Enabling end-to-end machine learning pipelines in real-world applications
The O’Reilly Data Show Podcast: Nick Pentreath on overcoming challenges in productionizing machine learning models.
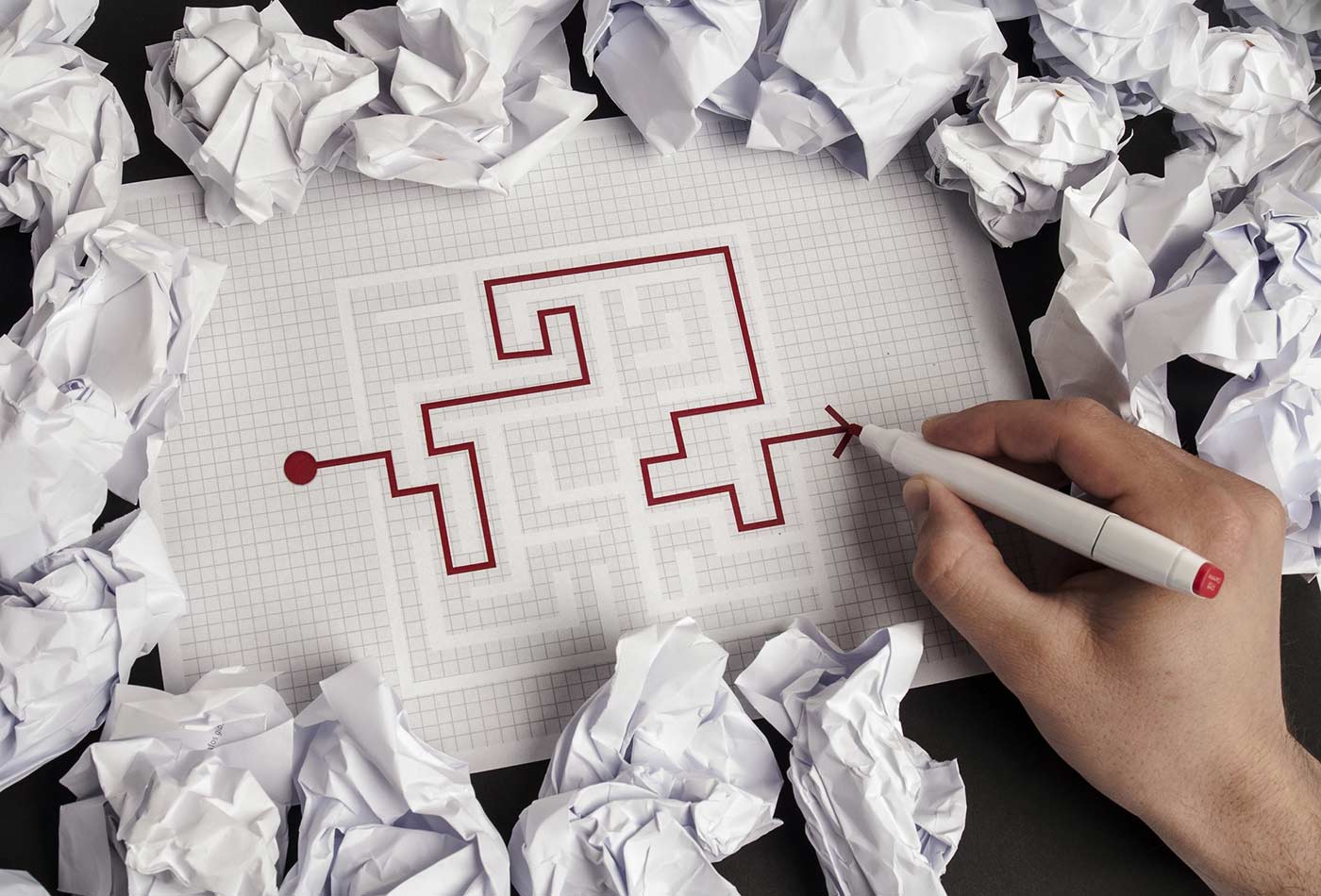
In this episode of the Data Show, I spoke with Nick Pentreath, principal engineer at IBM. Pentreath was an early and avid user of Apache Spark, and he subsequently became a Spark committer and PMC member. Most recently his focus has been on machine learning, particularly deep learning, and he is part of a group within IBM focused on building open source tools that enable end-to-end machine learning pipelines.
We had a great conversation spanning many topics, including:
- AI Fairness 360 (AIF360), a set of fairness metrics for data sets and machine learning models
- Adversarial Robustness Toolbox (ART), a Python library for adversarial attacks and defenses.
- Model Asset eXchange (MAX), a curated and standardized collection of free and open source deep learning models.
- Tools for model development, governance, and operations, including MLflow, Seldon Core, and Fabric for deep learning
- Reinforcement learning in the enterprise, and the emergence of relevant open source tools like Ray.
Related resources:
- “Modern Deep Learning: Tools and Techniques”—a new tutorial at the Artificial Intelligence conference in San Jose
- Harish Doddi on “Simplifying machine learning lifecycle management”
- Sharad Goel and Sam Corbett-Davies on “Why it’s hard to design fair machine learning models”
- “Managing risk in machine learning”: considerations for a world where ML models are becoming mission critical
- “The evolution and expanding utility of Ray”
- “Local Interpretable Model-Agnostic Explanations (LIME): An Introduction”
- Forough Poursabzi Sangdeh on why “It’s time for data scientists to collaborate with researchers in other disciplines”