Chapter 4. Preparing People and the Organization for Augmented Analytics
In this chapter, we share best practices for setting up your organization in a way that can support an effective implementation of AA. We’ll focus on roles: business, technical, and in between. We also discuss why your organization needs an analytics center of excellence (CoE) to drive the analytics transformation.
In the context of an analytics transformation, we use the terms role and persona with specific meanings. Role refers to the overarching responsibilities and expectations associated with a broad function or position within the transformation. It captures the general scope and purpose of a particular category of responsibility. A persona, on the other hand, delves deeper and identifies a specific job or position within that broader role. Each persona has its own unique set of tasks, tools, and outcomes. It provides a detailed view of the people who perform the technical or business tasks associated with a role. For clarity, think of a role as a broad category of responsibility while a persona is a detailed, more nuanced representation of a specific position within that role.
This chapter focuses on how to successfully implement what you’ve learned so far about working with people and technical readiness to create the perfect foundation for adopting and using AA in your organization. To help people cope with the changes, it’s essential that you empower and educate them. They need to understand the opportunities, the competitive advantages, and the responsibilities of each individual in this change. Technical readiness, on the other hand, includes the technical data infrastructure, processes, and advanced approaches to meet business demands.
Before we discuss how to raise the data literacy of the whole organization, though, we need to introduce a very effective framework for guiding change from a technical and human perspective: the influence model. Then we’ll explore the fundamental role of data awareness in modern organizations, the art of data storytelling for effective communication, the strategic benefits of data-driven management, and the dynamics of leadership in the age of AI. We’ll discuss the use-case approach, then finish with a plan for enabling, training, and motivating analytics leaders and translators.
Tailoring Augmented Analytics for Different Organizational Roles
This is a transformation made by people, for people. Therefore, you need to address the people in your organization and their roles. All roles are important to a successful transformation; they all have their own requirements, needs, and focus; and they are all interconnected. For example, you will need a variety of data scientists to apply analytics. But their actions are only relevant because the business users’ needs and experiences shape the business and how it makes money.
The skills required to build a successful advanced analytics organization can be divided into three categories: business knowledge, analytical skills, and technical skills, as depicted in the Venn diagram in Figure 4-1. Depending on each role’s orientation and position in the company, the way it combines these categories will vary greatly.
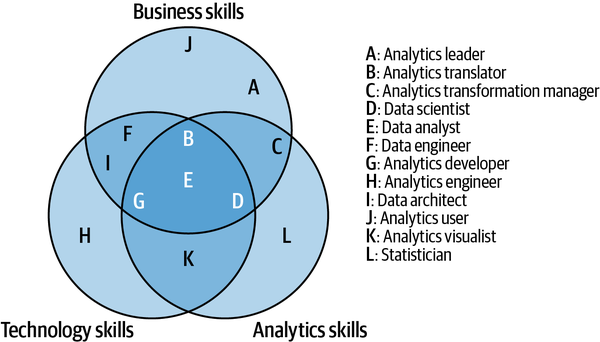
Figure 4-1. Analytics transformation personas in the three skill categories
Not all of these roles are necessary for your team; we just want to give you an overview of possible roles in the analytics transformation. The four top-level roles in the transformation are shown in Figure 4-2. First, leaders have a specific role in the transformation as analytics leaders. Then there are the people who stand between business and technology and perform business analysis. We address them specifically and give them a crucial new responsibility in the transformation: the analytics translator.
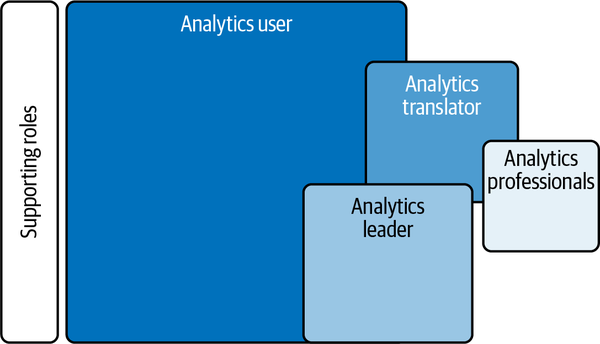
Figure 4-2. The four top-level roles in the analytics transformation
The analytics user uses data analytics to make better business decisions every day. This role could describe nearly everyone in your organization; from portfolio managers to marketing researchers, insurance underwriters to construction surveyors, machine operators to chefs, everyone needs to be able to work with analytical results and become more data-driven.
You can (and should) define these roles in great detail. For example, the fourth role, analytics professional, includes more specialized roles, such as data engineer, data scientist, data analyst, and software developer, as well as specialized support roles, such as transformation manager and data architect. For the moment, though, we’ll focus on broader categories, so we’ll refer to all the roles we just named as data professionals.
As your transformation reaches stage 2, the Data Active maturity level, we recommend focusing only on these four mandatory roles. Later, you can differentiate them more deeply. For now, let’s explore them in detail, beginning at the top.
Analytics Leader
Analytics leaders are the role models who push data-driven transformation, drive the analytical mindset, and champion impactful use cases for business success.
“All good things come from above.” This saying isn’t always right, but there’s a grain of truth to it: the impetus for change is naturally stronger when the leadership carries it forward. That’s why it’s crucial to categorize the organization’s management and executive leaders into one dedicated role, with its own responsibilities and expectations.
Role and responsibilities
All leaders within an organization must embody the principles of analytics and cultivate a mindset centered on data-driven insights and innovative use cases that leverage business objectives. Analytics leaders play a pivotal role in driving the transformation. By setting an example for others, they underscore the significance of using analytics to achieve success in all aspects of the business.
Necessary skills
A well-rounded analytics leader should have sound business and technical knowledge, basic statistical skills, and a fundamental understanding of IT. It is critical that they be familiar with the whole enterprise’s general KPIs, IT landscape, and current projects, and they should understand measures of central tendency and stochasticity. It is also important for them to understand how IT systems interoperate and how the company uses data to drive decision-making processes, enabling the organization to stay competitive and responsive to market changes.
Mindset
Leaders should be intrinsically motivated to contribute to the transformation. There will be leaders who, for some reason, will not be able to go along for the ride, but in most cases, empowering them will help get them on board.
The most important attitude an analytics leader should have is openness to the opportunities and changes this transformation will bring. They should be naturally curious to dig deeper and deeper into the data, question things, and find hidden insights. They must also think strategically about how to align those insights with broader business goals. A good degree of skepticism is just as essential, however, to ensure the quality and reliability of the data.
Adaptability is critical in data analytics, where new methods, approaches, tools, and techniques are constantly emerging; for the same reason, analytics leaders should also have a strong desire for continuous learning. Collaboration is essential, too: data analytics is often a cross-functional, cross-domain discipline that requires effective teamwork and breaking down silos.
Finally, resilience and tenacity are necessary to overcome the inevitable challenges and obstacles that arise in data projects. Many data projects fail. Leaders must learn to manage people’s expectations and help them understand failed use cases for what they are: valuable insights into what works and what just doesn’t.
Importance in driving transformation
Analytics leaders’ vision and use-case ideas are the fuel for change. As role models, analytic leaders will make or break the transformation to a data-driven culture. As you learned in Chapter 3, the top-down approach is indispensable to the analytics transformation. Don’t underestimate the influence of analytics leaders: without them, no transformation could succeed.
Analytics Translator
Analytics translators bridge the gap between business units’ analytics needs and technical analytic expertise, and they translate the insights generated from data into real-world, large-scale impact on the organization.
This second role that we want to introduce is perhaps unexpected, a sort of ambassador who helps connect the business units more closely with analytics. The analytics translator is motivated to be part of the change—after all, this is a voluntary role.
In our experience, the question always comes up about who should fill this role, who is responsible for providing the skills and capabilities, whether these people are organized centrally in the CoE, and whether they are pulled away from their business units, creating an operational gap. Let’s define the role very clearly.
Analytics translators ensure that the business gets real impact from its analytics initiatives. Much like linguistic translators, they communicate ideas and insights between the languages of business and analytics, demystifying buzzwords and interpreting complex algorithmic results in business terms. They foster information sharing, drive adoption among business users, and help ensure that a consensus emerges.
Translators are part of the business. Regardless of where in the organization they are located, they are critical to foster sharing that will improve analytic insights and drive adoption among business users. It is important to build a community to share best practices and lessons learned on a regular basis. Their willingness to collaborate is what breaks down silos.
Role and responsibilities
Analytics translators help analytics leaders brainstorm to identify and prioritize the business problems that will create the highest value when solved by an analytics initiative. These could be opportunities within a single line of business or cross-organizational improvements. Translators ensure that the solution delivers insights that the business can interpret and act on. They communicate the benefits of the solution across domains to drive adoption.
