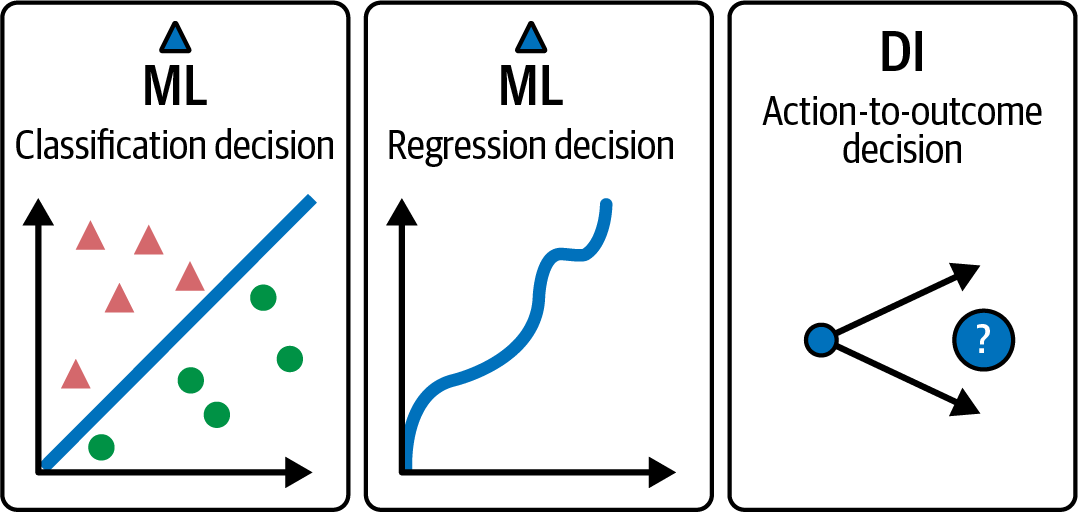
Figure 1-2. Different meanings of the word decision.
This book primarily covers the third category: decisions about actions that lead to outcomes. In the history of data, statistics, and AI, the first two categories—classification and regression decisions—have received the lion’s share of attention while action-to-outcome decisions have received short shrift, despite how core they are to how people think. DI fills this gap.
Here are some examples that fit this actions-to-outcomes pattern:
A teacher deciding whether to offer a course online or in person
An employee deciding whether to travel to a conference or attend virtually
A facilities manager deciding how and when to reopen an office building at the end of a pandemic work-from-home period
A citizen deciding to which charity they should donate, along with when and how much
A human resources department deciding on the details of a diversity hiring policy
A farmer deciding on the best pest-management option for their crops
A legislator deciding what elements to include in a bill that would ensure equitable access to broadband for all citizens
A doctor comparing potential treatments for a patient
What all these examples have in common is that someone wants to achieve, or is responsible for achieving, one or more outcomes and has the authority to take one of several actions to achieve those outcomes. The decision maker wants to know which action(s) will produce their desired outcomes.
The best decisions to address with DI are ones for which at least one of the following is true:
Someone in your organization cares about the decision.
The decision has a big enough impact on you or someone else that you believe it deserves careful thought.
You think that the decision could be made in a better way.
You think that some data might help improve the decision.
Note that you are not required to have a lot of knowledge about the decision. Indeed, sometimes organizations are working “in the dark,” making decisions about entirely new circumstances. These situations can be a good fit for DI, which helps to bring together the best minds and technology in novel situations. On the other hand, DI is not just for big, one-of-a-kind strategic decisions. DI delivers repeated value in tactical decisions, which are often the decisions at different steps in organizational business processes.
DI Is About Human-in-the-Loop Decisions
Another dimension we use to classify decisions is the degree to which they involve humans, AI, or both: that is, automated, hybrid, or manual decisions.
The DI methodology described in this book applies to decisions made by people (usually with support from technology) to take one or more actions to achieve one or more outcomes. Fully automated decisions, such as Amazon’s product recommender, are not core to this methodology—though it turns out that DI is very useful for them, too! Simply put, DI helps organizations to make better decisions by combining the best of human expertise with all sorts of technology.
