Chapter 4. LLM and Generative AI’s Patient and Clinical Potential
The latest AI design paradigms—encompassing agentic reasoning and vastly expanded context windows in LLM and generative AI prompts—will fundamentally change the nature and functions of healthcare applications. While revolutionary, the next generation of AI systems will not transform healthcare fundamentally overnight.
Some of the principal change drivers, however, will likely consist of startups and other tech companies. Many of these aren’t as encumbered by the inertia and risk aversion that can be found across large healthcare companies, and they may be the kind of early adopters that new technologies with yet-to-be-proven return on investment are hungry to find. On the other hand, traditional healthcare incumbents will likely be more cautious, likely jumping into AI when and if these kinds of technologies prove themselves in the marketplace and are seen to be safe and financially sound.
Yet, ironically, as tech multinationals and startups create a critical mass of diverse successes by infusing AI into healthcare, these positive results will filter out to much of the wider field. With returns beginning to accrue, healthcare companies will move toward AI solutions that help them deliver better care while remaining competitive. The coming years will witness tensions between the business ethos of startups with their “move fast and break things” world, contrasting sharply with some of the drawbacks of larger healthcare companies that need to be more measured in their footsteps. Cooperation and competition will play out between them, from which the future of healthcare is bound to emerge, as applications of AI assist in increasing new quality standards for patient outcomes and overall efficiency and personalization of healthcare services.
Patient Experience
Patient experience refers to patients’ broader perceptions and feelings during their interactions with the healthcare system. It encompasses everything from scheduling appointments to interacting with staff and the physical environment to receiving care. It focuses on the emotional and psychological aspects of the journey, including factors like respect, communication, empathy, and overall satisfaction. Arguably, many healthcare systems and applications need to focus on the patient experience, and this is where LLMs can make a huge difference and improve patient care. Patient-centered care that prioritizes a positive healthcare experience delivers inherent value with cascading benefits beyond perception alone. Studies confirm patient satisfaction ties directly to critical downstream results such as treatment adherence, engagement in managing health, and ultimately clinical outcomes.
The use cases described in this section focus on apps that improve patient experience. LLMs and generative AI will improve patient experiences in several ways. LLMs can generate customized explanations of complex health topics, procedures, and medication issues to each patient’s preferences, learning styles, and background. LLM and generative AI chatbots, voice assistants, and avatar interfaces allow 24/7 support to conveniently schedule appointments and connect medical records, get answers about billing questions, and get help with other hassles.
LLMs can provide care continuity by reviewing longitudinal records, prompting patients and providers to follow up on open items, and revisiting unresolved symptoms across past visits to prevent patients from “falling through the cracks.” Emotion-designed LLMs actively listening and responding empathetically to patient contexts beyond medical history alone can make interactions more supportive. LLMs can automate administrative and clinical documentation as well as paperwork to allow providers to focus more on face time with patients and to reduce wait times.
LLMs and generative AI introduce opportunities to systemically streamline coordination hassles and synthesize personalized insights that help patients feel understood. The AI can communicate with patients at their own pace, using language they understand, which may lead to better engagement in their own health management. The future offers brighter, barrier-free patient experiences. Next, we describe a few examples of future emerging apps ushering in this new patient experience.
Health Bot Concierge
Imagine a healthcare system where patients receive personalized support, understand complex medical jargon, and quickly navigate administrative hurdles. This is the future promised by generative AI conversational chatbots, empathetic AI companions, and a breed of intelligent assistants poised to revolutionize the healthcare landscape. These bots—powered by deep learning, LLMs, and natural language processing (NLP)—hold genuine conversations with users, understanding their questions and responding with contextually relevant, human-like language. Their applications extend beyond simple customer service, transforming first-line triage, chronic disease management, and mental health support. Health bot concierge, illustrated in Figure 4-1, will be one of early use cases realized with LLMs and generative AI.
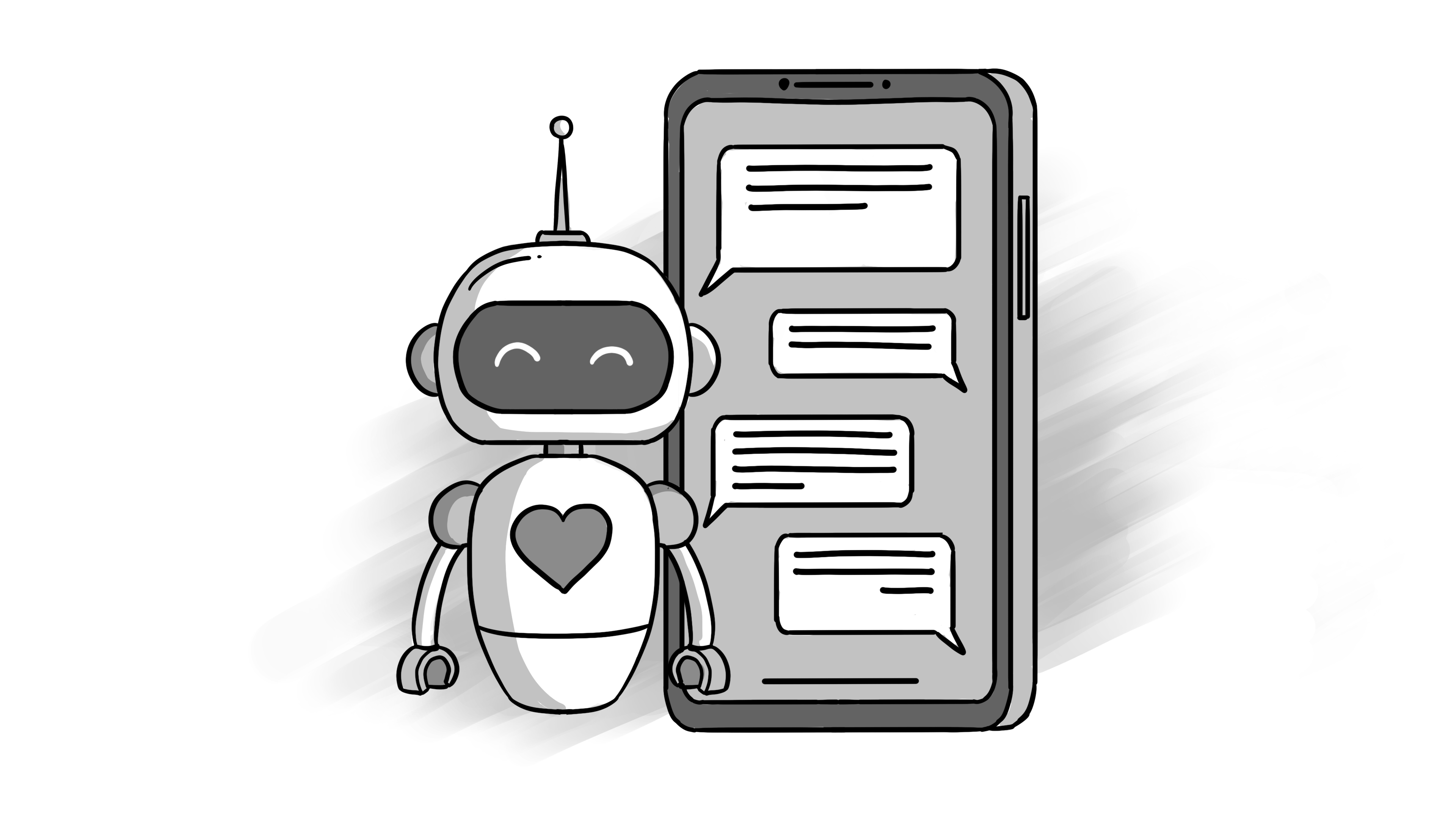
Figure 4-1. Health bot concierge
At the low-acuity end of the scale, a chatbot could act as a triage consultant for a patient at home who could have their symptoms discussed in order to identify the best course of care. Ever wonder what the future of triage will be like? Instead of having to endure waiting rooms filled with patients with runny noses, we could discuss our sore throats with a chatbot who might suggest some soothing honey and lemon or recommend calling for a virtual consultation with a doctor instead.
Beyond triage, algorithms could start as virtual personal health coaches, assessing information gathered from wearable devices or postsymptom questionnaires, and recommending a daily routine or a pill schedule based on personalized suggestions on how to improve mood and alertness, sleeping patterns, and pain. Think of a chatbot sending the patient daily health indicators. It could send you a reminder, a gentle nudge, to take your insulin within the hour if a blood glucose reading crests, or she could prompt you to answer a “How are you feeling today?” The chatbot might inquire with an idea for a few stretches to calm your mind at bedtime, resulting in some fresher and longer z’s.
For patients with chronic health problems or with mental health issues, these chatbots can provide unparalleled support. For example, imagine you receive daily encouraging messages from a wellness AI coach that checks your emotional wellness, gives you motivational quotes, etc. Chatbots for the elderly can be a source of social interaction and mental stimulation and also help to prevent and treat depression, which is more common in the aging population.
There are benefits to staff, too. Generative AI chatbots have the potential to close the gap between doctor and patient; some illnesses are so complex that, if you trust your doctor, it might be helpful to have the chat rewritten in simpler language. You won’t have to wade through medical jargon and wonder what your doctor means by this or that, or later try to dredge up what he said you should now remember to do. Imagine the chat summarized the information, emphasizing the important takeaway words in boldface, making the next steps understandable. You leave the room with complete understanding.
The administrative tasks that most patients dread can be transformed, thanks to the increasing use of chatbots. There are still many horizons for using generative AI chatbots in healthcare, which will only increase as the algorithms learn and improve. The whole paradigm of personalized, accessible, and affordable healthcare will change, with the patient holding the steering wheel. The conversational revolution is here—healthcare will never be the same.
Doctor’s Notes and Visits
One of the most common patient frustrations includes reviewing doctors’ summaries of diagnoses, prescribed medications, and recommended care. Figure 4-2 illustrates the scenario of comprehending doctors’ notes. A critical gap often exists between what patients understand about their health condition and what doctors document in their medical records. This discrepancy can have severe, even life-threatening consequences.
Consider the following scenario. Greg, a 30-year-old man, has a family history of a genetic condition that increases the risk of aortic dissection—a dangerous tearing of the large blood vessel branching off the heart. Greg begins seeing a cardiologist to monitor and manage this risk. However, a crucial miscommunication occurs:
- What Greg understands
-
He perceives his risk as moderate and think occasional check-ups are sufficient.
- What the cardiologist documents
-
The medical notes detail a high-risk condition requiring frequent monitoring and possibly preventive measures.
Tragically, Greg died from an aortic dissection just one year after his initial cardiology appointment. This outcome might have been prevented if the cardiologist had communicated the severity and urgency of Greg’s condition more clearly, and if Greg fully understood the serious nature of his risk and the importance of rigorous monitoring and treatment adherence.