Necessary skills
Translators need excellent domain knowledge; look for experts in the business who have a strong overall understanding of processes across the company. They should be well-versed in the business’s KPIs and other operational metrics and their impact on profit and loss.
In addition, analytics translators need a passion for quantitative statistics and structured problem solving. They are not necessarily dedicated analytics professionals, and they don’t need deep technical expertise in programming or modeling, but they do need to know what models or analytical methods are available and can best be applied to solve a problem.
Translators must also understand the effects of potential model errors that can introduce bias, such as overfitting and outliers. In short, communication is the critical skill in this role. Translators ensure the transfer of results, findings, insights, and knowledge from analytics to business and management.
Mindset
Analytics translators need an innovative, open, and entrepreneurial mindset. Their enthusiasm, commitment, and business acumen help the organization navigate technical, political, and organizational obstacles. Analytics translators also need a tolerance for frustration; they should understand that failure is part of the process.
The most important skill that a successful analytics translator has is in-depth business knowledge. Thus, the best way to fill this role is to train motivated internal colleagues. Building the statistical and technical skills of someone with years of experience is more likely to succeed than trying to impart all that knowledge to new external hires.
There are currently no certifications or degrees for analytics translators. Some companies create their own business-specific analytics translator academies, using business, methodological, and technical immersion. This allows them to adapt their training frequently and respond to the data-maturity level of each individual translator.
Importance in driving transformation
Organized in a community to share experiences of the best practices and lessons learned, analytics translators will be a key factor in the success of individual business units undergoing transformation. This role is indispensable to the team. Establishing this role early and building organizational competence in it will generate strong benefits in the medium term as you move to become a Data Progressive company.
Analytics User
Analytics users are people who consume analytics and use the results to gain and interpret insights, make decisions, and create value.
Role and responsibilities
This role is a more passive one that represents most people in the organization—easily 80%. They are deeply involved in the business and, for the most part, have no direct involvement in data analysis. The whole transformation is done mainly for them.
This is the key group to win over to achieve broad analytics adoption. Your biggest leverage in achieving data fluency is to help these people overcome barriers and be more data-driven in their daily workflows.
Necessary skills
Analytics users’ skills are mainly business related; some may have a slight statistical orientation, which really helps to support problem-solving skills. They must have appropriate analytics awareness.
The challenge is that, because this role is so broadly defined, it is very diverse. You’ll have people whose work is deeply business related and who rarely touch analytics. Others will be directly affected by analytics results—for example, because they directly use dashboards or knowledge repositories. Their levels of maturity in technology and analytics-related skills will be very different.
Mindset
Approaching this role as a unified group will not lead to success. Individual analytics users need different approaches to help them embark on the analytics journey. Unlike analytics leaders and analytics translators—who tend to have intrinsic motivation to contribute, understand the need, and see the change through—most analytics users are likely to be motivated by a sense of compulsion. They are expected to take part in the transformation as part of their jobs. In the beginning, they probably don’t realize how important their active contribution to the transformation is.
Honestly, that’s fine. They just want to do their jobs. A sales manager wants to be a sales manager, an underwriter wants to be an underwriter, and an accountant wants to be an accountant. For them, data analytics is not an end in itself. They mostly don’t care whether their workflows are supported by analytics or digitized. What’s more important is to involve them in the transformation in an appropriate way to increase value contribution, and this will have to go beyond conventional skills training and awareness raising.
It is therefore difficult to say what skills are generally required for this role; the answer is: it depends. But an open mind is a desirable quality in all transformation and change processes, and everyone should have that.
Personas
If you are very early in the Data Active step—for example, providing domain-specific solutions in reporting or BI dashboarding—you’ll need to define your user personas much earlier. By the time your organization reaches the transition from Data Progressive to Data Fluent, you should have a clear picture of how to differentiate the user role in terms of the value streams in your organization and their activities. While you can always proceed without a specific definition, having one will give your work and actions more structure and focus and will make users feel recognized. Each job has a unique perspective as well as a specific set of concerns and potential barriers related to the transformation.
Let’s look at some examples of job personas for analytics users:
- Sales manager
-
Responsible for achieving sales goals and motivating the team, the sales manager relies on analytics to gauge strategy effectiveness, predict trends, and assess team performance. Understanding key metrics, such as lead-conversion rates and regional sales patterns, is critical to achieving targets.
- Marketing specialist
-
The marketing specialist wears many hats, from orchestrating compelling campaigns to understanding customer behavior. Analytics is like a compass for them, showing them what resonates with their audience. Knowing which campaigns deliver the best ROI or how a demographic engages with content can refine their marketing approach.
- Accountant
-
Accountants ensure that the company remains fiscally sound and forward-looking. Analytics provides the foundation for forecasting and strategizing. Looking into detailed financial reports allows accountants to optimize budgeting, understand the nuances of profitability, and make data-driven fiscal decisions.
- Supply chain manager
-
Supply chain managers ensure that products flow seamlessly from warehouses to customers and choreograph the intricate dance of supply and demand. Analytics is their early warning system, alerting them to potential supply chain disruptions, inventory mismatches, or supplier hiccups. A clear snapshot of demand forecasts or supplier performance can streamline operations and prevent costly missteps.
- HR manager
-
Beyond hiring, HR managers are the stewards of company culture and employee growth, ensuring that the organization remains a thriving environment for talent. Using analytics, they can gain insight into the heartbeat of the organization: understanding employee satisfaction, identifying hiring bottlenecks, and charting talent trajectories.
- Customer service representative
-
Key to customer satisfaction, customer service representatives use analytics to evaluate service performance, identify common issues, and improve service quality. Metrics on resolution time and customer feedback guide service enhancements.
- Product manager
-
Product managers drive product development from concept to launch, utilizing analytics to understand customer interactions, feature popularity, and areas for improvement. Insights into user behavior inform innovation and product competitiveness.
The right personas will depend on your organization and its business model. When designing them, try to define different responsibilities and analytics needs. Ask questions like:
-
How does this person deliver to the value chain?
-
What are they responsible for?
-
What are their analytical needs?
-
What challenges do they face?
-
What sets them apart? Do they require individualized handling in your transformation?
For instance, an accountant has different analytics needs than a risk engineer. The accountant is primarily concerned with standard BI descriptive analytics whereas the risk engineer requires assessment instruments, such as potential as-if analysis, and might even use gen AI to summarize complex third-party risk surveys.
Try to answer these questions for the personas of analytics users in your organization. (See the sidebar “Example User Persona: Insurance Underwriter” for an example.) If you identify a specific need for analytics, handle each persona individually.
Importance in driving the transformation
Analytics users are critical to an organization’s analytics transformation as they represent the majority of those who use data insights and apply them to decision making, embodying the shift to an insight-driven culture. Their diverse backgrounds require customized analytics solutions that meet different needs and drive broad adoption and continuous improvement through valuable feedback. Crucially, their role goes beyond technology, and they drive a mindset shift across the organization by demonstrating the benefits of analytics in their daily workflows. The engagement of this group is critical, making them key players in integrating analytics into the company DNA and ensuring the success of the transformation process.
Analytics Professional
Now let’s look at the last of the four roles, the people who implement the transformation: analytics professionals. Analytics professionals are professionally trained in advanced data analysis, statistics, ML, handling data, and/or programming.
Role and responsibilities
Outsiders often imagine this role to be a unified group with unified skills, but of course that’s not the case. Certainly, most of them have strong technical skills, but each individual specialization has its raison d’être and is critical to developing the analytics maturity of the organization. We’ll look more closely at individual personas for these specializations in a moment.
Necessary skills
Despite their differences, analytics professionals tend to be on the technical side of the skill spectrum. Coding, computer science, and programming skills are crucial for them since they translate business requirements into machine-executable forms. Mathematical and statistical skills are also important but are critical only for specific roles. In general, it is important that they have a good understanding of the business.
Mindset
Critical to the success of an analytics professional is a relentless curiosity, a willingness to experiment and push the boundaries of what is possible to uncover hidden insights. They also need to be collaborative and ensure the seamless integration of data insights and business functions. Agile thinking and continuous personal development enable them to adapt quickly to the ever-changing data landscape while their tenacity in problem solving helps them overcome technical and conceptual obstacles.
A great data professional not only looks for the best solution but also has a strong business acumen that ensures their efforts are aligned with the strategic goals and vision of the organization. In doing so, they take responsibility for the value of the solution and actively drive adoption.
Importance in driving transformation
Analytics professionals are key catalysts in an organization’s technical analytics transformation. They are tasked with the dual responsibility of understanding complex business objectives and translating them into meaningful analytical solutions. They meticulously build, refine, and optimize models to ensure that the insights that are extracted from the data are both accurate and actionable. A hallmark of their expertise is their commitment to data integrity, ensuring that data is reliable, usable, and ready for analysis.