The AI world doesn’t always pay much attention to decisions that include a human in the loop: a person who consults some resource—maybe data charts and graphs—before making a decision. We think that’s because a lot of the big AI “wins” over the years have been in fully automated use cases, such as Facebook and Google advertisements. This is another gap that DI fills. DI falls into the category of hybrid—also called augmented—decision-making methods, those that involve both humans and AI, which McKinsey notes constitute one of the most rapidly growing technology markets right now.3
You can have automated, hybrid, or manual decisions in each of the three decision categories: classification, regression, or action-to-outcome. This book, and our work, focuses on hybrid action-to-outcome decisions. Because other methods don’t cover them, we’ve been asked to address hybrid action-to-outcome decisions over and over again.
AI models can make valuable single-link decisions, like what cross-sell item to suggest or what ad to display. DI lets you use multiple AI models together to inform a decision, and integrate AI and other technologies with human expertise and human judgment in your decision making. If you put action-to-outcome decisions together with human-in-the-loop decision making, you get a set of desperately needed capabilities that are new compared to standard AI and analytics methods. We hope the value this provides will motivate you to keep reading this book and to embrace DI in your work.
The core of DI is the idea that you can design these action-to-outcome decisions the way engineers design cars, buildings, and airplanes before building them using drawings or tools. Just as a blueprint helps many collaborators to build a skyscraper, a design of a decision helps to align everyone involved—including stakeholders—around its rationale. And if you treat decisions as a design problem, you can also bring to bear best practices from the field of design, such as ideation, documentation, rendering, refinement, QA, and design thinking. Under the hood, DI is all about integration and connecting human decision makers with data, AI, complex systems modeling, human behavior, and many other disciplines. Simply put, DI helps organizations to make better decisions by combining the best of human expertise with all sorts of technology.
Why Data-Driven Decision Makers Need DI
Many decision makers want to go beyond improving manual decision-making methods and learn to use data and technology more effectively. You might argue, even, that a modern organizational leader is behind the times if they’re relying only on “gut instinct” in making important choices. This is why so many organizations strive to be “data-driven” and “evidence-based.”
Time and again, we’ve seen technical analysts open a meeting on a new project by saying, “Here are the data and AI models we have for you.” This approach is back-to-front: how can the analysts provide the right information before they know what their “customer” (from outside the analytics department) needs? Sorry to say, they are usually only guessing at how decision makers will use their data and information. They talk about what data and charts are “interesting” and where are the best “insights,” without really understanding how any of it translates into actions and business outcomes. Without understanding the cause-and-effect chains that link actions to outcomes and what data informs those chains, technologists will usually gather and process more data than you actually need, as we described at the start of this chapter. Preparing data that is not useful is likely to take too long and entail a lot of unnecessary work.
There is a better way, and it begins by starting the conversation in the right direction. Consider a closely analogous situation. When an IT organization needs to create software that solves a problem, the first thing they are given is a list of customer requirements. The interaction between data science and analytics teams and their customers should begin the same way. The analytics customer should be able to say, “Here’s what I need you to do for me” in a language that both technical and nontechnical people understand.
So why is there such a difference between data projects, where the tail seems to be wagging the dog, and software projects, where the deliverable is defined by those who have the problems that the software is intended to solve?
The reason is that software engineering has spent years, if not decades, recognizing that software is only successful when it meets end users’ needs. The discipline thus developed formal and informal methodologies for eliciting requirements from users, stated in ways that nontechnical users can understand and that software developers can use to build systems that meet users’ needs. This is the first stage of any software engineering project.
Decision makers need “requirements” tools to reap similar benefits. DI is starting to bring this same kind of maturity to the world of decision making. But software requirements alone won’t cut it, because decisions are quite different than software applications. In Chapter 3, we show you how to design a decision so that you can see what data you need. Then, in Chapter 4, we show you how to link the data to your designed decision.
