What machine learning engineers need to know
The O’Reilly Data Show Podcast: Jesse Anderson and Paco Nathan on organizing data teams and next-generation messaging with Apache Pulsar.
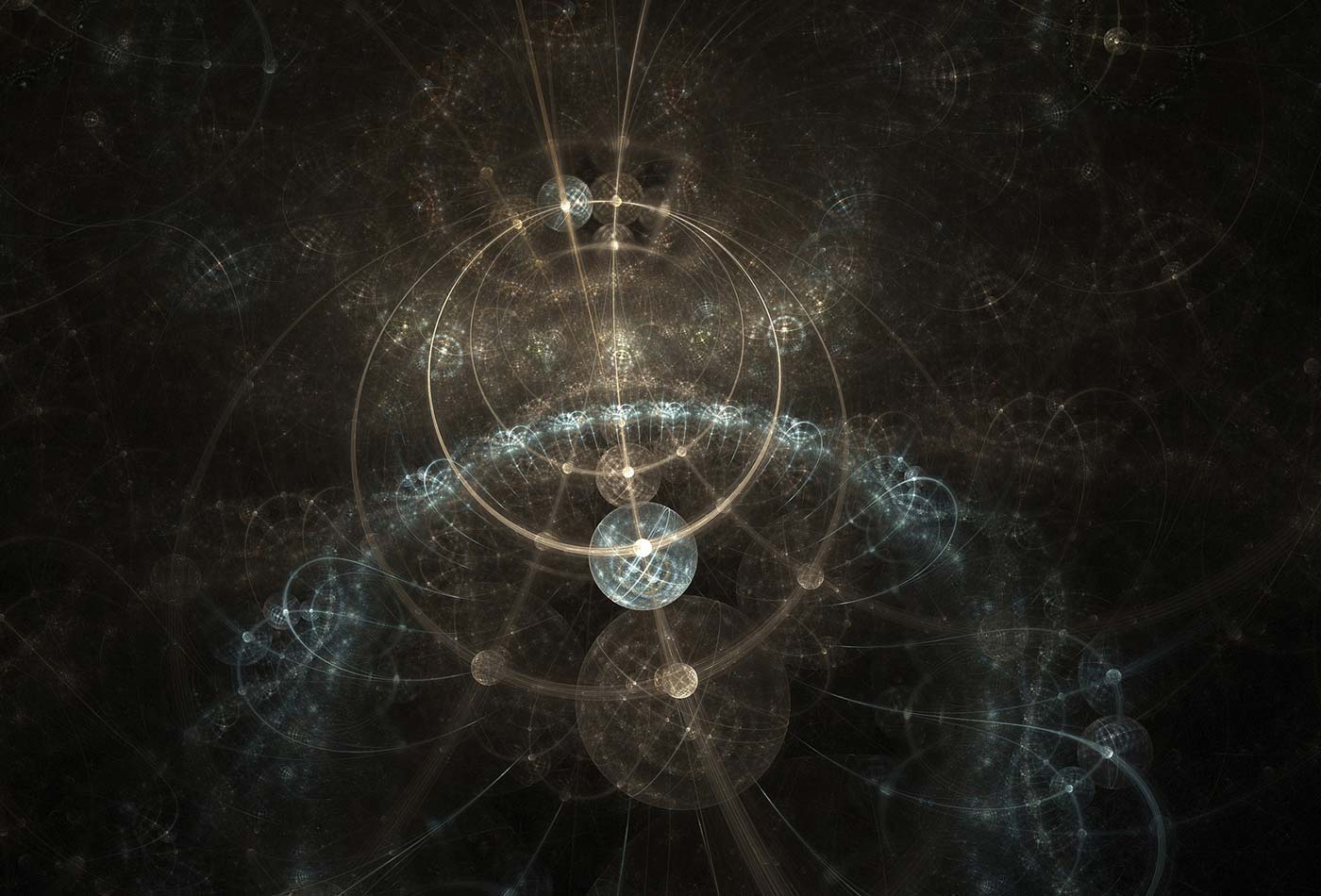
In this episode of the Data Show, I spoke with Jesse Anderson, managing director of the Big Data Institute, and my colleague Paco Nathan, who recently became co-chair of Jupytercon. This conversation grew out of a recent email thread the three of us had on machine learning engineers, a new job role that LinkedIn recently pegged as the fastest growing job in the U.S. In our email discussion, there was some disagreement on whether such a specialized job role/title was needed in the first place. As Eric Colson pointed out in his beautiful keynote at Strata Data San Jose, when done too soon, creating specialized roles can slow down your data team.
We recorded this conversation at Strata San Jose, while Anderson was in the middle of teaching his very popular two-day training course on real-time systems. We closed the conversation with Anderson’s take on Apache Pulsar, a very impressive new messaging system that is starting to gain fans among data engineers.