However, their role goes beyond technical tasks. They are storytellers who work with the analytics translator to translate complex data results into clear, understandable insights that drive business strategies. In an ever-evolving data landscape, they are also at the forefront of constantly adapting and staying current with the latest methods and tools.
Technical specialist personas
Let’s look at some specialist analytics professionals and their roles on the path to analytics. They are all different, and they are not all equally important at each maturity level. They are also interdependent. To differentiate and structure the professional roles a bit more, we’ll divide them into two groups: technical roles and supporting roles. The technical roles are the ones most laypeople imagine—data analysts, statisticians, data scientists, and data engineers, among others:
- Data scientist
-
Develops advanced models and algorithms to extract insights and predictions from data. Data scientists are more on the mathematical and statistical side of the skill spectrum. They tend to have limited programming skills compared to analytics developers and data analysts but are great with ML and statistical analysis.
- Data analyst
-
Derives insights by querying data, performing statistical descriptive and diagnostic analysis, reporting, and visualizing data. Data analysts often use tools such as SQL, Excel, and BI platforms as well as analytical programming languages like Python and R. They typically have an intermediate level of business understanding and are a good intersection between business translators and technology.
- Data engineer
-
One of the most underrated technical specialist roles, the data engineer prepares “big data” for analytical or operational use, enabling the work of data analysts and data scientists. Data engineers manage and optimize data lakes and databases so that data can be stored, queried, and piped effectively, and they ensure the accuracy and availability of the data. They build, maintain, and optimize the data pipelines and architecture.
- Analytics developer
-
This role develops, implements, tests, and integrates analytics solutions and applications, web applications, and API web services. Analytics developers work closely with analysts and scientists and translate analytical insights into programmatic packages, engines, and software.
- Analytics engineer
-
Ensures that ML models and analytics solutions are seamlessly deployed, scaled, and maintained. DevOps is the analytics engineer’s field of action. They are typically required at a later stage in the analytics value chain and are critical during the late stages of the transformation.
- Analytics visualist
-
Specializes in representing data and insights visually, often using tools such as Tableau, Power BI, and Markdown reports. Analytics visualists specialize in explaining, rather than exploring, data. They have a flair for design and storytelling, so communication is a big part of their skillset. They often design dashboards and user interfaces for analytics applications.
Support specialist personas
There are also some supporting roles that are critical to the transformation. Please keep in mind that this list is by no means complete:
- Data architect
-
Responsible for designing and implementing the data infrastructure, ensuring scalability and accessibility. Data architects design the new future-proof technology stack and define operational environments for analytics solutions. They don’t have to be based in the CoE but should be closely aligned with their counterparts on the IT side.
- Data governance officer
-
This role ensures data quality, privacy, and regulatory compliance and acts as the guardian of quality. It focuses on ensuring that analytics insights are based on a reliable foundation. Data governance during transformation is a complicated task. Data governance officers must participate in inspiring people and fostering the creative mindset that a transformation requires but balance this with compliance, regulations, and policies—responsibilities and tasks that are not much fun. They will need support to fulfill this critical role.
- Change management specialist
-
This role helps the organization adapt to the new data-driven culture, addressing resistance and facilitating training. Change managers are critical to any change in an organization, especially when their methodology is applied to the analytics journey. Many people will struggle with the changes of becoming data-driven. Helping people find their place in the change is essential to the new culture you want to establish.
- Business analyst (BA)
-
This role often works closely with analytics translators to define business problems that analytics can solve. Analytics translators are mainly taking over this position in the analytics transformation, but BAs are often established in an organization for IT requirements. As mentioned previously, BAs can even become analytics translators if they are located in the business rather than in specialized capability units.
- Subject matter experts (SMEs)
-
Specialists in specific business areas. SMEs provide context and expertise to ensure that analytics solutions are relevant and actionable, working alongside BAs and primarily supporting analytics translators. SMEs typically come from the business units; they don’t necessarily have close contact with the CoE and aren’t really involved in analytics. They are excellent candidates for the analytics translator role.
- Analytics transformation manager
-
The most critical supporting role and the driving force behind the transformation, located within the CoE. If you have a good transformation manager and a supportive transformation team, you’re already halfway to a successful transformation. In fact, this role is so important that we’re going to give it its own section.
Analytics Transformation Manager
Analytics transformation managers play a pivotal role in driving the strategic vision and ensuring that the organization successfully evolves into a data-driven entity.
You might have one transformation manager or a few. Figure 4-3 illustrates just how broad their field of action is. Finding the right person will be challenging but well worth it.
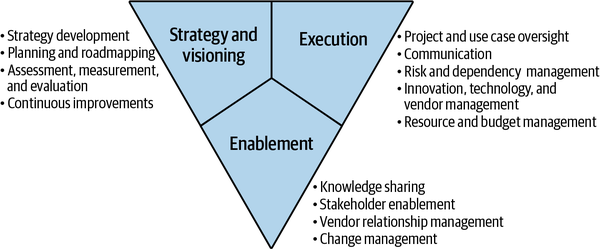
Figure 4-3. Fields of action for the analytics transformation manager
Role and responsibilities
Analytics transformation managers orchestrate and enable change. They work closely with stakeholders, organize awareness initiatives, and act as the first point of contact and spokesperson for the CoE. In later stages of the transformation, their responsibilities expand to include managing resources, mitigating risk, and ensuring profitability. Whereas the change manager role focuses on mitigating the impact of a change in progress, the transformation manager actively shapes the strategic vision.
Strategy
This role works with senior leadership to define the strategic goals of the analytics transformation and align them with overall business objectives. Transformation managers plan how to achieve those goals by creating a comprehensive roadmap that outlines milestones, timelines, and resource allocation.
Measurement
Transformation managers define, track, and monitor KPIs and other metrics of the transformation’s success and impact. After the initial transformation, they evaluate its ongoing impact, identify areas for further improvement, and ensure its sustainability.
Execution
Transformation managers must monitor the progress of analytics projects and initiatives to keep them on track and within scope. They determine how the transformation is communicated internally and externally. They identify potential risks, dependencies, and other challenges and develop strategies to mitigate them. They manage the transformation budget, allocating human and technology resources and ensuring that they are used efficiently and effectively. They seek out and integrate innovative tools, methodologies, and approaches to enhance the journey of the analytics transformation and manage vendor relationships. They also advocate for the responsible use of data, ensuring that the transformation respects both legal boundaries and ethical considerations.
Enablement
Transformation managers develop and implement change management strategies, engage with stakeholders across the organization to build support, and facilitate knowledge sharing and collaboration across teams and departments. They establish mechanisms for regular employee, stakeholder, and even customer feedback and use the feedback to guide and assess the transformation process.
Necessary skills
Transformation managers need a wide range of management and cultural skills, in addition to their technical and strategic competencies. These “soft skills” include leadership, communication, collaboration, problem solving, and adaptability. The transformation manager doesn’t have to be a technical expert but should have enough of a foundation in the latest analytics innovations, technologies, methodologies, and best practices to make informed decisions.
Successful transformation managers need a visionary outlook; they must anticipate trends and prepare the organization for what’s coming. Because not everyone in the organization may understand analytics or what it can do, the transformation leader must also have the skills of an educator and coach, helping people at all levels understand the value of the transformation and how to get involved.
Mindset
Transformation leaders need a mindset of continuous improvement. They must champion the cultural shift and even become the face of change, embodying the values and behaviors that the organization is trying to instill. They must embrace challenges and drive change with passion and ownership. And since the journey of transformation is fraught with many challenges, they should be resilient, able to overcome setbacks and keep the momentum going.
Importance in driving transformation
On the ambitious journey of analytics transformation, the analytics transformation manager becomes the linchpin of success. This role goes far beyond mere project management—it’s about designing a data-driven future and paving the way to get there.
The manager’s expertise lies not only in strategy formulation but also in orchestrating all implementation activities. Transformation managers bring together stakeholders from the C-suite to the frontline to ensure collective buy-in by bridging communication gaps. They demystify analytics, transforming it from an intimidating concept into an accessible, valuable tool for everyone through targeted awareness efforts. To do this, they organize professional development initiatives to ensure that the workforce is ready for the new data-centric landscape and to create a broad understanding of the value of analytics to the business.
Without analytics transformation managers, an organization’s journey to analytics maturity will remain essentially unorganized and disjointed. They are the face of the transformation, and their passion for the transformation serves as a model for many others who are adapting it for themselves. Finding the right person will be challenging but well worth it; a good transformation manager and a supportive transformation team will be halfway to a successful transformation.
Summary of Key Roles
Table 4-1 summarizes the four key roles of analytical transformations. Remember that all of these roles are required for success.