Erik Balodis, director of analytics and decision support for the Bank of Canada, illustrates in a Medium article why DI is needed, pointing out “a recognition that investing in data to ‘make better decisions’ is too vague.” He also describes a pattern he’s perceived within organizations as:
a desire to improve decision culture and to mitigate the risks inherent in unstructured or ad hoc decision-making based principally on heuristics; a desire to see decision intelligence (DI) as a unifying discipline, bringing together much-needed influence from a variety of social sciences, quantitative methods, and business concepts.
Balodis also mentions “a range of very business-sounding reasons,” including “data and analytic investment optimization; optimized design and re-use of data, analytics, and decision products and artifacts; [and] driving focus in decision-support activities to enable business strategy and oversight.”
DI offers a practical methodology that tackles two problems that are widespread in data-driven decision making.
- Problem 1: How can you and your data science team identify the data you need to support your decisions?
You need to know how the outcomes you are trying to achieve depend on the actions you can take. But rarely is data available that directly relates actions to outcomes. While you can often determine this relationship from data, how can you know which data to use and how it relates to the decision you need to make?
DI gives you a systematic method for using a decision statement to derive requirements and specifications for the data science and analytic work required to support your decision. The core of DI is decision modeling, or creating a CDD (or “decision blueprint”) and adding existing data and models to it. (See Chapters 3 and 4 for more details.)
- Problem 2: How can you use your preexisting technology and human investments to find actions that lead to the outcomes you want?
Action-to-outcome decisions are based on this question: “If we take this course of action, what will the outcome be?” Historically, there have been a number of technologies to answer this question, including linear programming and the field of operations research. DI adds a new tool to the toolkit, simulation: the process of using your brain or a computer to see how different actions affect your outcomes. (We’ll cover simulation much more in Chapter 5.)
Where DI Comes From
Unlike AI and data science, DI was born in the commercial world and takes a problem-first—not a solution-first—approach to bridging the gap from stakeholders to technology. DI begins and ends with the decision customer, starting from defining the problem or goals and ending with the solution, something that can be (but doesn’t have to be) very sophisticated “under the hood.”
Mark Zangari and L. Y. Pratt, a coauthor of this book, invented DI in 2008 based on hundreds of interviews with decision makers. We originally called it “decision engineering,” but later renamed it “decision intelligence” to make it clear that DI is more than simply a technical field. While the term decision intelligence appeared in print as early as 1994, the DI methodology in this handbook originated with Pratt and Zangari’s 2008 whitepaper (Pratt and Zangari were not aware of, nor did they build on, the previous meaning of this phrase).
Our 2008 research asked an ambitious question: “If technology should solve one problem for you that it doesn’t solve today, what would it be?” Surprisingly, we heard a consistent answer: “Help me make better decisions, using data and other emerging technologies.”
L. Y. Pratt’s Link: How Decision Intelligence Connects Data, Actions, and Outcomes for a Better World was the first book on DI. It provides a beginner’s introduction to DI best practices and describes how DI projects worldwide have saved and generated many hundreds of millions of dollars of value, as well as nonfinancial benefits. You don’t need to read Link before this book, but you might find it an interesting and less technical overview of the field.
Link generated a new wave of interest in DI, with the Gartner Group including DI in its “Top Ten Trends” reports for 2020, 2021, and 2022. However, we have found that Link needs a practical guide as a companion volume. This handbook is that guide. It is loosely based on a source book we wrote in partnership with a G20 central bank as we implemented DI across that organization.
Another important DI thought leader is Dr. Cassie Kozyrkov, Google Cloud’s chief decision scientist, who writes that DI is “a vital science for the AI era, covering the skills needed to lead AI projects responsibly and design objectives, metrics, and safety-nets for automation at scale.” Kozyrkov defines DI as a blend of behavioral economics and psychology, data science, statistics, and decision science. We agree with this multidisciplinary approach: Kozyrkov’s “unified field” definition of DI correctly includes statistical rigor as well as both “hard” and “soft” decision-making factors.
DI is not the only discipline designed to support better decisions. Others include business intelligence, decision support, knowledge management, balanced scorecards, KPIs, data visualization, data science, business process modeling,4 and decision analysis.5 Many organizations have found that these practices can be very helpful, but for decision making in complex circumstances, they don’t go quite far enough. And DI builds on them; it doesn’t replace them.