This case illustrates how vital it is to have clear, thorough communication between healthcare providers and patients. When patients truly understand their health situations, they’re better equipped to participate actively in their care, potentially averting dire outcomes.
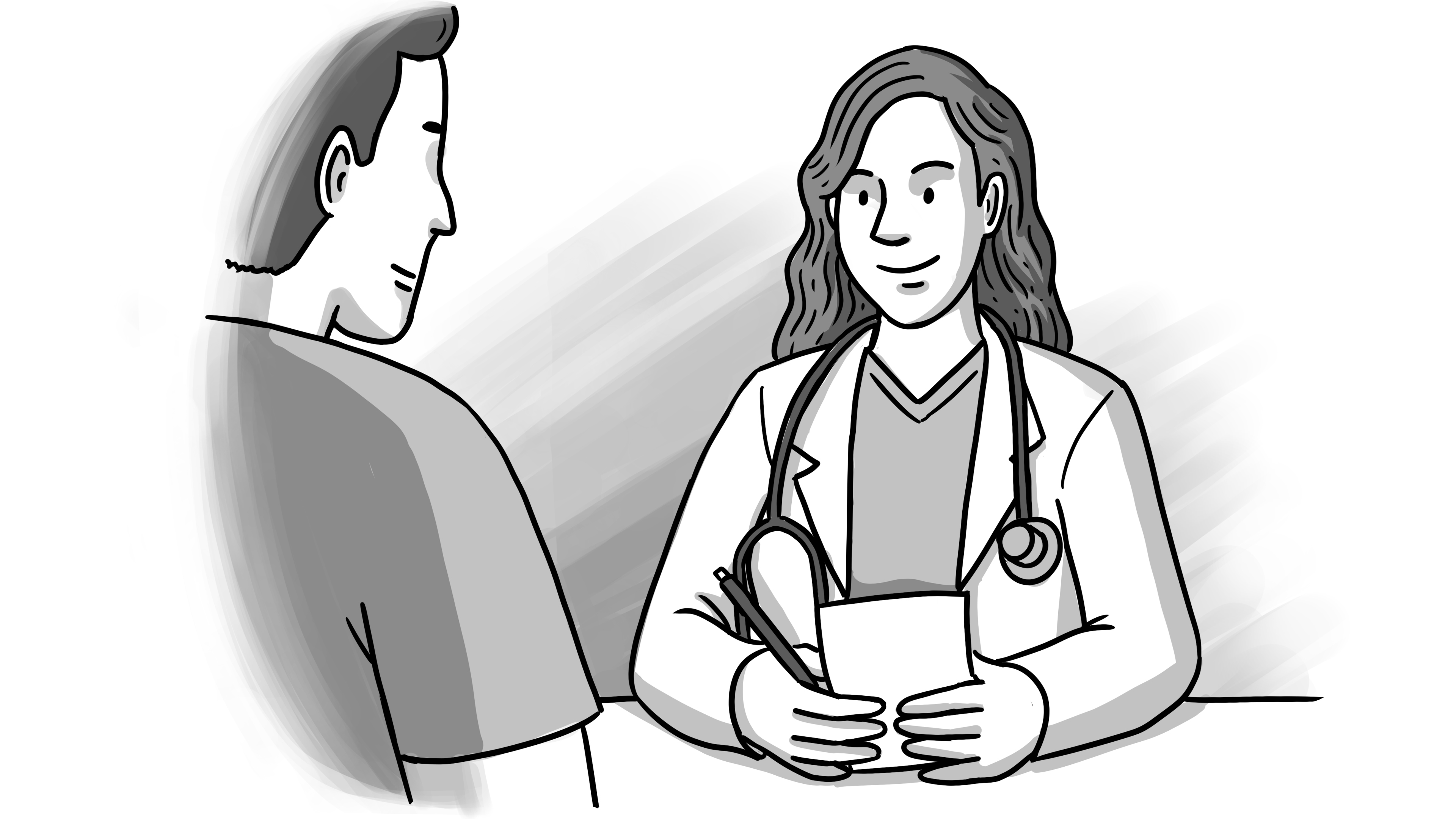
Figure 4-2. Comprehending doctors’ notes
Trying to remember a doctor’s communication and understanding a doctor’s notes often feels like deciphering ancient runes or recalling a specific meal from weeks ago: it’s a struggle filled with gaps and uncertainty. Patients are frequently left without a clear explanation of their condition or a concrete plan of action.
This disconnect between medical professionals and patients, caused by complex and often opaque medical documentation, creates a thick fog of misunderstanding. As a result, patients may experience:
-
Confusion about their health status and treatment plan
-
Increased anxiety due to lack of clear information
-
A diminished understanding of their overall health journey
This communication gap can affect a patient’s ability to manage their health effectively. Clear medical information is crucial for patients to actively participate in their care and make informed decisions about their health.
Notes sometimes poorly summarize patient information drawn from different specialties. A unified narrative needs to be formed to make sense of insights offered by multiple clinicians. The conclusion here is that many patients require assistance to understand the diagnoses, management plans, and directions of care recommended by doctors. As a result, patients can struggle to follow through on recommendations, and nonadherence to recommendations can lead to poor health outcomes. This problem is widespread and requires more convenient mechanisms to convert these technical summaries of the visit into more patient-friendly plain language.
A generated clinical summary could explain your test results and tell you not only what doctors want to do but why they want to do it. The summary would also give you a range of possible treatments and walk you through the reasoning that would make it appropriate to embark upon one of those versus another. Imagine how much time this might save for physicians and clinical staff in creating a visit summary and treatment plan. It would be indispensable to have a voice-to-text generated and shared summary from the visit, with or without your doctor’s input, explaining the questions you might have and also both their answers and generated-by-computer educated guesses of what might be useful to you to know, summarized and explained for you, annotated by your doctor if they have the time.– This would, for instance, allow you to query parts of your records in more natural language via chatbot interfaces powered by generative AI. The GenAI could explain everything, and then hopefully answer whatever part of the explanation you now want to be educated about.
Generative AI fosters explanatory media. Beyond text translation, AI might generate explanatory diagrams, videos, or other multimedia that make the content of a doctor’s note intelligible to patients in more interesting, interactive formats. LLMs can surface patient questions, and AI can scan notes and formulate questions about any subdots or portions of them that might warrant patient reconsideration and thinking through, tailoring bespoke questions for clarifying comprehension.
The gains go far beyond just operating a little more smoothly. Understanding your diagnosis without any confusion can empower you to take a more active role in your healthcare. When you have the ability to scan the diagnostic balloon and read your diagnosis, you have the capacity to ask more informed questions, you can make better choices for treatment, and you’ll tend to follow up on treatment plans, knowing they are working or that you’re well on your way. If you can help the doctor diagnose the problem, this creates a reciprocal communication loop that can create a more productive effective relationship between the patient and the doctor, improving outcomes in health and beyond.
Health Plan Wizard
Healthcare innovators are striving to make disparate systems dynamic—spanning insurance plans, provider networks, facilities, pharmacies, and social services—when managing and explaining policies and bureaucracies to patients seeking care access and/or complementary resources. Many healthcare organizations are looking to create seamless member experiences across fractured touchpoints.
At the ready stands the new generation of personalized health plans spearheaded by LLM wizards—virtual conversational agents encompassing behaviors of administrative as well as clinical functions to a central point of contact. Equipped with empathetic language models, health plan wizards engage in natural dialogue “checkups” with members—assessing a member’s needs—while the AI connects the relevance of doctor referrals, usage trends, associated care searches, and outcomes to identify appropriate and timely suggestions to members. Figure 4-3 shows a patient leveraging a health plan wizard to understand and navigate their health plans.
LLM health plan wizards can describe insurance vernacular about claims, referrals, formulary tiers, and eligibility to promote insurance utilization. Plain language can be their hub, and their spokes create confident consumers making healthcare decisions. Social determinants of health screening will tie patients experiencing hardship around food, housing, and finances with community organizations that can be beneficial. Culturally aware assistance can guide patients into equitable opportunities.
Armed with rich contextual insights regarding their patients, such personal health wizards enable improved experiences along the healthcare continuum, amid administrative and lifestyle barriers. Members receive personalized, targeted information rather than generic advice. All actions and communications are centered around the individual’s specific needs and circumstances, breaking down the traditional barriers of siloed, transaction-based interactions. Smoother interactions lead to more effective long-term health outcomes, fulfilling care promises.
Black Maternal Health
LLMs could support maternal health disparities more equally while promoting minority women’s journey toward a better pregnancy journey. With benefits such as omnichannel care linking clinical guidelines with wraparound care—which acknowledges all aspects of a vulnerable mother’s life, physical and mental—GenAI is a technology whose time has come to address the current crisis of increased morbidity and mortality among Black and Brown pregnant women (Figure 4-4).
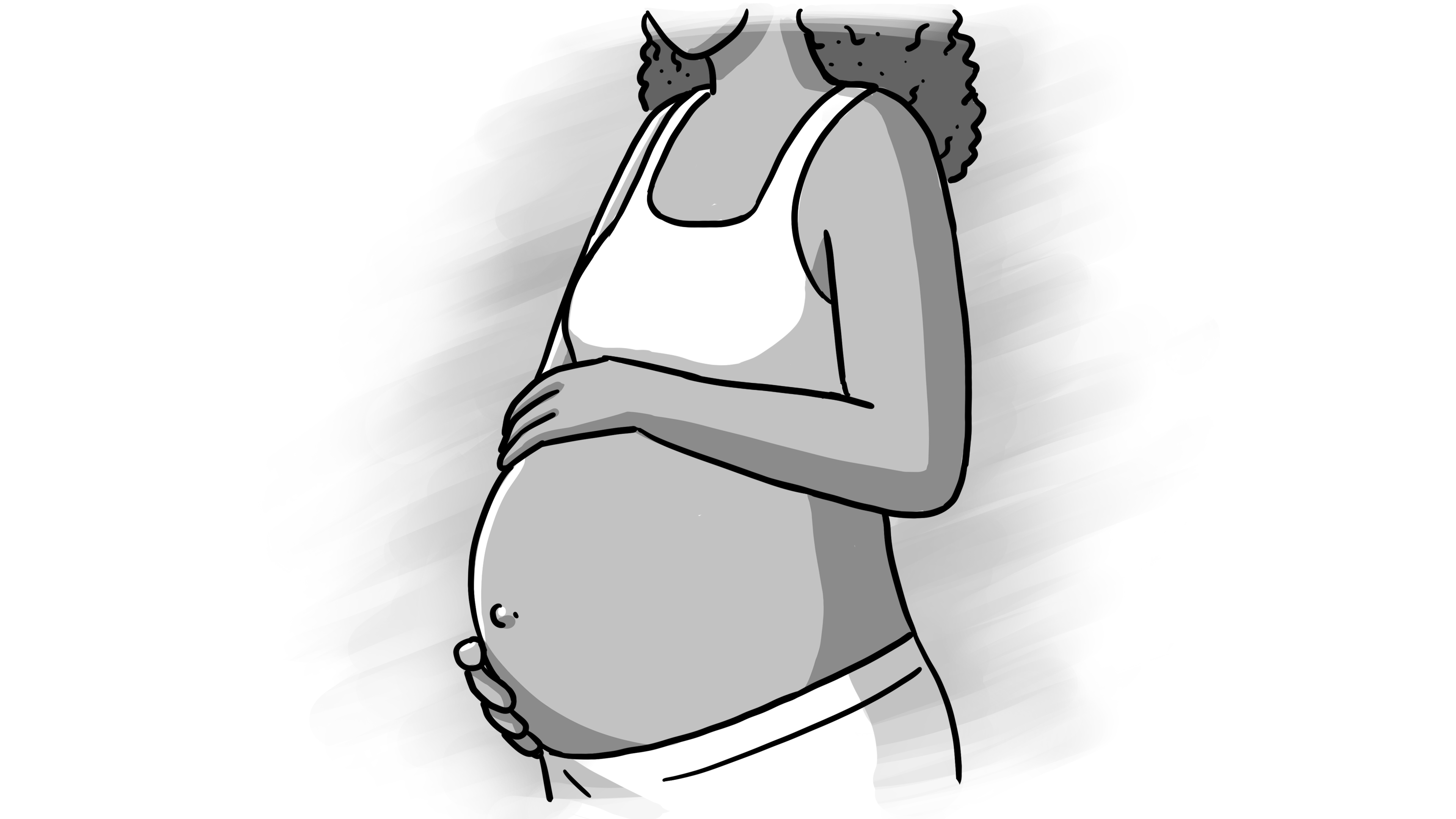
Figure 4-4. Expectant Brown mother
An equitable maternal health LLM app might invite more extensive discussions about social contexts, barriers to access, and specific concerns—beyond medical histories. It would tailor the support to the woman, directing her to a program for food support if required, counseling her on her rights in the workplace, birth preparation classes precisely chosen for her cultural needs, or even secure a ride service to enable her access to appointments.