Analytics leader | Analytics translator |
Role and responsibilities: Drives an analytics mindset, aligns with business goals, and formulates ideas for their specific business area. | Role and responsibilities: Connects technical and business teams, extracts insights from data, and collaborates across organizational centers of excellence. |
Necessary skills: Profound business acumen, basic statistics knowledge, IT understanding, and awareness of IT system interdependencies. | Necessary skills: Solid business acumen, proficiency in mathematics and statistics, and foundational computer science skills. |
Importance in driving transformation: Leads analytics projects and sets direction for business unit transformation. Essential for fostering a data-driven organizational culture. | Importance in driving transformation: Bridges the gap, aligning analytics with business objectives and translating complex data into actionable business insights. |
Analytics professional | Analytics user |
Role and responsibilities: Key players in analytics transformation, including data engineering, analysis, and science. | Role and responsibilities: Making data-informed decisions in their daily roles, even if not directly involved in the analytics process. |
Necessary skills: Proficient in computer science, math, and statistics; understanding of business for effective stakeholder communication. | Necessary skills: Business domain knowledge; basic IT and statistics understanding can improve their ability to interpret analytics outcomes. |
Importance in driving transformation: Essential for executing complex data tasks, they develop and oversee data-driven decision systems. | Importance in driving transformation: Applying data insights to business challenges; driving the success of analytics in decision making. |
The analytics user group, easily the majority, is the key group to win over for broad analytics adoption. Helping these people overcome typical barriers to becoming more data-driven in their daily workflows is a major source of leverage.
The Center of Excellence
A CoE is, in short, a unit dedicated to organizing the analytics transformation. During the Data Active maturity phase, it collects and connects all the loose threads of analytics activities that emerge.
Creating a Center of Excellence
Your transformation will need a CoE. Without it, you will not reach the Data Progressive stage.
CoEs are often built from the sophisticated analytical capabilities of established business units. We recommend identifying these areas and units early on. The CoE is usually initiated by the chief information officer or chief operating officer, with the commitment of board members who understand the relevance of analytics to the business.
The CoE should not necessarily bundle all data and analytics functions together. In fact, we recommend separating responsibility for data analytics from responsibility for data management, data governance, and data infrastructure. There is no question that these units, departments, and responsibilities need to work very closely together, but as Julius Caesar once said, “Divide et impera”: divide and conquer. The overall transformation is so large that one unit will rarely have all the necessary skills; in attempting to handle everything, such a unit quickly loses focus and connection to the business.
A good division, as mentioned previously, is between data management, BI with standard reporting, and analytics skills with data science. The CoE comes into play at a later stage of maturity, as we mentioned, especially in the transition from the Data Active stage to the Data Progressive stage, which involves intense transformational tasks.
For this reason, the CoE’s staff rarely comes from IT. In fact, we strongly recommend developing the CoE from within the business rather than from within IT. If you don’t have dedicated and easily data-ready business units capable of driving the transformation, then you should look to IT to handle the responsibility. But remember that the whole transformation is about supporting the business and breaking down silos. Ideally, you want a dedicated CoE team to emerge from the most data-savvy and analytics-savvy departments so that the team fully understands the problems and needs of the business units.
There’s an adage in computer science called Conway’s Law that states: “Any organization that designs a system (defined broadly…) will produce a design whose structure is a copy of the organization’s communication structure.”1 In this context, this means that setting up a CoE on the IT side keeps the skills and responsibility on the IT side. If you really want to break down silos, though, you need to bring the responsibility of becoming data-driven close to the business by setting up your CoE on the business side.
However, this raises a fundamental problem. We’ve established that the CoE does not handle all analytics issues and challenges; depending on how you divide the work, data management (and possibly other functions) will be handled in a different department or business unit. When you initially staff the CoE, you draw experts away from those business units. This weakens them for a time, but the division managers of those units must remember that this is an investment in achieving the overall goals of the company.
At HDI Global in 2019, for example, we decided to take this approach. The company was setting up a completely new CoE team, on the business side and closely linked to the business units, and drew its initial staff from its data-based areas: Natural Catastrophes, Underwriting, Controlling, Consulting, and Risk Management.
If you don’t have the appropriate analytical and technical skills on the business side, bring in some people from IT, but you should still give more weight and focus to the business side, as shown in Figure 4-4. People from the business units will always have a stronger connection to, and thus stronger credibility with, future stakeholders there.
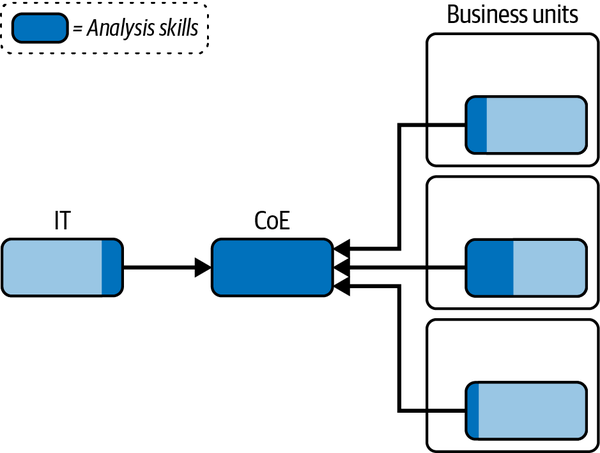
Figure 4-4. A center of excellence drawn from both IT and business units
Your next challenge, then, is convincing the business units to give up their best data experts to staff a new CoE. Here, communication is king. Connect your request to your common understanding of the company’s mission. The manager certainly won’t be happy, but they will understand the necessity.
Look for people in the company who have a good reputation outside their fields and who are respected for their expertise. If you can persuade them to participate in the CoE, or perhaps support it personally, others will follow their lead. Again, don’t underestimate the importance of role models in the overall transition.
Above all, though, convince the people you want to recruit. Some people may feel insecure about leaving their comfort zones, where they have the strongest expertise. They may have reservations about whether the company will be able to sustain its cultural change in the long term.
Your new team will need to establish a dynamic together as a team, and that takes time. We recommend that you emphasize their previous successes in their current domains and let them know how important their skills and abilities are to the company’s success in this important step in the transformation. These people will make the difference. Make sure they realize that this is an opportunity to shape the future success of the company.
Defuse any possible fears of not being strong enough to really take the next step here. Show them that the mindset has changed and that the company is ready for the transformation.
Approaches to Organizing a CoE
To help you understand how the CoE fits into the organization, this section looks at centralized and decentralized approaches and which work best, especially in the Data Progressive maturity stage (stage 3).
The decentralized approach
Usually, in a Data Reactive (stage 1) organization, one at the beginning of its analytics journey, you will find centralized capabilities for data and reporting on the IT side and some of these capabilities in the business units—some of which have advanced data savvy, some of which do not. While the non-data-savvy business units are totally dependent on IT solutions, the more sophisticated business units approach data and insights in their own way. They are agile in responding to their own domain’s needs, innovating, and coming up with new ways of doing things. This can result in disconnected and unaligned domains, but it also provides the highest degree of independence.
The decentralized approach can be very tempting, but its disadvantages for the organization outweigh the advantages. Domains’ development capabilities and design know-how for sustainable analytics infrastructures and processes may be insufficient, which can lead to operational inefficiencies.
For example, risk management departments are often staffed with mathematicians: well-trained analytical thinkers with strong problem-solving skills. It is not uncommon for them to start optimizing what they do with the skills they have at hand, usually Excel and VBA. In the best cases, they may use a functional programming language like Scala, R, Python, or SQL. They tend to produce semi-automated solutions for calculations, data transformations, and so on that solve their current problem. However, the solution often can’t be scaled because they’ve never learned how to design an analytical solution with interdependencies on other domains or on organization-wide processes and systems.
Business units operate independently when it comes to data and analytics, with a strong focus on addressing specific business needs. There is often weak governance and unclear ownership as well as few opportunities for other units to benefit from these solutions. Collaboration and support among units are weak due to the lack of a unified strategy. The risk of creating significant silos within the organization increases as a result of this disjointed approach. These shortcomings need to be addressed, or they will become an obstacle to transformation.
Maybe you’re a little confused now, because we mentioned that decentralizing analytics is one of the most important enablers to becoming data-driven. It is, but in a different, more organized, and more collaborative way: a federated approach.
The centralized approach
But first, the other extreme is a completely centralized approach to analytics ownership. This approach is most common in smaller companies, and it can be a perfectly valid business model for them. This approach is often taken where there is little or no technical or analysis capability in the business units, either because they are highly involved in repetitive, simple, manual work or because there are just a few experts who are highly focused on a specific issue that offers little automation potential and usually has little to do with analysis. It is common to have a tightly centralized CoE that handles all the core functions, including standard reporting.