An analogy best illustrates what’s happening. The world had effective airplane technology long before it had flight simulators. To learn to fly, you had to watch a pilot in real life, then take the controls yourself. That was dangerous! It’s much better—and much safer for pilots and passengers alike—for student pilots to learn from challenging scenarios within flight simulators many times before taking the controls of a real airplane.
DI is like that: it introduces a “simulation” environment to decision making. It pulls other technologies together to fundamentally change the experience and safety of “flying”—making organizational decisions. You can try things out and “crash” in simulation instead of reality, which is a lot more cost-effective. This approach integrates effective preexisting data and AI technologies and makes using them easier and more natural for human decision makers. By doing so, it helps to solve complex, previously unsolvable problems.
Finally, DI is an approach to what some call neurosymbolic computing, which integrates the historical symbolic (words and logic) world of AI with more modern data-centric approaches. Those of us who have been around the AI block a few times aren’t married to one or the other of these approaches, but rather see DI as an approach to integrating them for the best of both worlds. James Duez, CEO at Rainbird.AI, says it best:
Throughout the years, the field of AI has experienced remarkable shifts. While symbolic AI dominated the 1980s, today the focus has shifted considerably towards data science and machine learning, valuing predictions from data and insights over symbolic models of human knowledge. As we look ahead, the future of AI will be shaped by a balanced integration of these approaches, capitalising on the merits of both symbolic reasoning and data science and machine learning through decision intelligence.
What DI Is Not
There are a number of disciplines that are often confused with DI. Let’s take a quick look at them to clear up the differences.
DI is not process modeling or project planning
Process modeling is the practice of agreeing to a sequence of steps to achieve some goal. A related area is project planning. Both often use boxes and arrows to represent tasks and dependencies, respectively.
Here’s a way to understand the difference between process modeling/project planning and DI: think about the difference between choosing a price for a product and implementing that pricing in some software. These are fundamentally different tasks. Choosing the price depends on your model of the chain of events that leads to your revenues, such as the demand curve that determines how many people will buy your product, as well as external factors like the economic climate and your competitor’s price.
In contrast, setting up your website to charge $20 per month for your product involves a very different set of activities that might be better captured in a process diagram or project plan. That sequence of steps might consist of updating two fields, changing a coupon, and then testing that the payment system still works. Each of these steps could be represented as a box in a diagram, with arrows between them to indicate their relative order. This is not DI, but it interacts with DI at the point where you choose to charge $20 per month.
DI is not a decision tree
A decision tree is a sequence of questions to ask to reach some conclusion. For instance, a doctor might use a decision tree to diagnose a patient with heart disease. The tree might start with “measure blood pressure,” then “if the blood pressure is greater than 140 systolic, then give the patient a blood test,” and so forth. At the end of the question sequence, the decision tree might say, “this patient does not have heart disease.” Note that the decision tree leads the doctor through a series of questions and measurements. The decision tree determines which measurements to take in what order, but it does not show any cause and effect.
A decision tree is fundamentally different than a CDD, because the boxes in a CDD represent a cause-and-effect chain between actions and outcomes. It might tell you that “if you charge this price,” combined with “if you market to this demographic,” then “this many people will be interested in your product,” which, in turn, might cause “this many people to buy your product,” which, in turn, might cause “this amount of revenues in this fiscal year.” As you can see, this is a representation of a chain of events set in motion by your action, not a list of tests or questions to answer to reach a decision.
DI is not data flow
You might also see lots of boxes and arrows in a data flow diagram (also called a data pipeline). There, depending on how you do things, the boxes might represent data in a particular format or location, and the arrows might then represent how that data is changed to a new format or location. Modeling how data flows is not the same as modeling how a nondata causal chain might play out in the world: data flow happens inside a computer, and causal chains happen outside a computer.
What can get confusing here is that you might use data flow to simulate events that happen outside of a computer, as we’ll talk about in more detail in Chapter 5. The key difference is that data flow can be designed however you like, but causal flow should represent some noncomputer process: the computer is only a simulation of the flow from actions to outcomes, even though you might use some sort of data to simulate that.