Unconscious biases are insidious, with minority maternal health consequently affected. An LLM app could play a crucial role in combating these implicit physician assumptions in the following ways:
-
By retaining a relevant LLM—through powerful medical profiles that incorporate cues about the social/cultural context in which a birthing goal is envisioned—physicians who might exploit an unfettered decision-making process are blocked. The LLM slows down rushed recommendations.
-
It could watch patient-physician interactions to guard against the discounting of minority women’s symptoms and concerns, insert prompts for validating the patient’s concerns, and recommend additional testing as required.
-
It could help patients review the proceedings following appointments and flag areas where unconscious stereotyping might have resulted in the dismissal of their concerns or caused alterations in their treatment plan. For example, with access to the full timelines of patients, the LLM can perform differential analytics against baseline standards and champion patients receiving fewer proactive interventions than peers with similar risk presentation profiles.
At a minimum, patterns of differential treatment might become detectable over time, and drive policy directives and standards for evidence-based care regimens that aim to compensate for differential quality, maybe even unintentionally inspired by implicit bias in prenatal care settings.
The prompts and milestone-markers would trigger personalized reminders and questions for the patient to discuss with her doctors, from testing schedules to birthing plans, while also providing interactive education regarding the maternal health hazards that disproportionately threaten minorities. The on-call advocate could step in over troubling symptoms and could equip women to get the best possible care.
After birth, the LLM app would continue to protect a woman throughout recovery and her child’s development through targeted advice and connections to community resources. It puts women at the center of their own health. The app creates opportunities that bypass inherently racist legal systems and bolster minority women’s agency in accessing the healthy, empowered pregnancies they rightfully deserve.
In conclusion, an AI-powered chatbot using LLMs can be a vigilant partner in maternal healthcare. This technology can:
-
Actively identify unconscious biases that negatively impact minority maternal health
-
Provide consistent advocacy for patients
-
Offer real-time support during medical encounters
-
Monitor long-term care to ensure continuity and equity
The ultimate goal is to ensure that every mother, regardless of background, receives high-quality, advanced care free from prejudice. This AI partner works continuously to promote equitable treatment in immediate medical situations and throughout the maternal health journey.
Equity-focused LLMs offer promising innovations to support those most affected by healthcare disparities. These AI systems can:
-
Transform historical data and experiences into meaningful insights
-
Improve access to quality healthcare for underserved populations
Looking to the future, this technology could contribute to a world where:
-
All viable embryos have the opportunity to develop and be born, regardless of socioeconomic factors
-
Women of all racial and ethnic backgrounds have equal access to advanced reproductive technologies, high-quality prenatal and maternal care, and safe childbirth experiences
The goal is to create a healthcare system where race, ethnicity, and socioeconomic status no longer determine maternal and infant health outcomes.
Medication Reminder
Following medication regimens can be astonishingly challenging. Treatment plans often involve intricate instructions around timing, diet, conflicts with other remedies, and more. Even motivated patients need help with forgetfulness, confusing details, or just finding the regimen unpleasant. Hence, barriers to prescription adherence remain prevalent across diverse groups.
Healthcare providers and research shows that patients who stop taking their medicine risk the progression of disease, higher healthcare costs, and even death—particularly for those managing chronic illness. Physicians do make attempts to simplify treatment, for example, by striving for a single-dose, once-a-day regimens. But unavoidable complexity remains an ingredient in certain treatment formulations.
A 2017 medication adherence study1 compared a variety of low-priced memory aids against a control group who received no intervention. The tools were simple: a pill bottle strip with slide toggles to indicate which day’s dosage had been taken; a cap tracking open timestamps; and an eight-compartment standard daily pill organizer.
Here are the key findings in the study’s results:
-
The low-priced memory aids (pill bottle strip with toggles, cap tracking open-time timestamps, and standard daily pill organizer) did not improve medication adherence compared to the control group that received no intervention.
-
This conclusion was not immediately apparent from the study’s primary results but was revealed through analysis of pharmacy refill data.
-
The ineffectiveness of these common adherence tools was an unexpected or potentially unwelcome finding.
-
The pharmacy refill data provided a more accurate or comprehensive picture of adherence than the study’s primary measures.
These buried findings are significant because they challenge the assumed effectiveness of commonly used, low-cost methods to improve medication adherence. More innovative or comprehensive approaches may be needed to impact patient behavior when taking prescribed medications.
The authors of the study speculated that this emphasis on reminders ignores why and when doses are missed. What’s needed for medication adherence is better insights into patient contexts, interests, and barriers. Yet, the failure of efficacy in even basic medication memory aids speaks to the stubbornness of the adherence problem. Figure 4-5 depicts a patient using technology to help them remember when to take their medications.

Figure 4-5. Medication reminders
A 2022 NIH report2 examining technologies for medication adherence monitoring reached some interesting conclusions:
-
Current adherence-monitoring technologies have varying features and approaches to data capture. They can be further enhanced through technological innovation.
-
New research paradigms should be deeply integrated and interoperable with clinical settings and health information systems; in other words, the utility of a research approach needs to maximize the potential of the broader technological ecosystem.
-
While promising, individually, none of these technologies constitutes a magic-bullet “gold standard.” More likely, they will work best in conjunction with one another as part of a multimodal solution tapping the strengths of emerging tech and traditional methods.
-
There’s no doubt that the evidence base for adherence technologies is growing, and they could be a real driver of better adherence behaviors and health outcomes. But the evidence base for the functionality of technologies and their impact on adherence outcomes needs to expand still more.
Once we’re aware of and debiased against such artifacts, we shouldn’t expect LLMs to be a magic bullet—they will only be a promising complement to current modalities. A collaborative, evidence-based approach to creating LLMs that can be iteratively used alongside current, context-aware, patient-centered adherence-support tools may be the realistic and only realistic route.
Oral Health
LLMs and generative AI can help teledentistry in several ways:
- Routine tasks
-
LLMs automate those routine tasks such as sending reminders and processing payments, freeing up dentists and dental hygienists to serve patients.
- Data analyses
-
LLMs analyze data from teledentistry sessions, in the form of video chats and dental images, to improve quality of care by identifying trends and patterns.
- Report generation
-
LLMs create reports detailing the teledentistry session results to communicate with patients and other healthcare providers.
- Patient education
-
An LLM provides dental health education by talking with you over video chat, text messaging, or using a chatbot.
- Translation
-
LLMs are proficient in translating languages. This gives rise to teledentistry patients speaking in any language.
LLMs can significantly transform the field of teledentistry in several ways, including enhanced patient interactions and personalized communication.
LLMs can power virtual assistants and chatbots that answer patients’ initial queries about teledentistry services, insurance coverage, or basic oral health information. This can free up dentists’ time for more complex consultations.
LLMs can analyze a patient’s medical history and dental concerns to tailor communication and educational materials. Imagine an LLM that generates customized preappointment emails or post-treatment instructions based on the patient’s needs.
LLMs could educate patients on oral health. Based on analyzing millions of studies and millions of patients’ electronic medical records, an LLM could develop personalized education about brushing, flossing, diet, and other dental hygiene topics that match a patient’s risk factors and preferences. LLMs could interpret patient-reported symptoms, and could often provide answers on why patients might be having problems and when to see their dentist. LLMs could explain procedures and medications in plain language, and they could describe the expected benefits and burdens of a treatment plan. LLMs could discuss and review options with patients, which might improve how well patients follow expert diagnoses and recommendations. LLMs could provide interactive chatbots that might differ from dental assistants in that they would be programmed to provide compassionate support and anxiety-arresting techniques to reduce the fear of visiting the dentist in patients with dental anxiety.
For dentists, they could examine patient records and medical histories, providing suggested diagnosis and treatment recommendations and even flagging potential side effects and contraindications. LLMs could rapidly crawl through the dental literature to help them stay up to speed on the latest research and best practice. LLMs could input accurate and comprehensive notes for the dentist without wasting time on formatting and spelling, and they could improve precision. They could be programmed to tailor communication to patients’ individual needs and preferences, improving engagement and satisfaction. Figure 4-6 illustrates a dentist using AI technology for oral health while an LLM provides summarization on a patient’s oral health history.