The biggest advantage is clear: well-trained developers can design the best, most efficient infrastructure and data-handling processes. However, they lack business knowledge and insight into customer needs. To successfully apply this operating model, the guiding principles should be close cooperation, a manageable number of people on both sides, a strong commitment to working together, and giving the same amount of attention, priority, and effort to all issues, major and minor. The latter is one of the biggest challenges because a centralized unit will always be a bottleneck.
A centralized CoE must prioritize its efforts to manage its limited capacity. It may lag in emerging changes in the organization and struggle to act quickly on them. Centralization rarely translates to shared responsibility and ownership of data delivery across the business. Often, IT lacks insight into business interdependencies and the specifics of the CoE’s solutions, leading them to resist taking full ownership beyond the technical part of the solution.
The federated approach
We’ll now introduce you to the federated model, an approach that falls between the two extremes—a model we believe is the only way to successfully combine ownership, technical sophistication, and collaboration. This model will ultimately take you to a higher cultural level and gain value for the business.
There’s no single definition of a federated system, and it manifests differently in different organizations depending on whether the central CoE or the decentralized units are more pronounced and how far along the organization is in its transformation.
In short, though, a federated model is one in which the CoE drives the transformation centrally at the beginning, but then the organization shifts toward a stronger decentralized federated model as its data maturity grows.
At the outset, many tasks are unclear. The technology is not fully ready, collaboration models are undefined, and a common analytics governance model is not yet in place. The CoE can address this by demonstrating how to tackle use cases and initiating programs to improve data literacy or at least analytics awareness. We thus recommend starting the CoE with a more centralized approach, then moving toward decentralization as you assign roles within the business units and as you establish appropriate data literacy within the business units.
This shift also lays the groundwork for transferring more responsibility to the business units, which helps to democratize data: with stronger analytical capabilities, business units can become data providers, not just consumers. This is the idea behind the data mesh, a data architecture concept defined by Zhamak Dehghani in her book Data Mesh (O’Reilly). Dehghani describes data mesh as shared responsibility for data products across business domains. Treating data and insights as assets that deserve proper ownership, she argues, leads organizations to a higher level of data culture.
But let’s take a step back. Why is it so important to decentralize analytics at a higher level of maturity, as pictured in Figure 4-5?
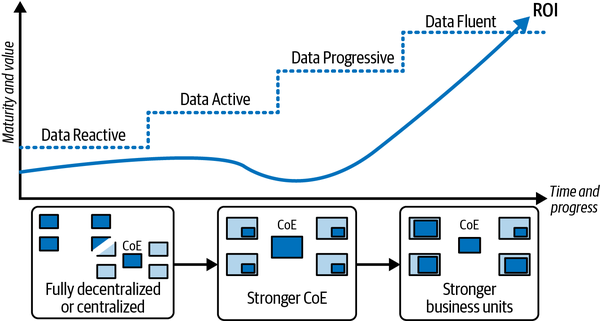
Figure 4-5. Types of federated models by maturity level
First, as awareness of analytics has increased, organizations have gained a greater understanding of the opportunities to leverage analytics for their business and are keen to become data-driven. This leads them to generate more ideas for insights they want and more requests (and demands) for analytics solutions. Ultimately, if they’re operating from a centralized position, they can’t meet these demands. They end up evaluating, prioritizing, and weeding out ideas because of a lack of capacity.
The advantage of a federated model is that if you empower the business units to handle most of their needs, you increase their sense of ownership of their problems and their ability to create value for the whole organization. This is a good feeling, and it’s important for establishing a new culture.
In addition, business units mostly do common descriptive and diagnostic analytics tasks. In a federated structure, the CoE doesn’t need to deal with these simpler tasks. It can focus on the really challenging issues and on supporting weaker business domains. The business units, meanwhile, are better able to combine their new analytics knowledge with their understanding of the business and its needs. It saves a lot of time and effort to do the thinking yourself rather than spending hours explaining it to a third party.
Be aware, though, that increasing federation requires stronger governance, or at least guidance and a commitment to a common framework of regulations and the limitations of what each unit can do.
It’s up to you how you design your federated approach. We recommend a distributed, decentralized approach such as federation collaboration, which fosters efficiency, ownership, and a strong sense of togetherness. However, there is a spectrum of federated models. Look for the sweet spot that fits your organization’s size, business model, staffing, strategic vision, and maturity. Find out what you need and where you stand: is your CoE the transformation driver you still need to kick-start the transformation and get everything rolling, or is it now a supporter, helping business units become excellent on their individual analytics journeys?
The first is characterized by the fact that, as the lead unit driving the analytics transformation, the CoE plays a pivotal role. Business units have begun to nurture and develop their analytics capabilities and expertise under the strong guidance of the CoE. While these units manage foundational tasks, the responsibility for the execution, implementation, and application of analytics, data, and relevant use cases remains firmly within the purview of the CoE.
The second is defined by the fact that the CoE operates as a compact, specialized unit. Its primary focus is to provide guidance. Acting as a facilitator, the CoE spearheads key initiatives, such as centralized learning, ensuring technical readiness, and conducting technology research. While capabilities are shared between the CoE and the business units, the CoE holds the overarching responsibility for ensuring that the transformation succeeds. However, the business units are responsible for use cases and analytics specific to the business domain.
Figure 4-6 explores the different levels of federation. The next section will help you better understand their pros and cons as well as how your approach might change over time as your analytics journey matures.
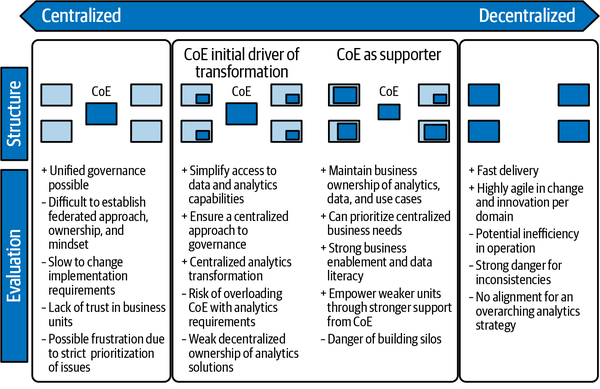
Figure 4-6. Federated business models throughout the analytics transformation
To drive a common analytics strategy, you need a CoE. This is critical. Its existence is a strong indicator of a more mature analytics transformation. A completely decentralized or fully centralized solution, on the other hand, is a strong indicator that a company is operating at stage 1, the Data Reactive maturity framework.
If your organization’s goal is to become Data Progressive (stage 3), choose a model somewhere in the middle. We don’t recommend starting with a fully (or almost fully) centralized approach. If the business units are strongly disconnected from analytics, you might have little choice but to centralize. But even then, always try to involve the business units in the CoE’s activities as soon as possible. Gaining the business units’ commitment, awareness, and curiosity will be critical. Therefore, try to quickly shift the initial full responsibility for use cases and their implementation from the CoE to a combination of both models, and at least transfer ownership of analytical use cases to the business units.
The more shared responsibility you can give the business units, the easier it will be to build people’s interest in data skills and leverage the analytics transformation for business success. But this will be a marathon and not a sprint; you will need a lot of perseverance and patience.
The CoE’s main role is to drive analytic transformation across the enterprise. Its tasks include:
-
Ensuring data liberalization
-
Creating analytics awareness and a bring-along data competency
-
Building a “lighthouse” use-case execution pipeline to get the organization thinking analytically
-
Collecting analytics opportunities that offer quick wins and low-hanging fruit to create momentum
-
Working closely with the business units and data management and connecting their strategies
-
Creating an analytics culture in the company
-
Convincing the most ambitious and passionate people in the company to take ownership of the transformation
In summary, you absolutely need a CoE to reach the Data Progressive stage of maturity. We recommend a federated approach, starting with a strong CoE at the beginning, then decentralizing more and more once the business units are fully involved.
Driving Transformational Change with the Influence Model
An analytics transformation is a serious, often difficult organizational change. Many transformation initiatives fail, often due to human factors. Employees—the backbone of any organization—must understand and fully embrace the change for it to take root and succeed.
This is where the Influence Model comes in: a strategic framework based on sound academic research, first introduced by McKinsey in 2003. The Influence Model addresses the core of successful organizational change: the human element. It’s not just about having a plan or implementing a new software system; it’s about influencing mindsets, shaping behaviors, creating cohesive understanding, and helping people believe in the organization’s direction. This model focuses on four key areas, shown in Table 4-2:
-
Fostering understanding and conviction
-
Reinforcing change through formal mechanisms
-
Developing talent and skills
-
Role modeling
“I will change my mindset and behavior if...” | Principle |
---|---|
“...I understand what is being asked of me, and it makes sense.” | Fostering understanding and conviction |
“...I see that our structures, processes, and systems support the changes I am being asked to make.” | Reinforcement with formal mechanisms |
“...I have the skills and opportunities to behave in the new way.” | Developing talent and skills |
“...I see my leaders, colleagues, and staff behaving differently.” | Role modeling |
Each of these key areas addresses the psychological and behavioral aspects of a change to ensure not only that the change is implemented but that employees internalize it.