DI is not about “decisions that”
Something to keep in mind: the word decision is a bit overloaded. We can use it to mean human or automated “decisions that” or predictions. For example, we might decide that a particular picture shows a cat (classification) or predict that there is a 20% chance that a user will click on a button (regression). In DI, the word decision has a third meaning: a choice to take an action with the intent to lead to an outcome.
DI is not operations research or linear programming
For some decisions, you can use math to determine the best action to lead to your outcomes. If so, then the mature and powerful discipline of operations research has great answers for you (formally, operations research focuses on analytical methods in contrast to DI’s numerical focus). But the vast majority of decisions aren’t amenable to this approach, so we need something different. If you’ve got some math that works for your decision, then we say to go for it! But if not (and especially if math is not your thing), then this book is for you.
DI is not decision analysis
Although they’re closely related, DI and decision analysis (DA) are not the same. Here’s how Link describes the difference:
With an over-30-year history, the field of Decision Analysis (DA) covers the philosophy, methodology, and professional practice for formally addressing important decisions ... often in complex situations where there are multiple objectives and decisions must be made under uncertainty.
DA has a considerable overlap with DI, but with a particular focus: providing tools and techniques for teams and leaders to formalize and structure high-value decisions in complex situations. It is less technology- and data-focused than decision support, business intelligence, and DI, all of which go beyond decision making to provide tools that are used continuously in an organization.
Although it was invented independently, you can think of DI as a natural descendent to the important discipline of DA. DI adds technology integration, simulation, data, and AI to the picture. It stands alone, but if you really want to be an expert decision maker, we recommend you take a look at a few DA books as well.
The Decision Framing process of Chapter 2 gives more details about how to decide if your decision is right for DI.
The DI Maturity Model
The DI Maturity Model in Figure 1-3 captures a number of trajectories by which organizations improve their adoption of DI over time.
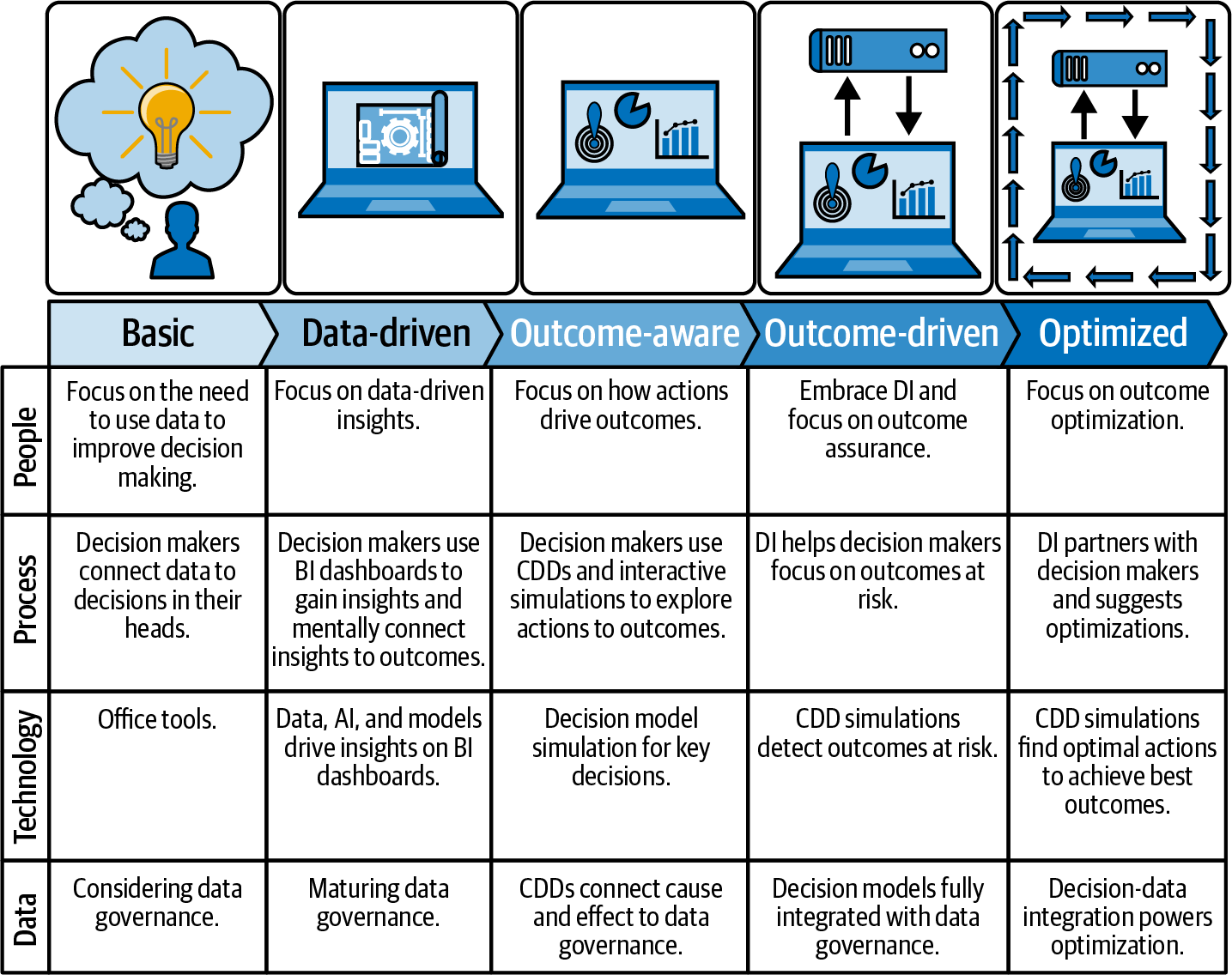
Figure 1-3. The Decision Intelligence Maturity Model, version 1.3.