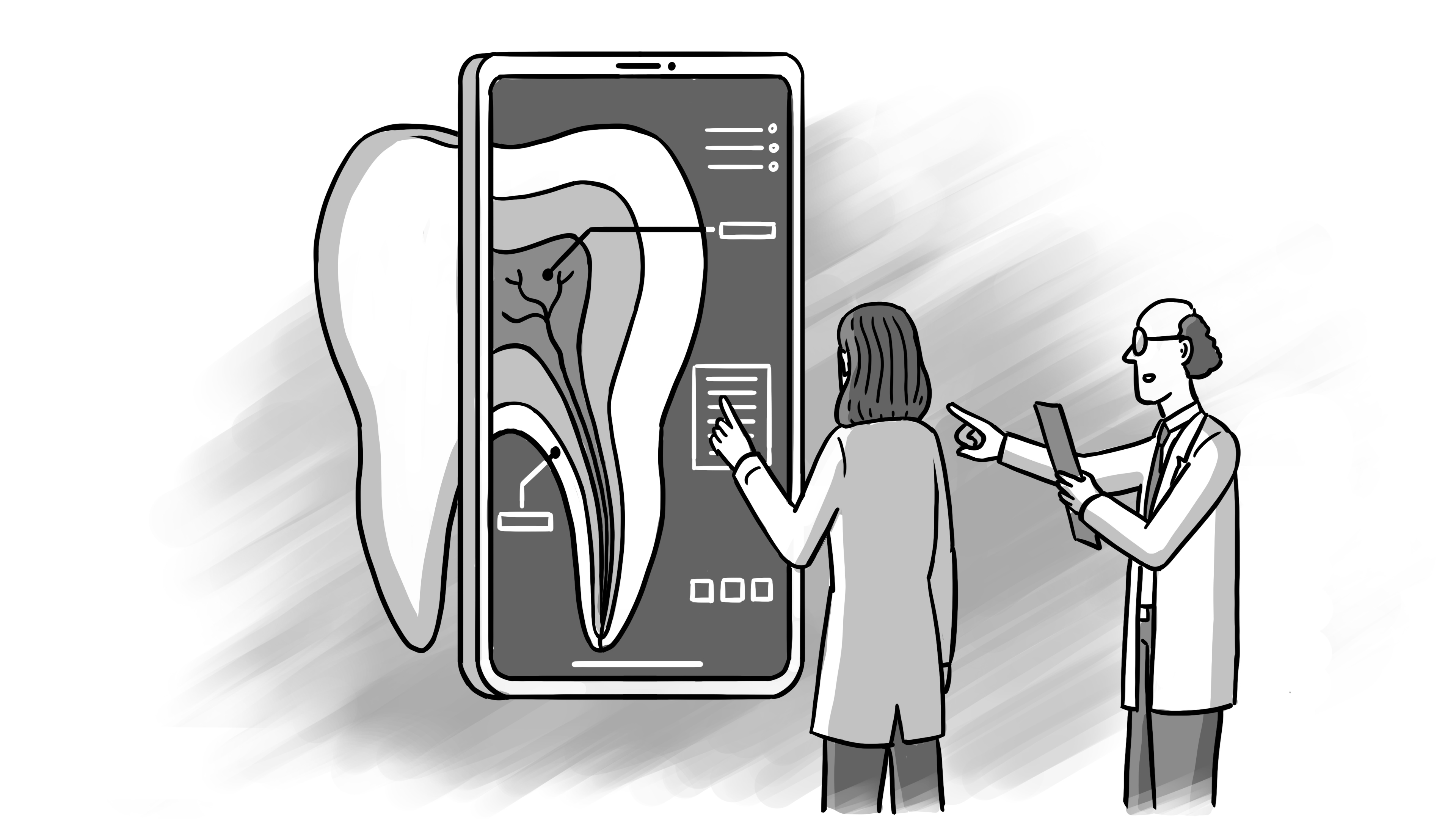
Figure 4-6. Oral health
LLMs are there 24/7, helping to overcome barriers associated with time and geographical distance. They are never too busy to answer clinical questions, and are not distracted by phones ringing or frustrated patients waiting in the queue to be seen. But they are not the end of the road—dentists still need to exercise their clinical judgment. LLM answers are there to support dentists, not supplant them. Overall, therefore, LLMs hold immense promise for moving beyond teledentistry. LLMs could place power back into patients’ hands; they could support dentists; and they, too, would have a role to play in improving the oral health of our populations.
Symptom Checker
Symptom checkers are computerized tools or apps that allow patients to enter their chief complaint and possible concurrent symptoms, and provide the patient with an assessment or potential diagnosis. Well-known examples include WebMD, the symptom checker by the Mayo Clinic, as well as Ada Health. Most symptom checkers today use a rule-based approach, where patients enter symptoms, and then the tool uses this information to draw upon a compiled database of disease descriptions by medical experts and to provide a potential diagnosis. Though symptom checkers can indeed provide helpful contributions to patient self-diagnosis, they are limited in a number of ways:
-
They fail to capture the nuances and subtleties of patients’ actual symptoms.
-
They provide a laundry list of potential conditions without clear probabilistic rankings.
-
They account poorly for comorbidities, and they do not iterate initial questions intelligently.
-
They possess a limited ability to explain their reasoning or advise optimal next steps.
-
They often present a limited set of possible diagnoses, potentially overlooking other possibilities.
-
Their diagnoses are based on user-reported data and can be prone to inaccuracy or partiality. Algorithmic systems must be more nuanced to handle edge cases or rare conditions.
LLMs have the potential to enhance symptom checkers (Figure 4-7) dramatically in several ways:
-
Symptom checkers identify salient figures of speech and patient specifics in the text in natural language, and refine them using language qualified by more accurate terms thanks to suggestions made by LLMs trained on a large medical data set (including diagnoses of patients, their cases, and relevant research articles).
-
Symptom checkers attach predicted levels (probabilities) of benefit to the various possible diagnoses using statistical calculations and clinician-guided heuristics. They engage in a series of follow-up questions based on the results of prior questions in order to discard improbable conditions.
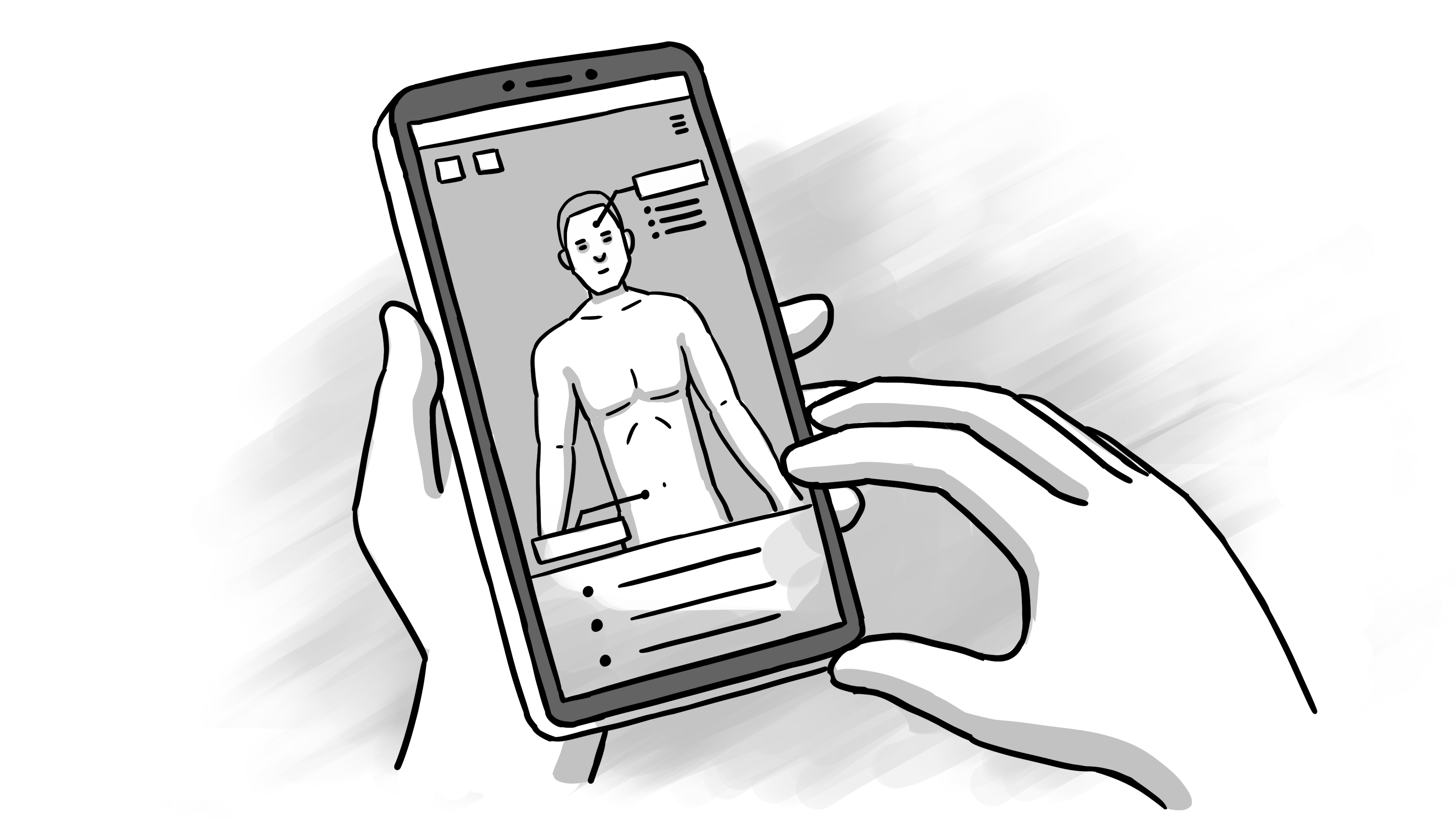
Figure 4-7. Symptom checker
LLMs offer the opportunity for a better understanding of context. LLMs can analyze complex narratives and consider individual contexts like medical history, age, and lifestyle factors, leading to more personalized recommendations. LLMs can provide more nuanced information, highlighting uncertainties and guiding users toward reliable sources such as healthcare professionals. LLMs can identify high-risk cases based on specific symptoms and direct users to seek immediate medical attention. They can continuously learn from new data and feedback, improving accuracy and adapting to evolving medical knowledge.
Combining large-scale pretraining, expert medical knowledge, and rigorous validation testing, LLM-powered symptom checkers could greatly improve patient self-service capabilities, clinical efficiency, and health outcomes.
Clinical Decision Support
The potential of LLMs and generative AI in the field of clinical decision support is vast. Clinical care routinely involves planning patient treatment, which includes carefully considering potential risks and benefits of the treatment options. Clinical practice guidelines (CPGs) published by medical associations are based on the best available population-level evidence and are intended to assist healthcare professionals in making clinical decisions.
However, these practice guidelines may be ambiguous or suboptimal when considering polychronic patients that suffer from multiple intersecting chronic conditions. These complexities pose challenges because CPGs are oriented to single conditions, and it is left to clinician judgment to adjudicate between conflicting recommendations from multiple guidelines. For example, consider an aging population that exhibits increasing clinical complexities and care demands, resulting in patterns of super-additive costs when diseases interact.
Application of disease-specific CPGs to patients with multiple diseases can lead to competing recommendations and the potential for adverse drug-drug or drug-disease interactions. For example, medications indicated for heart failure could compromise kidney function in those with kidney disease, or nonsteroidal anti-inflammatory drugs (NSAIDs) may be suggested to treat osteoarthritis pain but turn out to have relative contraindication in patients with a history of peptic ulcer disease.