At its core, this model is about understanding the “why” behind change and managing that change effectively. The Influence Model provides the tools to help you do this. For each initiative or change process you undertake, carefully consider the four “building blocks” outlined in the next sections.
Fostering Understanding and Conviction
Humans naturally seek consistency between their beliefs and their actions. A common challenge in change is assuming that everyone already knows why the change is happening. Two notable psychological tendencies contribute to this: the false consensus effect, where people overestimate the extent to which others share their own attitudes, beliefs, and opinions; and the curse of knowledge, where people find it difficult to imagine that others don’t know something they know.
So what’s the solution? Leaders must create a compelling change story and establish feedback loops to ensure that everyone understands it. Digital platforms and awareness-raising initiatives can help engage employees in these changes and make them feel that they’re a part of the journey. If everyone isn’t convinced about the transformation, the change will be over before it has really started.
Reinforcing with Formal Mechanisms
Our behaviors are generally the result of reinforcement and conditioning. But employers often go about this in the wrong way, without aligning rewards and desired behaviors. For example, rewarding employees financially may work up to a point, but money is probably not the ultimate motivator; other factors, like purpose and collaboration, may have a greater impact. How you use these rewards matters, too: they can be less effective if they’re too predictable. It’s best to make rewards fair, varied, and truly purposeful.
Moreover, the organization must improve the working environment in which employees operate, which encompasses working conditions, restrictions, and culture. If the “new” environment offers the same old structures and processes, you can’t expect behavioral change.
Developing Talent and Skills
Humans are capable of learning and adapting throughout life. But a few things can get in the way of that process:
- Inherent bias
-
Many of us are unaware of our knowledge gaps because we are inherently biased. We don’t know what we don’t know.
- Perceived solvability2
-
People often assume that complex problems can be solved somehow, without actually tackling them and testing the approaches. This is particularly true in the AI environment, where the underlying technology accomplishes wonderful things in vaguely understood ways. It’s important that people understand how problem solving actually works, because finding a solution usually involves a lot of hard work.
- Learned helplessness
-
Repeated mistakes or negative experiences can lead individuals to believe that they cannot change, creating a state of “learned helplessness.” Organizations must instill the belief that effort can lead to improvement. With the advent of analytics, there are more opportunities than ever to demonstrate this in action.
Role Modeling
People naturally imitate others, both unconsciously (such as mirroring others’ speech patterns) and consciously (such as deliberately adopting a particular behavior). While many think of role models in terms of historical figures or celebrities, influence within organizations can come from unexpected sources. In our digital age, people often value the opinions of crowds, such as when we trust Yelp reviews or read posts by LinkedIn users with large followings.
What this means for transformation is: don’t underestimate the role of people with large “circles of influence.” Convince these people early on of the transformation’s purpose and the opportunities it brings. Their advocacy will help to embed the transformation more quickly and sustainably in the minds of others, and thus drive it forward. This is why analytics leaders are so important; they lead by example. How they behave will be how the organization behaves.
It is surprising how often these critical elements are overlooked during major organizational change, even though the fundamentals of the influence model are rooted in human nature. For successful change, all of these elements should be addressed holistically. They may seem like common sense, but in the chaos of change, even the basics can be easily forgotten.
Any time you introduce a new initiative, solution, or change, we recommend asking yourself if all four elements are being adequately addressed. For example, when introducing a new use case, you might ask:
-
Have I made the purpose of this use case clear?
-
Can people use the results of this use case properly?
-
Does embedding these processes ensure easier access to insights?
-
How do I “reward” the early adopters?
-
Do I have the support of people with influence?
This sort of cross-checking helps you to test your own actions and course correct if necessary. Use this framework. It really is very powerful.
The Influence Model is critical to supporting analytic transformation in organizations. It focuses comprehensively on the human element that is key to the success of such initiatives. By addressing the psychological and behavioral aspects of change, the Influence Model ensures that employees not only adopt new technologies and processes but also internalize these changes. This creates a more receptive environment for change by addressing key causes of restructuring failure. It aligns employees’ behaviors and attitudes with business and transformational goals, overcomes resistance, and improves overall business performance. Changing the environment in which you operate is only half the battle.
Cultivating a Data-Literate Culture
We divide the big topic of data literacy into four subtopics (Figure 4-7): data awareness, data storytelling, the strategic benefits of data-driven management, and the dynamics of leadership in the age of AI. Each of these topics equips analytics leaders and translators with the skills they’ll need to lead their organizations through the transformative power of data. This educational journey is about creating a data-centric culture that thrives on informed decision making and continuous innovation.
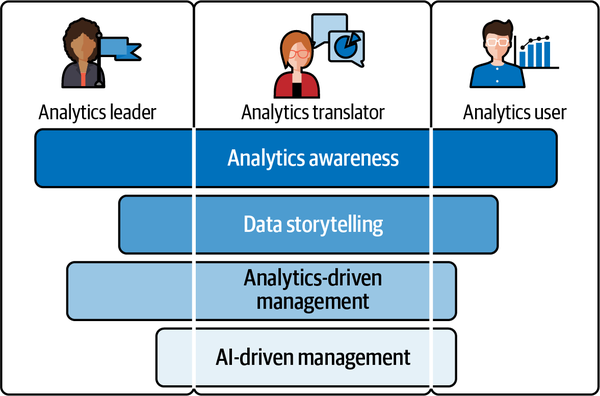
Figure 4-7. Four key aspects of data literacy
You may be wondering: how is data literacy even necessary if AA is just being integrated into your business workflows? The answer is simple: you need a high level of data literacy—especially among your analytics translators—to be able to find and develop the use cases that should augment critical business workflows in the sense of AA. On the other hand, analytics users—the masses—don’t need a high level of data literacy to benefit from these workflows. But they need something called “analytics awareness,” a concept that we’ll explain in the next section.
That’s why each role requires different training. Analytics leaders should focus (at the least) on analytics awareness and data-driven management while analytics translators need the full range of this training. For analytics practitioners, awareness and storytelling provide a solid foundation for analytics adoption, although the skills required will vary by position and role, as you can see in Figure 4-7. Let’s take a closer look.
Cultivating Analytics Awareness
The evolution to data-driven decision making requires people who aren’t analytics professionals to understand the full spectrum of data technologies and analytics processes, so they can communicate effectively with analytics professionals and use data to gain strategic advantages. The primary goal of data awareness is to ensure that they recognize the value of high-quality structured data.
At the heart of data awareness is understanding the data analytics process: a complex journey from data collection to analytical insights. As you, as a business professional, immerse yourself in this area, you’ll become adept at ensuring the integrity and maximizing the quality of data.
Immersing yourself in the world of data also means becoming familiar with how to visualize it. Analytics leaders, translators, and users can study best practices to learn to transform complex data sets into clear and compelling visual narratives. Each visualization serves as a critical link between data and decision making.
The data-awareness journey continues with the concepts of AI, ML, and deep learning. Understanding these terms and their applications allows nonanalytics professionals to critically assess the capabilities and limitations of AI, foster constructive dialogue with data scientists, and formulate realistic expectations for AI projects. This includes treating responsible and realistic use of gen AI tools, especially LLMs like GPT, as a core competency, given that they are now part of business professionals’ everyday toolbox.
As the digital and physical worlds merge through IoT, sensors and smart devices are contributing to the data explosion, with unprecedented opportunities to improve processes and create value.
Storytelling with Data
In an era of data saturation, the ability to tell a compelling story is paramount. Data storytelling bridges the gap between raw numbers and effective communication by balancing statistical rationality with narrative clarity. This skill is essential for professionals who need to present complex data findings to different audiences within the organization.
Again, it helps to first understand the purpose, the “why,” behind data storytelling. It’s a preparation for a deeper dive into the “how”: the methods and techniques that turn data into compelling stories tailored to the needs of the audience and the goals of the presentation. Business professionals must learn to communicate with data in a way that is appropriate for their audience, mastering the subtleties of tone, context, and character to deliver presentations that resonate with and influence their audiences.
As they hone their storytelling skills, professionals also become curators of data narratives. They distill vast amounts of information into cohesive stories, using techniques such as storyboarding. This structured approach ensures that key messages are conveyed accurately and that audiences remain engaged and informed.
Mastering visual tools is another essential part of data storytelling. Charts and visual formats all have their strengths and applications. Choosing the right visualization is a strategic decision that can significantly improve how well your audience understands and absorbs information.
Decluttering, or removing the unnecessary to focus on the essential, isn’t just a design principle; it’s also a critical communication strategy. By learning to declutter visualizations, professionals ensure that data presentations are not only aesthetically pleasing but also psychologically compelling, focusing the audience’s attention on what really matters.