Like Capability Maturity Model Integration for software or ISO/IEC 15504 for processes, the DI Maturity Model is a roadmap for improving how an organization does DI. It shows the attributes of organizations at different maturity levels. It does not, however, tell you how to “personalize” the maturity levels so that they work within your specific organizational context or how to recognize whether your organization is ready to start moving up a level. No book can do that! Look instead to the same HR, change management, or organizational development people who can help you communicate the value of DI. They can also help you bring your organization to a higher DI maturity level.
The Shifting Meaning of “Decision Intelligence”
As is common in many exciting new fields, a number of organizations—especially technology vendors—have started to use the name “decision intelligence” with inconsistent meanings. Some of these companies use “DI” in a way that is consistent with our approach (and Google’s), while others have a strong focus on technology but not people or processes.
This handbook seeks to correct that imbalance. To realize the maximum benefit of this important new discipline, all three “legs of the stool” must be included: people, process, and technology.
Who Is Doing DI Today?
DI is being applied in many domains, including agriculture, telecommunications, government, health care, climate, space, energy, earth science, and venture investment. As covered extensively in Link, there is a growing DI community of practice: people and organizations who are passionate about DI and are working to improve the methodology and develop tooling. There is a vibrant market of DI vendors and organizations around the world, many of whom are graduates of DI courses. Many of them fall into the Decision Intelligence Service Provider (DISP) category: experts within specific verticals/decisions that use DI to bring their products to market. Companies include Data Innovation.AI, which uses DI to help buildings make decisions about employee health, CModel, IntelliPhi (recently acquired by a large management consulting firm), SatSure, and C-Plan.IT; the Decision Intelligence Tokyo meetup group; and companies like Astral Insights, Diwo, Pyramid Analytics, Tellius, Peak, Rainbird.AI, and Aera. Decision Intelligence News covers the discipline as well. And Trillium Technologies is working with Oxford University and the European Space Agency to bring DI to important problems of climate stewardship.
As any discipline matures, certifications (like the PMP for project managers or the Six Sigma belt system for quality managers) become important for recruiting and consulting. A number of DI certification programs are currently in development.
The Nine DI Processes
The core of this book is a nine-part DI process model, shown in Figure 1-4.