To account for the patient’s unique circumstances, such as demographics, family and disease history, or individual physician practice patterns, doctors may deviate from applicable guidelines partially or fully. While these deviations may be appropriate in certain cases, they can also lead to unwarranted variation and poorer health outcomes. In contrast to deviations that manually personalize clinical care, deviations may also result from professional uncertainty, such as lack of specialized domain expertise or uncertainty about treatment options. The clinical insight bot or curbside physician use cases profiled in the next section could alleviate some of the risks just outlined for polychronic patients.
Clinical Insight Bot
The doctor does what doctors do. The patient’s story, history, labs and diagnostic tests, and tentative treatment plan are laid out before the clinical insight bot. The LLM digs deeper. It asks questions to learn more but not to judge or to conclude. Could it really be that unusual disease? Do the two medications interact? Are there some clinical trials studying this illness or multiple diseases afflicting the patient? Interacting with a virtual assistant, as depicted in Figure 4-8, provides enormous benefits to a physician.

Figure 4-8. Physician in conversation with an AI-powered clinical insights chatbot
The LLM reaches deep into its databases of medical literature, research papers, case studies, and even clinical guidelines, then picks out what it thinks you need to know. It looks for patterns, associations, and mistakes that the doctor might have missed. It scrutinizes the treatment plan, checks how well it’s worked so far, and nudges you in the direction of some more effective options that now have strong backing in the evidence.
But the LLM isn’t just a data processor: it is an analytical machine that puts forth assumptions and highlights potential biases. It proposes alternative diagnoses that might explain the patient’s symptoms or points to areas of uncertainty, prompting the doctor to order more testing or to consult with another physician. In short, it doesn’t replace human judgment with “computerized medicine.” Rather, it augments it, offers a broader perspective, and makes sure that every possible angle has been considered.
Now, a newly emboldened doctor examines the LLM’s output and leaves the room with a revised plan in hand. The consultation process has been nuanced yet natural as clinician and AI work together, with previous expertise augmented and guided by the LLM’s analysis. The risks and benefits of the new plan can be weighed, and the physician’s confidence in the conclusion is buoyed, if not fully won. She has an ally in her efforts to improve outcomes—a silent partner.
And that is only the beginning of what the future medical consultation might look like, with LLMs aiding but not replacing human doctors. They won’t be the end of the personal touch, the empathy, and the reasoning that define what it means to be a good physician. LLMs will be the most powerful tool that the doctor has available to sift through the plethora of information to provide informed, personalized, and humanistic care for all of us.
A clinical insight bot differs from the AI curbside physician as a core task for a clinical insight bot would be to surface insights, patterns, and trends from a set of clinical data—e.g., electronic health records, medical literature, clinical studies, and the like—in ways that help clinicians make better decisions.
A clinical insight bot would largely be fed by structured and unstructured clinical data sources, namely electronic health records, claims data, medical literature, results of clinical trials, and patient-generated health data, and then would utilize advanced analytics, NLP, and machine learning to extract clinically meaningful insights and patterns from these.
Unlike real-time individual analysis, clinical insight bots take a data-driven approach to generate insights for clinicians and healthcare professionals. These insights are delivered periodically, at predetermined intervals, or triggered by specific events. The bots analyze vast amounts of anonymized data to identify trends and patterns at the population level. This anonymized data protects patient privacy while allowing the bot to uncover broader healthcare trends. The insights are then presented in clear formats like reports, dashboards, or alerts. Ultimately, these data-driven insights are designed to empower clinicians and healthcare professionals to make informed judgments and decisions that benefit entire populations or healthcare systems.
AI Curbside Physician
A curbside consultation is an informal advice exchange between medical providers about real patient cases. An informal exchange of advice between medical providers, a curbside consultation considers cases that are still pending resolution in real patients, as metaphorically illustrated in Figure 4-9.
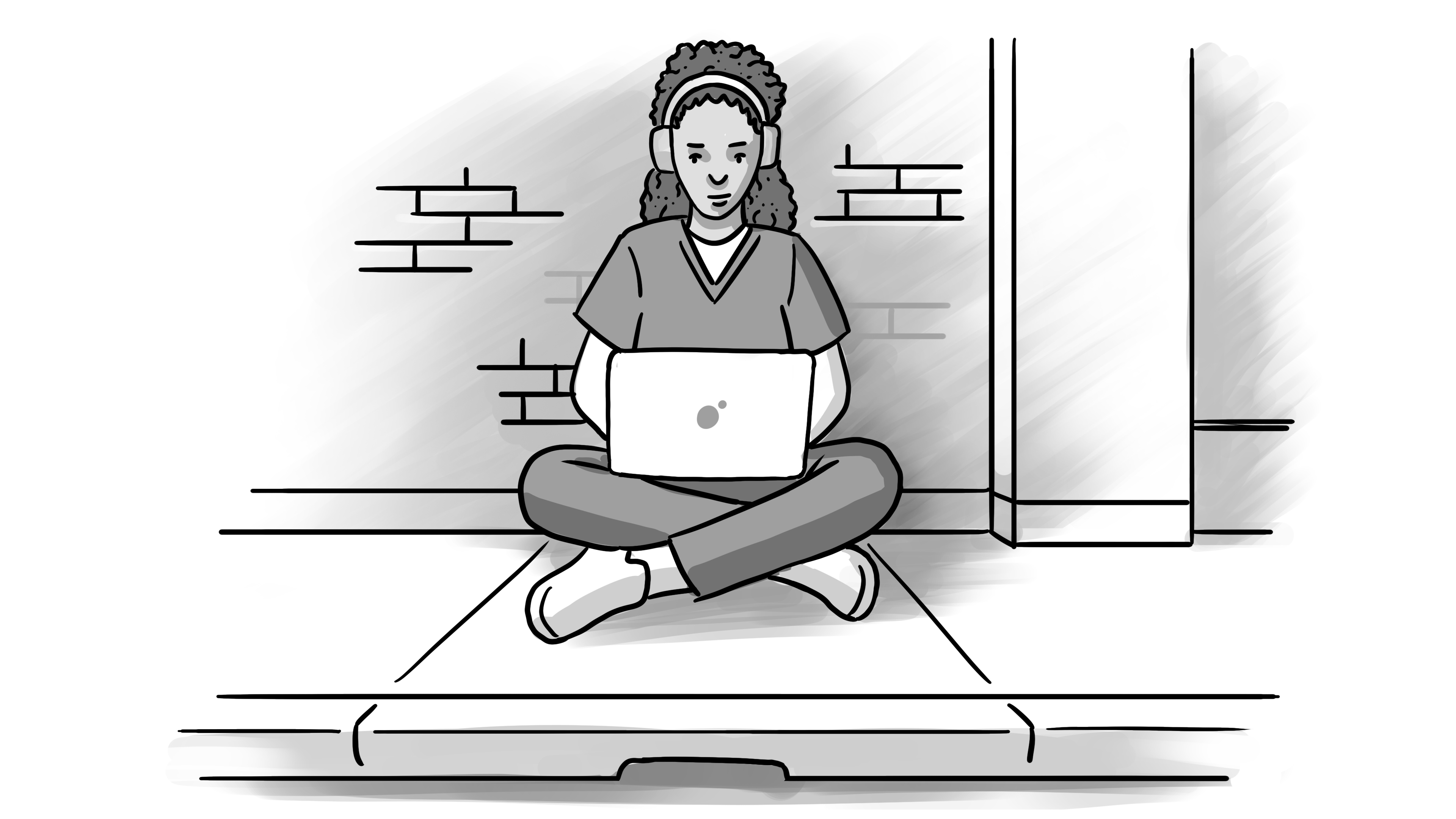
Figure 4-9. Curbside physician
Say, on a shift in the intensive care unit (ICU), you are concerned about the lack of fever in patient Jean. You have just spoken to a colleague in ICU, explaining that she has grade 4 lactic acidosis, an ECG with low voltages, a GI bleed in the past 12 hours, and newly onset platelet count reaching 70,000. However, the patient admitted at 7 p.m. remains afebrile. You are stumped. You know your colleague from ED has recently used an app that acts as a virtual curbside doc. You took her advice twice last week with excellent outcomes. You decide to give it a try.
Your patient got burned when her robe caught fire during a weed-vaping session. The burn was superficial, although it covered a large surface area (approximately 20% of total body surface area). Today, she is back in the burn clinic, and you are conducting a daily chemotherapy dose. A week earlier, the wound was filled with fluid and the pinch test was positive. Although the pinch test should not have been needed prior to today, you decided to go ahead with second-degree burn care, including bandaging and debridement. Now, the pinch test is negative, the dressings are smelly and discolored, and there is a white layer. You are surprised and concerned because the signs and symptoms are concerning. You want to consult the wound care expert, but there are no good ways to contact her. You remember your colleague telling you about an app that functions like a curbside doc.
An AI curbside doctor provides instant access to continuously evolving medical information, mimicking the valuable insights you might otherwise only get from impromptu consultations with colleagues. This digital tool replicates the knowledge-sharing that occurs during “house rounds” with master clinicians in the US, or the morning, midday, and afternoon “table-side” chats with peers in UK hospitals. By doing so, it brings the benefits of collaborative medical expertise to clinicians’ fingertips at any time, without the need for physical presence or scheduling. You can use an LLM to generate a set of hypotheses about 1) what is causing a patient’s symptoms, 2) the strategy for alleviating those symptoms, 3) what factors might be relevant, and 4) what steps should be taken next.
The AI curbside physician is ready for informal case discussions any time of day, any day of the week. You talk about your patient, the issue at hand (test results, physical exam findings, previous history), and get responses in return, often of a high diagnostic quality. The AI might also produce lists of likely diagnoses with associated probabilities. These probabilities would be based on specific symptoms present, the timeline or progression of these symptoms, and relevant risk factors.
The AI curbside physician would consider how these elements correspond to established diagnostic criteria or standards found in the medical literature.
It can even make appropriate probing questions to clarify or provide more details regarding missing case features that could distinguish between different possible diagnoses, or in certain cases recommend further testing or testing strategies. In a nutshell, LLMs will change curbside consultation for the better. They will help doctors provide more personalized and efficient care to patients.
The key function of an AI curbside physician would be to deliver rapid and casual advice and second opinions to healthcare professionals. To put it differently, it would focus on facilitating “curbside consultations.” Basically, it would attempt to help physicians make clinical decisions when they encounter challenges. It would attempt to deliver evidence-backed advice and expert opinions regarding specific pediatric patient cases or general inquiries about medicine.
A curbside physician bot would draw on the wisdom and knowledge of experienced physicians written down in the medical literature, clinical guidelines, and opinion pieces, and it would use soft logic and NLP and information retrieval techniques to retrieve and contextualize the relevant information for the given clinical question.
The curbside physician bot would carry on a conversation with you as if it were an expert at your side, addressing issues in a case-specific, personalized way that depended on the characteristics of the particular patient you saw and your specific questions or concerns.