Data visualization is certainly less a science than an art, but it is an important part of the data literacy skillset of people working in the analytical environment and should not be neglected.
Embracing Data-Driven Management
Becoming a data-driven organization is more than a technology upgrade: it’s a fundamental change in decision-making culture. For managers, this shift requires a deep understanding of data’s intrinsic value and the practical know-how to use it for strategic purposes.
From the outset, managers must learn the benefits of data-driven decision making and recognize the central role of the analytical value chain in refining raw data into strategic insights. This understanding is critical to recognizing how data can go beyond locally scoped operational support to become a core business asset and even pave the way for optimized processes and innovative data products. A central part of this transformation is committing to an analytics strategy. Once managers commit to and begin implementing the strategy, they can gain insight into common pitfalls and learn how to overcome challenges with strategic vision and operational acumen. They will also be empowered to optimally align their efforts with the individual business strategy.
Data integrity is critical. Managers have a special role as the guardians of data quality. They must understand how the integrity of a business’s data affects its health and work proactively to maintain that integrity.
Managers are also concerned with the human factor: building and maintaining data ownership, addressing capability gaps, and promoting a culture of continuous learning and improvement.
Leading in the Age of AI
As the final competency, data thinking encompasses the strategic mindset required to integrate data into the DNA of the organization. Leaders explore the synergy between design thinking and data analytics, using tools such as the Analytics Use Case Canvas to generate ideas and align data initiatives with business goals and user needs to shape a data-aware organization.
AI, once a distant concept, is now an integral part of the business conversation. As AI reshapes industries, managers and executives in particular need to move from mere awareness to active engagement and understand AI’s potential to drive innovation and profitability. This educational journey begins with demystifying AI and its complexities. From there, leaders can learn to identify and nurture AI opportunities, anticipate the challenges associated with AI initiatives, and create an environment in which AI can thrive. This foundational knowledge sets the stage for leaders to build an AI strategy as an extension of analytics strategy and project management, ensuring that AI investments translate into tangible business results.
The project-based learning competencies give managers the methods to manage the lifecycle of AI projects from idea to implementation. It is a practical roadmap, a use-case approach to AI project success that covers use-case development, proof of concept, and the intricacies of AI engineering and operations.
The culmination of this journey is business integration. Executives should tap into the wisdom of AI thought leaders by integrating best practices into a cohesive AI and business strategy that can guide the organization through the complexity of AI adoption, providing the necessary capabilities, technology stack, and management practices to leverage the transformational power of AI. By understanding and communicating this to your leaders, you will lay the foundation for a successful AA implementation.
Although many of the upskillings describe skills very much focused on new management competencies, these should be opened up for all analytics roles. Acknowledging how leaders act and why will help them adapt and strengthen them as role models. Through these learning focuses, analytics leaders and translators will not only be educated but also empowered to drive analytics transformation, foster data literacy, develop a data-centric culture, and lead their organization into a future where data and AI are an integral part of success. They are the ones who ultimately must identify, evaluate, and drive the value of use cases for the organization, and it is absolutely essential that they are fully prepared.
The Enablement Program
Data literacy alone is not an enabler. You need to embed it in an enablement program. This section describes different programs geared specifically toward analytics leaders and translators, who need to be treated differently. For leaders, the focus is on analytical thinking, data-driven management, understanding the analytics strategy, and serving as a role model. For translators, the focus is on their bridging role and how to tackle analytical challenges independently while driving a collaborative approach.
Training Formats for Analytics Leaders
Leaders need a starting point for their journey, with frequent follow-up delivered in formats that help them grow in their roles. That starting point is an initial engagement workshop or camp to get everyone on the same page. We recommend holding this workshop after the analysis strategy has been finalized.
These programs are time-consuming and expensive. They last for one or two days, and taking executives away from their work for that amount of time is a big deal. Once you find the right place and time, invest heavily in creating value-added content in a coherent format that doesn’t waste their time. This workshop doesn’t directly contribute to company ROI—it’s an investment in awareness—so it has to be clear to leaders that they will get something out of their time commitment.
Combine your content with fresh and exciting elements, and create an environment that gives leaders time to share and exchange ideas, build relationships, and network. We recommend no more than 20 people per workshop, ideally a truly diverse mix of leaders from different domains. Depending on the size of the organization, you may need to hold several events.
Initial workshop
The initial one- to two-day workshop (or “camp”) will be where you make a first impression on all of the organization’s leaders, so be aware that first impressions last. This is your chance to establish a common language, to make them aware of the change and the opportunities it will bring, and to introduce the skills and roles required. Your goal is to enlist the support and commitment of senior management and to gain a better understanding of how to shape further change.
It’s essential to give the workshop a memorable sequence. Here’s the structure we recommend:
- Step 1: Explain the purpose
-
Start with a detailed explanation of the purpose of and strategic approach to the transformation. Lay out the status quo, the company’s insight-driven ambitions, and your roadmap.
- Step 2: Demystify the buzzwords
-
Explain data analytics and other key terms and concepts, such as data science, descriptive and predictive analytics, artificial intelligence, and generative AI. Help everyone to understand data analytics at the highest level and show how analytics fits into strategic decision making.
-
Give concrete examples that are memorable enough for everyone to follow. For example, use a case that perfectly describes the path of analytics from descriptive to prescriptive, with house prices or the Iris data set or whatever (it’s best to ask your data scientists; they’ll provide lots of content).
- Step 3: Give examples
-
Give examples by presenting your first internal use cases or, if you don’t have any yet, using external examples from your industry or from other industries. Explain them and, if possible, demonstrate them live.
- Step 4: Introduce the use-case approach
-
Next, explain analytics-driven management. In particular, focus on ideation and use-case implementation. Show how your use-case pipeline will work and what your end-to-end approach is. In Chapter 5, you will find an example of a possible implementation of a use-case approach.
- Step 5: Brainstorm
-
Have participants brainstorm their own use-case ideas. Divide them into groups and guide them through a design-thinking process, which supports the audience with a well-structured approach: start with a well-defined problem statement of the case, then discuss the business value and required data, and draw a possible future solution. Let them present their ideas and challenge one another. You will find that this is a win-win situation for everyone involved as it encourages analytical thinking and can generate good use cases for your backlog.
- Step 6: Put their ideas through the use-case process
-
Next, show the leaders how their ideas fit into the use-case process as a starting point. Point out how ideas are evaluated for feasibility and business value and considered for possible pilot projects. You may even be able to demonstrate how a prototype is created. Pick a small, less complex case and show live how ML can provide initial insights. You can use no-code tools to do this, which are great for demonstrating a workflow and giving executives a sense of feasibility when they realize that not everything needs to be coded.
- Step 7: Introduce roles and responsibilities
-
Each manager and leader needs to know what their role is and what their responsibilities will be in the transformation as well as the roles and responsibilities of the people they’ll be managing. They need to understand the changes the transformation will bring and what the new roles are. Help them understand how to integrate the analytics translator role within their teams. They also need to get an initial idea of how to approach the integration of analytics for strategic decision making.
- Step 8: Establish the status quo
-
To gauge the transformation, you need a clear sense of the organization’s current maturity. Prepare a survey to ask participants how they view the use and relevance of analytics and how they perceive their own readiness, the readiness of their teams, and the readiness of the organization as a whole. These insights will help you determine further focus areas for the transformation and give leaders a sense of ownership.
- Step 9: Get buy-in
-
During the month following the workshop, get buy-in from participants. Ask each person what they want to do to fulfill their role as an analytics leader and what they need from the CoE to be successful.
The ideas you’ll get during this workshop will be pure gold—use them to guide your upcoming actions. So think of this “leadership camp” as a great opportunity for all participants, both as leaders and for the development of the CoE.
The initial camp is your first appearance in all leaders’ perception; always be aware that first impressions last. It is the chance to establish a common language, to make them aware of the opportunities and the change as well as the skills and roles required for the transformation. You can gain the support and commitment of your senior management and better understand how to shape further change.
Follow-up programs
The initial workshop is just the beginning. You’re creating a long-term leadership engagement program around analytics transformation, and that’s not a one-time thing. Your approach should be educational, experiential, and culturally integrated. Table 4-3 details how we suggest structuring such a program.