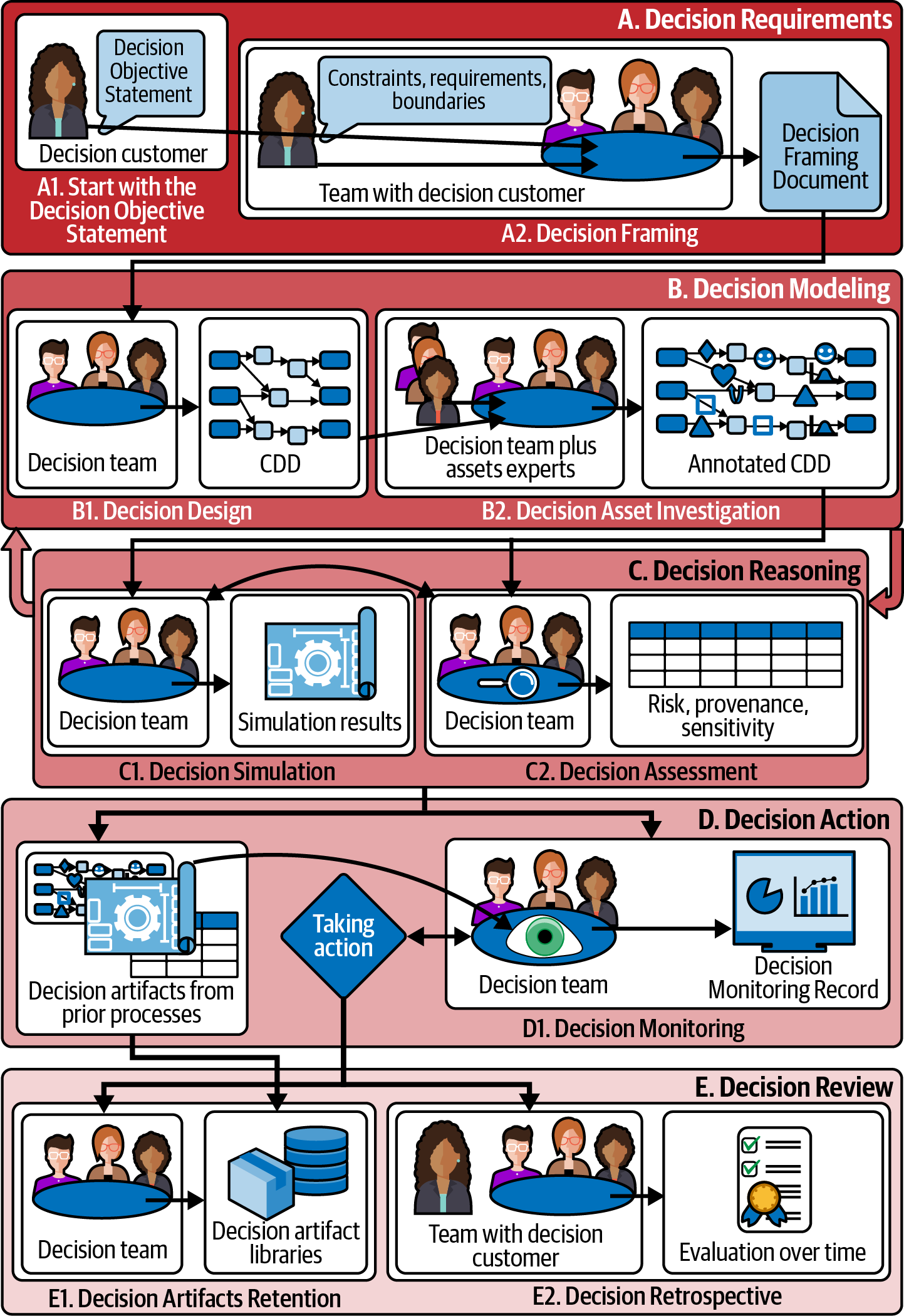
Figure 1-4. Overview of the nine DI processes.