A curbside physician bot would have a narrower domain of relevance, providing input and recommendations to very specific clinical scenarios, but drawing from a very generalized medical knowledge base and be able to offer general guidance for what the human physician might recommend. Such a system might offer rapid and convenient access to medical knowledge that comes with some potential for criticism if the recommendations weren’t tailored for that individual patient.
Remote Patient Monitoring
Remote patient monitoring is an increasingly viable approach to improving patient outcomes. Generative AI and LLM apps will be able to help remote patient monitoring in several ways. An LLM would be able to personalize each patient’s remote patient monitoring experience to ensure that the experience is as efficient and optimal as possible. By considering the patient and their preferences, an LLM might generate a schedule that best meets the patient’s needs each day. It also might be capable of providing personalized feedback regarding the patient’s progress.
LLMs can be used to give patients feedback on their health data in real-time based on their data readings. For instance, using remote patient monitoring, an LLM can alert patients if their blood pressure or heart rate is too low. Figure 4-10 illustrates a remote patient monitoring session.

Figure 4-10. Remote patient monitoring powered by LLM app
In the world of medicine, LLMs can search a patient’s health data and scan for problems. They can do so by spotting irregularities and patterns in the data that could indicate a problem, for instance, it could assist in identifying a patient who is at risk of developing diabetes or one who is likely to suffer a heart attack. LLMs can also remind the patient to take their medication or book an appointment with a doctor, which can assist the patient in taking care of their health and not missing a doctor’s appointment.
Furthermore, LLMs can be used for informative and engaging modes of communication with patients by using NLP to analyze their questions and concerns, and then replying to them again in a human-sounding manner and addressing individual aspects of their needs.
Digital Twin
A digital twin of an individual, an organ, or even a medical device could mimic its behavior and characteristics based on the real-world data it was created from. Through the use of digital twins, healthcare practitioners could make sense of the data, predict outcomes, and optimize treatment.
Some key aspects of digital twins in healthcare:
- Patient-specific modeling
-
One aim of developing digital twins for healthcare could be that each patient has their own digital twin model. This model would combine the patient’s medical history, their specific genetic information, lifestyle factors, and other relevant data. This digital twin would be continuously updated with real-time health data from personal devices (such as smartwatches or glucose monitors), medical test results, and changes in lifestyle or medication. The digital twin would be constantly receiving and integrating new data. Then the data would be analyzed frequently by AI systems to detect changes or trends and used to provide up-to-date insights for healthcare providers and the patient. This ongoing monitoring ensures that the digital twin remains an accurate, current representation of the patient’s health status, enabling more timely and personalized medical interventions as necessary.
- Organ/disease simulation
-
Digital twins can be developed for specific organs or diseases, e.g. a virtual heart, or even a digital version of a tumor, with the latter being able to simulate the way a disease progresses in the body or predict the outcome of a proposed course of treatment.
- Medical device optimization
-
Digital twins could simulate virtual sensor-rich versions of implantable devices such as pacemakers or insulin pumps whose performance could be explored under different circumstances. For example, manufacturers may be able to run the device virtually to optimize its output.
- Predictive analytics
-
Use of machine learning algorithms on digital twin data in real time enables modeling of likely health risks, adverse events, or treatment responses to initiate preemptive interventions.
- Virtual clinical trials
-
Digital twins could be put through virtual clinical trials to test new drugs or other therapies, reducing patients’ exposure to new pharmaceutical compounds and tests. Virtual clinical trials involving large populations of digital patient models can provide far more information about the effects of a treatment or simulate typical variability in a population.
- Remote monitoring and telemedicine
-
Digital twins enhance remote patient monitoring by continuously tracking patient status. The digital model is updated in real time with data from wearable devices and other health sensors. The digital twin can quickly identify unusual changes by comparing current data to the patient’s baseline and expected patterns. Healthcare providers can be alerted immediately when significant deviations are detected, allowing prompt action. The digital twin’s comprehensive model of the patient allows for more tailored monitoring and treatment plans.
Digital twins can improve patient outcomes, pharmaceutical development, and medical device design in and out of hospitals. There’s little downside to having a high-definition, prediction-powered, streamlined, and individualized version of you.
But it brings up new questions about data privacy, security, and ethics concerns related to the extension of digital twins into health. How will patient information be protected? What regulations will govern the use of digital twins? Figure 4-11 illustrates a digital twin.
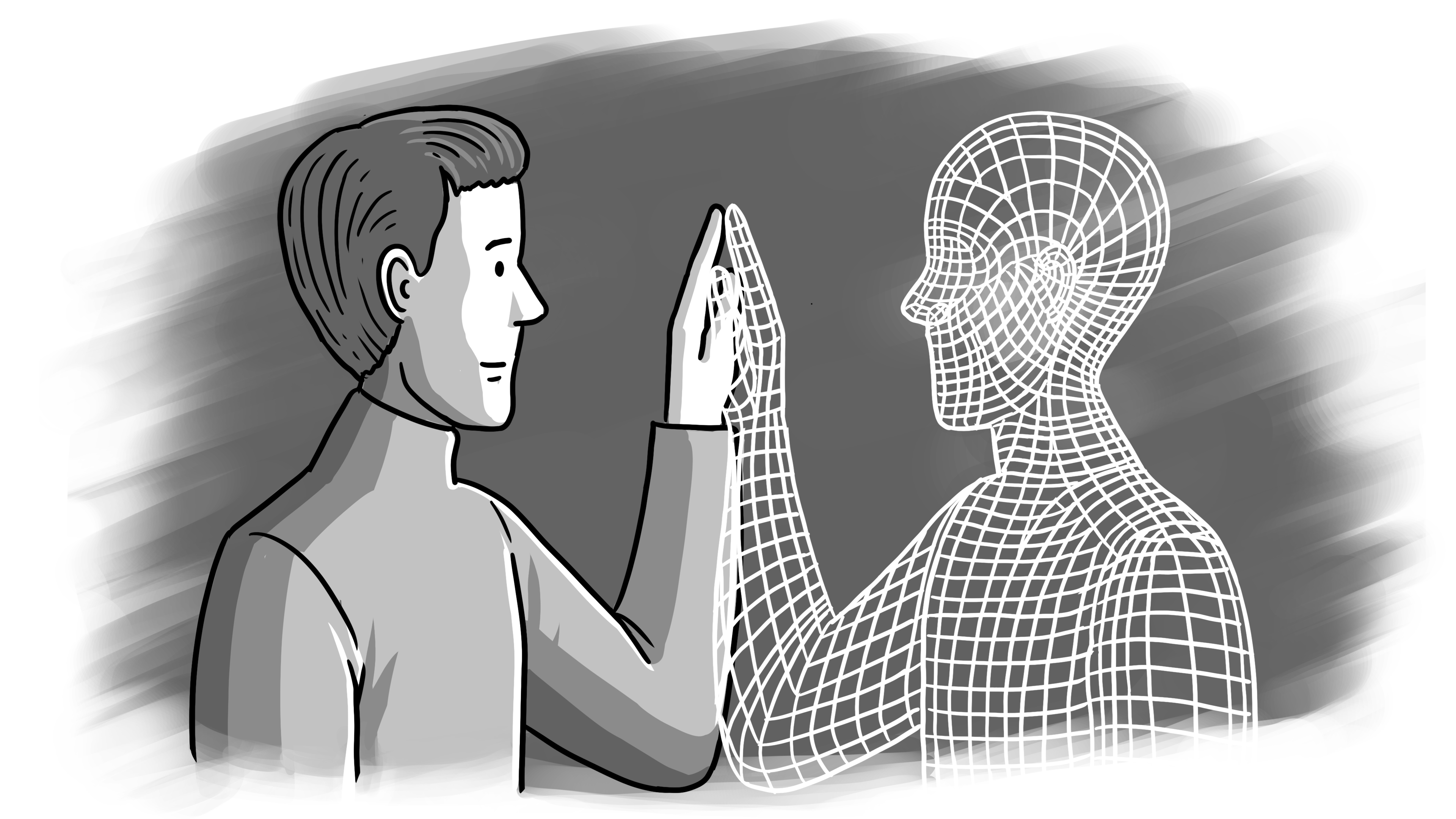
Figure 4-11. Digital twin
Routine analysis of integrated care records might reveal all the possible medication regimens to which the patient could be treated, and might reveal cautions, contraindications, precautions, interactions, or efficacy considerations. Side effects and outcomes might be projected at the personalized level under different treatment interventions for risk/benefit assessment based on that individual’s genome, biomarkers, and other prior response data. The bigger imperative is in clinicians simulating the digital twin, basically, to choose what course of intervention will be most effective and which medication or therapy will be most effective.
Digital streams from remote patient monitoring devices using wearable biosensors can be automatically monitored and contextualized with actionable clinical information (e.g., early infection warning signs). If anomalies in trends are detected, the digital twin will generate alerts and warnings to clinicians to allow for timely interventions and treatment regimen adjustments.
In addition to its ability to integrate data from various sources, conduct generative analysis, and provide high-precision recommendations, an LLM-powered digital twin of a patient would be a reliable knowledge-driven assistant that clinicians can query at the point of care for otherwise unattainable, data-driven insights. This would help improve the quality of clinical decisions and patient outcomes. An LLM-driven twin is a decision-support tool for clinicians guiding them toward insights, personalized recommendations, and continuous monitoring of patients, leading to enhanced clinical decision making and patient outcomes.
Doctor Letter Generation
Physicians face escalating paperwork demands that divert precious time from direct patient care. Letters addressing prior authorizations, disability claims, return-to-work needs, and other centralized requests prove administratively burdensome, given their unstructured formats. High volumes and inflexible templates multiply inefficiencies across practices. Figure 4-12 depicts a doctor using generative AI to do their paperwork.
One of the first adaptive doctor letter generation LLM solutions, known as ScribeMD,3 works by allowing doctors to input the letter verbally and then augment it in NLP until it reaches a complete professional missive. Physicians must dictate those points in a voice prompt, like so: “This former patient of ours at St. Francis Memorial Hospital named Sam Jones…” And they go on to list their history, to whom the note is intended, primary care details, hospital policy requirements, and recommended action for caregivers, including documentation, intubation, or antibiotic details.