Event | Frequency | Purpose | Description |
---|---|---|---|
Executive roundtables | Every two months | Ongoing conversation about their experiences with analytics | Regularly scheduled sessions cover rotating content on specific analytics topics, such as new technologies or use cases within or outside the industry, and encourage leaders to share their challenges and best practices. This ongoing exchange ensures that empowerment is not a one-time event but an integral part of leaders’ professional routines. |
Quarterly skills enhancement practical workshops | Every four months | Strengthening the role and consolidating soft skills | Quarterly workshops help managers deepen their roles and responsibilities in the transformation. The focus is less on the technical aspects of the transformation and more on consolidating soft skills, with practice and repetition. |
Analytics ThinkLabs | Every six months | Collaborative problem solving | ThinkLabs bring leaders together to apply their analytical skills to real business challenges with cross-functional teamwork, a problem-solving mindset, and outside-the-box thinking. External speakers inspire innovation and provide fresh perspectives on specific topics—for example, ideation for gen AI use cases or design thinking for integrating certain algorithms into dedicated workflows. These events also help keep the backlog of use cases current. |
Leadership summit | Once a year | Reflecting on and celebrating progress | This annual summit is the culmination of the engagement program and provides a forum to reflect on the year’s progress in transforming analytics. It is also an opportunity to celebrate successes and recognize the contributions of individuals and teams. This event plays a critical role in maintaining momentum and setting the agenda for the year ahead. |
In addition, fully leverage and consolidate other supporting activities. Consider adding mentoring from analytics experts to your internal leadership development program to personalize the learning experience and provide direct support. Give leaders access to online learning resources so that they can enhance their skills on their own.
As a more formal reinforcement mechanism, dashboards can help leaders track their personal contribution to the transformation by monitoring KPIs. You might also consider community networks, but with caution. Platforms such as Microsoft Teams can overwhelm managers, who sometimes perceive their content as undesirable. It’s better to stick to regular structured formats to avoid information overload.
Training Formats for Analytics Translators
Developing a long-term program for analytic translators requires an approach that evolves with their unique role as the bridge between technical analytics teams and business stakeholders.
Initial workshop
Begin with an intensive workshop, “Kick-off Workshop: Introduction to the Translator Role,” that sets the framework for the analytics translator’s journey. Its content should include the overarching goals of the analytics transformation, the importance of their role as translators, and how to align technical capabilities with business strategies. Address the individuals’ expectations, what their goals are, and how they want to develop. This workshop is essential for familiarizing participants with their roles and providing them with the basic knowledge they need to excel.
Follow-up programs
Table 4-4 provides an example of programming that can serve as follow-up to this workshop.
Event | Frequency | Purpose | Description |
---|---|---|---|
Deep-dive sessions | Every month | Honing technical and business acumen | On a monthly basis, analytics translators should attend sessions that provide a deep dive into specific analytics tools, methodologies, and use cases. This ongoing series ensures that translators are fluent in both the language of data and the language of business. Each session should end with actionable takeaways that translators can apply directly to ongoing projects. |
Analytics ThinkLabs | Every four months | Facilitating cross-disciplinary collaboration | Analytics translators should also take part in the ThinkLabs described in Table 4-3. Translators can ensure that teams within the lab maintain a balance between technical feasibility and business viability. They facilitate communication among data scientists, engineers, and business leaders. As champions of the analytics and business perspective, translators can encourage the adoption of insights derived from data to inform the ideation and prototyping processes within the lab. They can lead the discussion in ideation sessions and guide the development of concepts with their experience from other use cases. |
Analytics leadership summit | Once a year | Reflecting on and celebrating progress | Translators should also participate in the annual leadership summit, for presenting use cases and success stories that showcase how analytics has driven business results, providing evidence of the value of data-driven decision making. They can advise and participate in active presentations, giving insight into how data and analytics can help shape the strategic direction of the organization. The summits provide a platform for translators to build relationships with senior executives, raising the visibility and importance of analytics on the executive agenda. As the voice of technical interoperability, translators can articulate what is technologically possible. |
To complement these key events, we recommend the following supporting activities:
- Active involvement in use-case implementation
-
One of the most important levers for translators to fulfill their role is their active involvement in the use-case implementation process. Not only are they responsible for communicating the progress of the implementation, but they should also become more and more involved in active development. Feedback loops and peer coding sessions with the analysts will help improve translators’ analytical skills and enable them to implement more of their own ideas in the future, serving the federated approach.
- Mentoring and shadowing programs
-
Analytics translators are matched with leaders from other business areas and experienced analytics professionals to foster a deeper interdisciplinary understanding and provide insight into different business areas and analytical processes. Translators spend time with their mentors in their day-to-day work environment, which allows them to gain practical experience and identify potential parallels in the area of analytical solutions. This direct collaboration creates valuable synergies, helping translators solidify and expand their role as facilitators between the worlds of data and business strategy.
- Ongoing certification courses
-
Continuous learning and professional development courses offer certificates in the latest analytics methodologies, emerging technologies, and advanced lateral leadership skills. These courses are designed to deepen translators’ skills and equip them with the latest trends and tools for effective data analysis and interpretation.
- Project presentations
-
Regular project presentations let analytics translators demonstrate how to apply analytics to gain tangible business results. Translators explain their ideas, visualize their successes, share best practices, and learn new approaches. These events are critical to recognizing translators within the organization and building their confidence.
- Feedback loops
-
By establishing structured mechanisms to give translators constructive feedback from stakeholders, feedback loops are essential for continuous improvement. They provide valuable insight into the effectiveness of translators’ work and allow them to fine-tune their methods and approaches. Regular feedback ensures that translators can grow in their role and continuously adapt to the changing needs of the organization.
Data Literacy Training
Good content alone is not enough to ensure success. Even when participants recognize the need for training, time is always a limiting factor. Analytics translators might do well with a dedicated, long-term training program that is part of their agreed-upon role and can thus be built into their schedules. For managers’ schedules, however, a long course is unlikely to be suitable. Some employees may also lack the motivation and endurance to complete long courses, which is why a motivational approach is important. For example, not everyone is able to organize their own training.
Companies have begun to recognize this problem. StackFuel, for example, offers an upskilling program that combines organized and self-paced training within a certain time frame. This provides structure and helps participants track their overall progress and data literacy. This approach ensures that everyone is on the same page and no one is rushing ahead. So determine the right formats for leaders and translators, then combine them with events and programs designed to engage the community of analytics translators.
Technical Training
We’ve discussed data literacy for the business roles, but it’s also essential to have a proper technical development program for the analytics professionals. It’s hard to make any generic recommendations here: the training they need will depend a lot on the company’s technical orientation and analytics ecosystem. For instance, if the company depends heavily on vendor solutions, people will need specific training on those solutions.
What we can recommend is to focus on general analytics skills, such as basic statistics, data processing, and open source programming languages. SQL, Python, and R are crucial foundations for coding skills.
In addition to new skills, professionals need to improve their technical and methodological skills continuously. In our experience, analytics professionals usually know their needs best and often want to use specific sources to improve their knowledge. Specialized providers such as DataCamp, Udacity, Udemy, and LinkedIn Learning offer a wide range of online training. Look for engaging and motivating resources. Analytics professionals certainly don’t need a fully guided approach, but rewards and a structured approach will help them be more successful. In our opinion, DataCamp has current content and a strong motivational approach.
In addition, there are a lot of other concepts that can be helpful to retrain people to become data professionals. Reading books is always a good approach. You might also consider attending special programs like Springboard or StackFuel Reskills.
Conclusion
The critical takeaway from this chapter is the importance of the four key analytical roles to the business. Each of these roles has expectations; some produce content, and others consume it. The leaders, who are expected to drive the entire transformation by example, and the analytics translators, who bridge the two worlds of business and analytics, have particularly important functions. The transformation manager actively shapes and guides the transformation and actively accompanies the change. Each role requires a certain mindset and skillset, and can be further subdivided into different personas with their own individual functions, depending on the business model.
Without these roles, you will not be able to implement your transformation to the point of achieving stage 3, Data Progressive status. You will also need to create a CoE to kick-start the transformation and to lead and organize all change initiatives. This unit is the one most capable of understanding business needs and implementing analytics solutions.
As your organization matures in its AA transformation, it’s important to shift your approach to applying analytics. Serving increasing demand from a centralized unit will inevitably lead to limitations, so at a certain point, you will need to distribute responsibility for using analytics. We recommend adopting a federalized approach that empowers decentralized units while ensuring coordination and guidance from an accompanying CoE.
In the next chapter, we will discuss how use cases can help move the organization forward in data maturity. You will also understand how AA will be essential to achieving the final stage of data fluency. We will discuss the key aspects of the need for adoption and technical integration, their benefits, and their challenges.
1 For our purposes, “designing a system” means defining the structure of an approach to an analytics transformation. See Melvin Conway, “How Do Committees Invent?”, Datamation, April 1968; and Martin Fowler, “Conway’s Law”, MartinFowler.com, October 20, 2022.
2 Defined by Professor Michael Löwe, 2007 FHDW Hannover, in a direct conversation with his students in preparation for an exam.
Get Augmented Analytics now with the O’Reilly learning platform.
O’Reilly members experience books, live events, courses curated by job role, and more from O’Reilly and nearly 200 top publishers.