The nine processes are organized into five phases, A through E, that group closely related processes together, with one or two processes per phase. We introduce the phase and the processes in it, explain the terminology and concepts, and then provide the tools you need to execute the processes: process descriptions and the worksheets that document process deliverables. Some phases are covered in a single chapter, while others require two (one per process). Throughout the book, we include many use-case examples and “Try It Yourself” exercises, showing you how to perform and document the processes in that phase and allowing you to practice what you’ve learned.
Chapter 2 covers Phase A, the Decision Requirements phase, which establishes expectations for the deliverables of Decision Modeling. In Process A1, Decision Objective Statement, the decision customer (the person who has the authority and responsibility for the decision) provides a brief description of the decision to the decision team (or individual) tasked with executing the DI processes for that decision. In Process A2, Decision Framing, the team works with the customer to understand the decision requirements and constraints and record them on a worksheet or Decision Framing Document.
Chapter 3 begins Phase B, Decision Modeling, focusing on Process B1, Decision Design. Decision requirements are a prerequisite to effective decision modeling, but modeling is where you build value. In the Decision Design process, you create your initial CDD, the “blueprint” for your decision.
Chapter 4 covers the second process in Phase B: Process B2, Decision Asset Investigation. In this process, you identify the existing data, models, and human expertise that inform the decision and add these decision assets to your CDD.
Chapter 5 begins Phase C, Decision Reasoning, where you’ll use your CDD to understand the system behavior and risks associated with your decision. Process C1, Decision Simulation, lets you understand the behavior of the cause-and-effect system underlying the decision: the mechanisms by which actions lead to outcomes.
Chapter 6 continues Phase C with Process C2, Decision Assessment. This process helps you manage risk.
Chapter 7 covers Phase D, Decision Action, when you (or the decision maker) execute one or more actions. These actions are based on your CDD and the supporting documentation you created in prior processes. They set off the cause-and-effect chains that eventually lead to outcomes. Process D1, Decision Monitoring, allows you to monitor, manage, modify, and correct the decision causal chains as they play out, to achieve the outcomes you want.
We end with Chapter 8, on Phase E, Decision Review. This phase lets you improve your decisions over time and identify avenues for improvement. Process E1, Decision Artifacts Retention, preserves all the valuable information (or decision artifacts) from your well-documented decision so they can serve as starting points or reusable building blocks for future decisions. Process E2, Decision Retrospective, is the final process. It lets your team reflect on and learn from the current decision. Here, you’ll ask whether your decision process was sound, then determine possible improvements to your process, information, knowledge, and/or model.
Conclusion
If you’ve taken the time to read through Chapter 1, you’ve got a good understanding of where DI comes from, how it fits in with other disciplines, and in particular what DI is not. By now, you’re probably hungry for the details of how to do DI. Good, because that’s the main point of the book. Let’s start with Chapter 2, which guides you through the start of your DI journey.
1 L. Pratt and Mark Zangari, “Overcoming the Decision Complexity Ceiling Through Design”, December 2008, Quantellia; L. Pratt and Mark Zangari, “High Performance Decision Making: A Global Study”, January 2009, Quantellia.
2 Note for experts: of course a choice to drive your car, take the bus, or walk to work is, strictly speaking, a classification decision. But we don’t usually use statistical or ML regression or classification for this kind of choice. At heart, the difference is how we go about making that choice: using historical data (classification and regression) versus mechanistic reasoning and simulation, over an understanding of the world (DI’s action-to-outcome decisions).
3 Increasingly, even fully automated decision-making projects are using DI methods to align technical teams around decisions. Although it isn’t the main focus of this book, there is substantial material to support fully automated use cases starting in Chapter 3. This is especially true on projects that need to combine multiple AI models in a process called AI orchestration.
4 S. Williams, “Business Process Modeling Improves Administrative Control,” Automation, December, 1967, 44–50.
5 Ronald A. Howard and Ali E. Abbas, Foundations of Decision Analysis, Global Edition (Boston: Pearson, 2015).
Get The Decision Intelligence Handbook now with the O’Reilly learning platform.
O’Reilly members experience books, live events, courses curated by job role, and more from O’Reilly and nearly 200 top publishers.