How machine learning impacts information security
The O’Reilly Data Show Podcast: Andrew Burt on the need to modernize data protection tools and strategies.
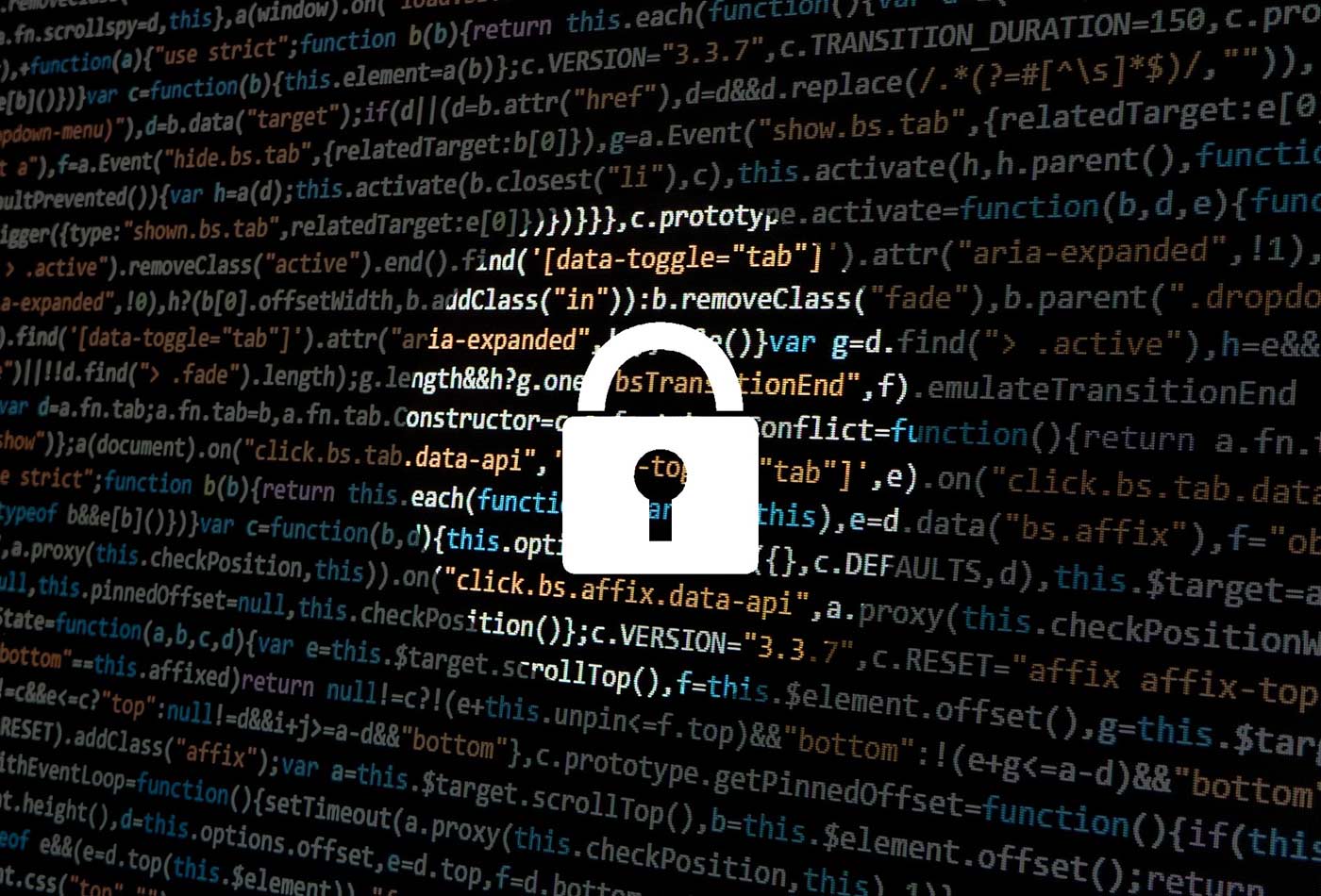
In this episode of the Data Show, I spoke with Andrew Burt, chief privacy officer and legal engineer at Immuta, a company building data management tools tuned for data science. Burt and cybersecurity pioneer Daniel Geer recently released a must-read white paper (“Flat Light”) that provides a great framework for how to think about information security in the age of big data and AI. They list important changes to the information landscape and offer suggestions on how to alleviate some of the new risks introduced by the rise of machine learning and AI.
We discussed their new white paper, cybersecurity (Burt was previously a special advisor at the FBI), and an exciting new Strata Data tutorial that Burt will be co-teaching in March.
Privacy and security are converging
The end goal of privacy and the end goal of security are now totally summed up by this idea: how do we control data in an environment where that control is harder and harder to achieve, and in an environment that is harder and harder to understand?
… As we see machine learning become more prominent, what’s going to be really fascinating is that, traditionally, both privacy and security are really related to different types of access. One was adversarial access in the case of security; the other is the party you’re giving the data to accessing it in a way that aligns with your expectations—that would be a traditional notion of privacy. … What we’re going to start to see is that both fields are going to be more and more worried about unintended entrances.
Data lineage and data provenance
One of the things we say in the paper is that as we move to a world where models and machine learning increasingly take the place of logical instruction-oriented programming, we’re going to have less and less source code, and we’re going to have more and more source data. And as that shift occurs, what then becomes most important is understanding everything we can about where that data came from, who touched it, and if its integrity has in fact been preserved.
In the white paper, we talk about how, when we think about integrity in this world of machine learning and models, it does us a disservice to think about a binary state, which is the traditional way: “either data is correct or it isn’t. Either it’s been tampered with, or it hasn’t been tampered with.” And that was really the measure by which we judged whether failures had occurred. But when we’re thinking not about source code but about source data for models, we need to be moving into more of a probabilistic mode. Because when we’re thinking about data, data in itself is never going to be fully accurate. It’s only going to be representative to some degree of whatever it’s actually trying to represent.
Related resources:
- “Managing risk in machine learning”
- Sharad Goel and Sam Corbett-Davies on “Why it’s hard to design fair machine learning models”
- Alon Kaufman on “Machine learning on encrypted data”
- “Managing risk in machine learning models”: Andrew Burt and Steven Touw on how companies can manage models they cannot fully explain.
- “We need to build machine learning tools to augment machine learning engineers”
- “Case studies in data ethics”