Here are some highlights from our conversation:
Why we need machine learning engineers
Jesse Anderson: (2:09) One of the issues I’m seeing as I work with teams is that they’re trying to operationalize machine learning models, and the data scientists are not the one to productionize these. They simply don’t have the engineering skills. Conversely, the data engineers don’t have the skills to operationalize this either. So, we’re seeing this kind of gap in between the data science and the data engineering, and the gap I’m seeing and the way I’m seeing it being filled, is through a machine learning engineer.
… I disagree with Paco that generalization is the way to go. I think it’s hyper-specialization, actually. This is coming from my experience having taught a lot of enterprises. At a startup, I would say that super-specialization is probably not going to be as possible, but at an enterprise, you are going to have to have a team that specializes in big data, and that is a part from a team, even a software engineering team, that doesn’t work with data.
Putting Apache Pulsar on the radar of data engineers
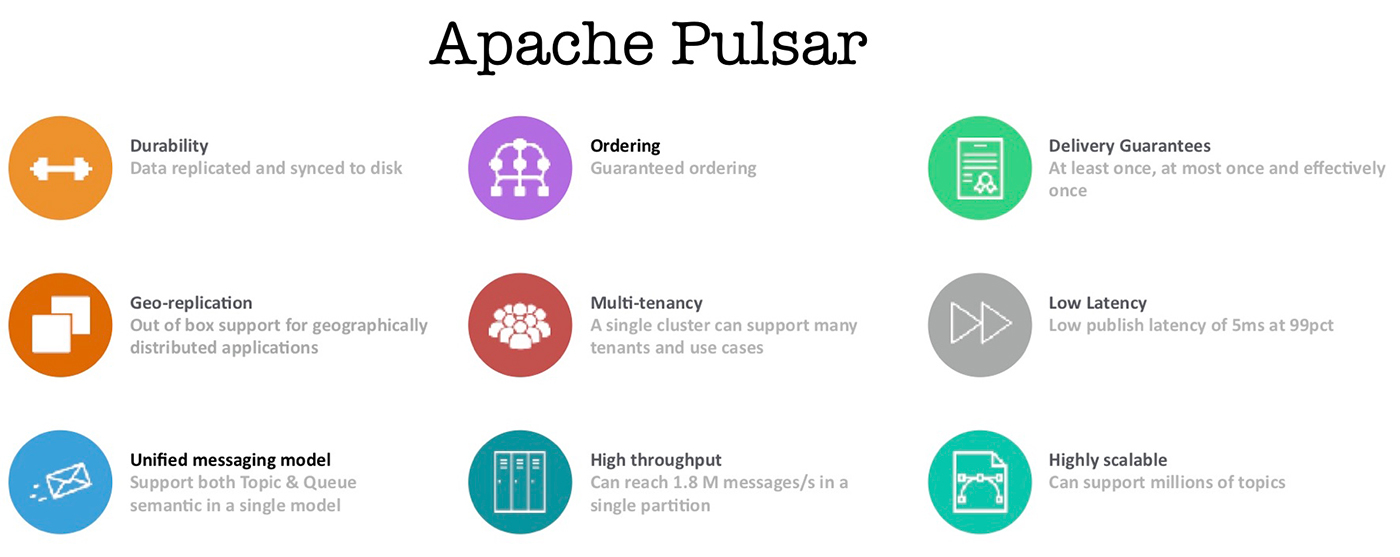
Jesse Anderson: (23:30) A lot of my time, since I’m really teaching data engineering is spent on data integration and data ingestion. How do we move this data around efficiently? For a lot of that time Kafka was really the only open source game in town for that. But now there’s another technology called Apache Pulsar. I’ve spent a decent amount of time actually going through Pulsar and there are some things that I see in it that Kafka will either have difficulty doing or won’t be able to do.
… Apache Pulsar separates pub-sub from storage. When I first read about that, I didn’t quite get it. I didn’t quite see, why is this so important or why this is so interesting. It’s because you can individually scale your pub-sub and your storage resources independently. Now you’ve got something. Now you can say, “Well, we originally decided I wanted to store data for seven days. All right, let’s spin up some more bookkeeper processes and now we can store fourteen days, now we can store twenty one days.” I think that’s going to be a pretty interesting addition there. Where the other side of that, the corollary to that is, “Okay, we’re hitting Black Friday and we don’t have so much more data coming through as we have way more consumption and have way more things hitting our pub-sub. We could spin up more pub-sub with that.” This separation is actually allowing some interesting use cases.
Related resources:
- “What are machine learning engineers?”
- “We need to build machine learning tools to augment machine learning engineers”
- “Differentiating via data science”: Eric Colson explains why companies must now think very differently about the role and placement of data science in organizations.
- “Architecting and building end-to-end streaming applications”: Karthik Ramasamy on Heron, DistributedLog, and designing real-time applications.
- “How machine learning will accelerate data management systems”: Tim Kraska on why ML will change how we build core algorithms and data