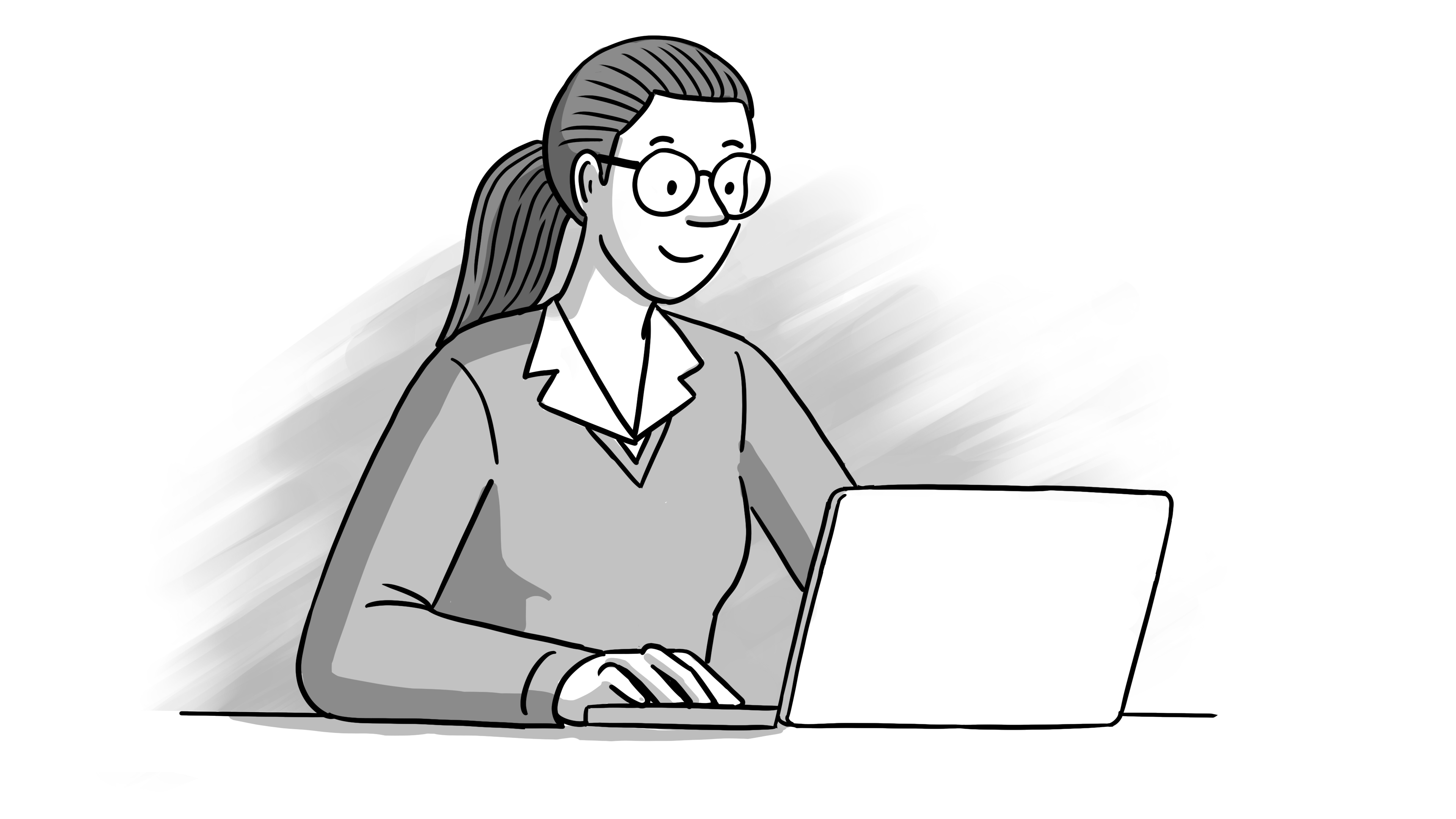
Figure 4-12. Doctor using generative AI to create letters, forms, and emails
ScribeMD, the tool running on this particular prompt, is conversant in HIPAA-compliant LLMs and dissects these prompt components before continuing the drafting of a letter. Physicians edit autogenerated letters when necessary before signing correspondence. Frequently, automation is doing the raw document creation, citation sourcing, and standardized formatting—from deeply detailed “History of Present Illness” to producing return-to-work release notes.
By iterating through multiple cases, ScribeMD’s predictive outputs gradually improve over time as the model learns various stylistic quirks, clues about where to cite evidence, and the structural profile “fixed expressions” specific to that particular provider, subspecialty, and area of practice. With broad uptake, over time both the training corpus used to develop this system and its continual feedback calibrations will grow exponentially, cutting drafting time to as little as 30 seconds.
Leaping from templates to adaptable narratives lowers the labor of clinical documentation while conveying nuance. Patients also benefit from personalization. ScribeMD thus helps physicians share care knowledge conversationally instead of battling form gaps in the production of health services, thereby avoiding the pain of perpetual paperwork.
Health Equity
Health equity means that all people (Figure 4-13) have a fair and just opportunity to achieve their highest level of health, unhindered by their social, economic, or demographic status. It means having no avoidable, unfair, or remediable differences among those populations in their overall health status and access to healthcare services.
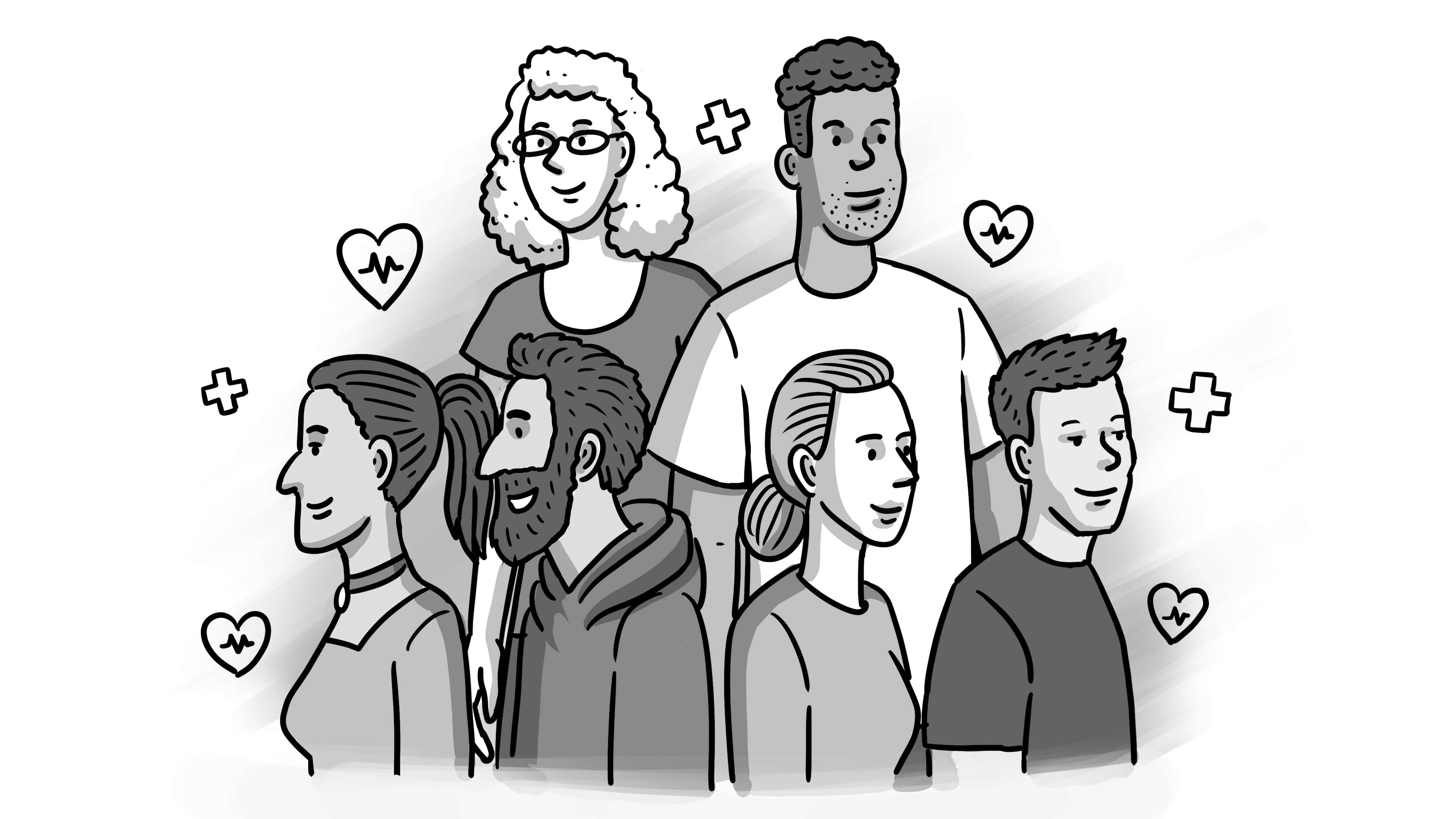
Figure 4-13. Health equity for all
Key aspects of health equity include:
- Equal access
-
Equal access to healthcare means that everyone—no matter if they are rich, poor, black, white, etc., and no matter their age, sex, gender, or physical capabilities—should have access to medications or any type of care that they need, whenever they need it.
- Social determinants of health
-
Achieving health equity will require attending to the social, economic, and environmental factors that play a direct and indirect role in shaping health outcomes, at the individual and community levels, including education, employment, housing, and transportation.
- Leveling the playing field
-
Health equity is pursued to eliminate disparities in health outcomes that are systemic, avoidable, and unjust as a consequence of specific social and economic policies and practices.
- Policies and programs
-
Health equity entails developing and implementing policies and programs that are designed to meet the needs of marginalized and vulnerable communities, taking into account any resource inequities.
- Empowerment and participation
-
Health equity entails strengthening the voice of individuals and communities to participate in decisions that affect their health and well-being.
- Cultural competence
-
Health equity demands that healthcare providers and healthcare systems, institutional or organizational, are competent in understanding and respecting the values, beliefs, behaviors, practices, and cultures of different populations.
Coming to grips with health inequities and achieving health equity is a complex and long-term process involving many sectors—both public and private—for many generations. Health systems and the rest of society should shift their focus from disease treatment at the end of the lifespan to preventing social inequities and health differences earlier in life.
LLMs can analyze social determinants of health (SDOH) data like income, housing, and education to predict potential health risks for specific communities. This allows for proactive interventions and resource allocation by clinicians to address those needs. Generative AI/LLM chatbots or virtual assistants can provide culturally sensitive information and connect individuals with appropriate social services and healthcare resources based on their specific SDOH.
These AI virtual assistants can tailor communication and education, providing personalized health information and educational materials based on individual needs and cultural backgrounds, improving understanding and access. LLMs can also identify and flag potentially discriminatory language within medical records, doctor notes, or algorithms, promoting more inclusive healthcare practices.
Clinicians and care teams, the backbone of the healthcare system, often need help with many tasks, leaving them with less time for what matters most: their patients. A virtual clinician could provide the doctor with personalized diagnostics for their patients. This includes analysis of patient data (medical history, lab reports, imaging, etc.) to identify patterns and generate nuanced prompts for the doctor, suggest potential diagnoses and help narrow down the investigation path.
Prior Authorization
Nurses and medical directors need to keep up with lots of data every day to navigate a decision on a prior authorization request. The process can be confusing, tedious, time-consuming, and error-prone. Prior authorization (Figure 4-14) can be a significant problem in the healthcare system for several reasons:
- Delayed care
-
Prior authorization processes can delay patients from receiving necessary medications, treatments, or procedures, as providers must wait for approval from insurance companies before proceeding. These delays can lead to worsening of conditions and poorer health outcomes.
- Administrative burden
-
The prior authorization process is often time-consuming and administratively burdensome for healthcare providers. Physicians and their staff spend considerable time filling out forms, making phone calls, and navigating complex bureaucratic systems, which takes away from direct patient care.
- Increased costs
-
The administrative costs associated with prior authorization can be substantial for healthcare providers and insurance companies. Additionally, delays in care can lead to more expensive interventions if conditions worsen due to a lack of timely treatment.
- Interference with clinical decision making
-
Prior authorization requirements can interfere with a healthcare provider’s clinical judgment, as insurance companies may override a physician’s treatment recommendations based on cost or other factors rather than what is best for the patient.
- Patient frustration
-
Prior authorization can be frustrating and confusing for patients, who may need help understanding why their treatment is delayed or denied. This can lead to dissatisfaction with the healthcare system and potential nonadherence to treatment plans.
- Health inequities
-
Prior authorization requirements may disproportionately affect specific patient populations, such as those with chronic conditions or complex healthcare needs, exacerbating existing health inequities.
Although prior authorization is intended to lower healthcare costs and ensure that the proper services are delivered at the right time, the burden of current processes can hinder timely, effective care. Fortunately, a growing number of healthcare providers, patient advocates, and policymakers are advocating for reform of prior authorization in an effort to relieve administrative burden, improve operational efficiency, and allow clinicians to focus on what matters most: safe, effective, and patient-centered care.
Machine-aided prior authorization with an LLM removes all that while allowing patients to get the care they need, when they need it: the approval process is sped up for proper procedures, while clinician productivity goes up. An LLM is able to replicate human reasoning, vet clinical criteria for almost all care situations that an insurer ever needs to consider, and acquire patient data in real time as needed, including past claims, lab results, prescriptions, and clinical notes. The end result is an LLM-driven system that issues real-time rulings on whether a medical “care necessity” metric was or was not met whenever called upon to do so. All that is left for a clinician to do is to approve or deny a given prior authorization request. In most cases, the clinician could issue a positive prior authorization approval mandate.
Machine-assisted LLM prior authorization enables better decisions quicker by payers, ensuring patients get needed services. Over time, the pain points associated with prior authorizations fade from view, allowing trusted relationships between payors, providers, and patients to blossom. When applied with machine-assisted LLM prior authorization, the healthcare system can work better for all.
As noted previously, a major problem with the prior authorization process is that things must be processed in real time. This is the way it works:
- Manual reviews
-
Because so many prior authorization requests are still adjudicated manually by insurance company staff, they can take a long time and therefore delay treatment. Because adjudications aren’t in real time, patients and providers must wait for decisions, even simple ones.
- Incomplete/incorrect info
-
Incomplete/incorrect info that requires the insurance company to collect info from the provider on the case further slows down the process of adjudication. In real-time processing, this is detected up front, giving providers the opportunity to fill in the information—and even correct the errors—before submitting the PA request the first time.
- Nonstandard criteria
-
Differing rules for approving prior authorizations at different insurance companies and shifting criteria over time are difficult to take into account, with providers having no knowledge of the latest details without real-time processing and a centralized database. A prior authorization is a requirement set by an insurance company that must be fulfilled before medical services can be provided.
- Lack of integration
-
Many prior authorization processes could use further integration with EHRs and other healthcare IT systems. Providers would typically enter information into a separate portal or form, later added to the clinical record, increasing the likelihood of errors and delays.
- Provider and patient frustration
-
Lack of real-time processing can result in significant frustration for providers and patients—delayed treatment, increased administrative burden, and uncertainty about coverage decisions.
Implementing real-time processing in prior authorization could help address these challenges in the following ways:
- Automating everyday requests
-
For routine or low-risk requests, we could allow automatic requests that meet specified criteria for approval immediately.
- Providing instant feedback
-
Real-time processing could give providers instant feedback about the appropriateness and accuracy of their requests, reducing the need for paper ping-pong and appeals.
- Keeping criteria up to date in real time
-
In theory, real-time processing could see health plans continuously updating the criteria providers use during prior authorizations so providers’ encounters are judged using up-to-date information rather than information that might be out of date, which could lead to a denial.
- Facilitating integration
-
Real-time transaction processing could enable integration between prior authorization systems and EHRs, eliminating the need for manual data entry.
Real-time processing of prior authorization is not easy, but it can go a long way toward improving the efficiency, accuracy, and immediacy of the process, ultimately helping patients, providers, and payers alike. LLMs may also improve real-time processing of prior authorizations4 in the following ways:
- Natural language processing (NLP)
-
Imagine an LLM that could make sense of the prior authorization requests you receive in free-form, unstructured language, parsing them for pertinent details like patient name, diagnosis codes, and treatment plans. It could make the process faster and our data input less time-consuming.
- Smart form parsing
-
LLMs could be trained to recognize and parse information from prior authorization forms for various check-ins for different auto, vision, and dental insurance companies. It could allow physicians to submit requests for preapproval in their preferred format, while insurers could then process these automatically.
- Decision automation
-
LLMs could be used together with rule-based systems or machine-learning models for purposes of automating decision making. For example, an LLM could extract relevant information from a request and match against the insurer’s decision guidelines, before making a real-time decision. This could lead to significant efficiency gains in processing routine requests.
- Contextual awareness
-
LLMs could become useful when interpreting clinical documentation, such as notes in the medical record or written clinical justifications in prior authorization requests. In this case, contextual awareness in the LLMs could help ensure that nuances of language don’t get lost and that the analytics are drawn from a holistic interpretation of what’s happening with the patient.
- Personalized communications
-
LLMs could create personalized communications or explanations of prior authorization decisions from providers to patients. Examples include explaining why a request was denied and how to appeal or resubmit the request.
- Continuous learning
-
LLMs can be trained on new data continuously, ensuring they respond to changing guideline thresholds, criteria, and best-care practices. This could enable them to revise prior authorization rules in a flexible way as evidence and standards change.
In the context of prior authorization, while LLMs have massive potential in enhancing real-time processing, it is also evident that this would have to operate in combination with other technologies such as rules-based systems, machine learning/deep learning models, and robust data integration. A robust solution would need data privacy, security, and explainability to ensure all decisions are transparent, fair, and in compliance with relevant policies and regulations.
Summary
With accelerating developments in LLMs and generative AI—technologies that promise to transform medical practice—we embark on an exciting journey to better inform, engage, and connect doctors and patients. In proceeding, we outline several use cases of the emerging promise to suggest how these systems might deliver improved care, better efficiencies in a difficult sector, and an immediate response to significant pressing needs.
One use case could be health bot concierges that would utilize LLMs as a paraphrasing tool in attempts to provide a patient conversing with a machine that has the semblance of a human companion. This companion would be part of the patient journey for a health condition, perhaps from the point of diagnosis onward. An AI companion could address patients’ questions, provide guidance, and help them navigate the healthcare system in a manner that is more amenable to the patient. This companion could deliver the requisite information to steer the care process in a more coordinated fashion while also sparing the clinician that clinical burden.
Within the healthcare sector, clinical workflows can be sped up by the deployment of LLMs and AI programs that can streamline the drafting of doctor notes and visits, affording more time and reducing errors in the development of structured clinical documentation. This would in turn allow physicians to reserve more time for direct patient care. LLMs could also interrogate patient data online to develop improved forms of clinical decision support that can boost and sustain the evidence-based nature of doctors’ decisions and outcomes.
For example, in the realm of health insurance, LLMs can feed robo-advisors that could advise patients about what to look for, and recommend, in terms of health insurance coverage, tailored to individual needs and preferences, thereby helping to demystify the often-obscure world of health insurance, which can be a major roadblock to care—and payment for care—for patients.
Generative AI could therefore help minimize health disparities, increase health equity, and substantially improve health outcomes, especially in populations who suffer from both. For instance, Black women have a higher rate of maternal morbidity or mortality than White women. Using AI and large-scale data, an AI-interpreted intervention could be initially more specific to them and supply them with better access to education and other resources. Similarly, LLMs may power, for instance, medication adherence and oral health promotion programs to improve health outcomes in underserved communities.
LLM-based symptom checkers could help patients more accurately understand and interpret their symptoms and generate recommendations about when tests or care might be appropriate. Given the burgeoning bottleneck of knowledge, LLM symptom checkers might be able to produce more accurate, reliable, and nuanced assessments than currently available symptom checkers, thereby reducing cases of nonutilization of timely care and encouraging earlier intervention in serious illness.
As an example, LLMs offer curbside medicine to clinicians as an authoritative source for evidence-based clinical questions answered using the most recent medical literature. Clinical insight bots for patients can curate, filter, and elaborate on clinical records and summarize relevant questions asked and answers given event by event but geared for individualized recommendations. In addition, digital twins can emulate actual patient behavior in response to nominal interventions under varying conditions (such as precision medicine type protocols).
LLMs and generative AI can be employed for remote patient monitoring to interpret data from wearables and smartwatches/systems, for example. This way, they can identify patterns for prediction and intervention even before patients experience physical symptoms, and they can send alerts to care teams well ahead of time to take action preventing a potential problem from escalating. LLMs and generative AI can also be used to create doctor letters and prior authorizations to help free up clinicians from burdensome administrative tasks that are part of the delivery of care.
We have a moral duty to protect patient privacy and security, and to transition toward embracing ethical tech design and use. In order to harness AI’s generative capacity to benefit patients, developers must avoid siloed efforts and foster coordinated action among healthcare entities and tech developers, as well as maintain a shared vision with policymakers.
In conclusion, if the use of LLMs and generative AI in various aspects of patient care is properly used, we may experience a multitude of changes in future care delivery models, patient encounters, and care optimization.
1 Monique Tello, “Taking Medicines Like You’re Supposed To: Why Is It so Hard?” Harvard Health Publishing, May 10, 2017, https://www.health.harvard.edu/blog/taking-medicines-like-youre-supposed-hard-2017051011628.
2 Madilyn Mason et al., “Technologies for Medication Adherence Monitoring and Technology Assessment Criteria: Narrative Review,” JMIR mHealth uHealth 10, no. 3 (March 2022): e35157, https://www.ncbi.nlm.nih.gov/pmc/articles/PMC8949687.
3 ScribeMD, accessed June 27, 2024, https://www.scribemd.ai.
4 Prashant Sharma, “LLM in Health Care: The Prior Authorization Opportunity,” Medium, August 14, 2023, https://medium.com/@prashant05kumar/llm-in-health-care-the-prior-authorization-opportunity-7e72b6058301.
Get LLMs and Generative AI for Healthcare now with the O’Reilly learning platform.
O’Reilly members experience books, live events, courses curated by job role, and more from O’Reilly and nearly 200 top publishers.