Data science for humans and data science for machines
The O’Reilly Data Show Podcast: Michael Li on the state of data engineering and data science training programs.
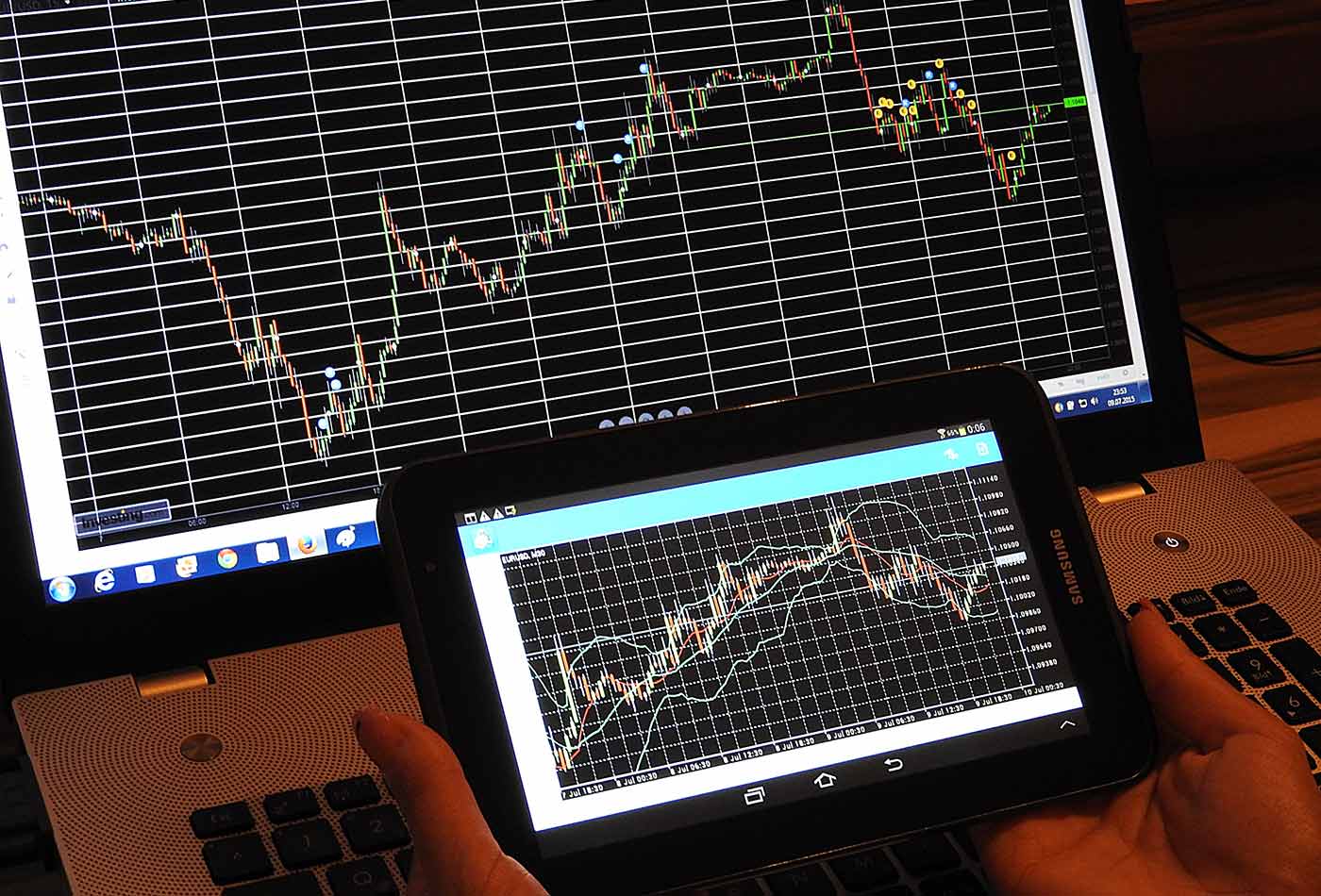
In this episode of the O’Reilly Data Show, I spoke with Michael Li, cofounder and CEO of the Data Incubator. We discussed the current state of data science and data engineering training programs, Apache Spark, quantitative finance, and the misunderstanding around the term “data science.”
Here are some highlights from our conversation:
Wall Street quants and data science
When I think about finance, I often think of it like data science 1.0 or maybe even data science 2.0, and what we call data science now is really more like data science 2.0 or 3.0. It’s the next wave of data science, so it means that when people were practicing data science on Wall Street, they had much more primitive tools in the ‘80s and the early ‘90s than what we’re using now, so they were kind of scraping by. But because they’ve been practicing data science for so much longer, there’s just so much more of a built-up understanding of how this works. …A lot of what I was doing at Foursquare was taking basic things that I learned on Wall Street, applying them toward monetization, and it did pretty well. I think there’s a lot that data science can learn from finance and vice versa.
Data science for humans and data science for machines
There is a distinction between data science for humans versus data science for machines. I think that a lot of people just think, ‘Oh, they’re data scientists. They just look at data,’ but it really depends. The kind of person you’re looking to hire really depends on whether the output of his or her analysis is meant to be given to human decision makers or whether that output is meant to be handed to a machine that will then process everything. I did a little bit of both at Foursquare, but the two approaches required very different skill sets. For one of them, I have a metric, and I need to improve that metric. Let me just turn this dial and make it as complex as possible. For the other one, you have to realize that a human has to understand this, so you have to make this model simple enough that humans can look at it and really wrap their minds around it. I think this distinction is very important.
Apache Spark training
We talk to a lot of hiring companies. We always want to understand what’s interesting to them. Just to give you a few examples, when we started the Data Incubator, I think Spark still wasn’t a very big thing, but now we’re seeing this kind of huge demand for Spark, and that’s one of the things that our corporate training partners are really asking for. It’s one of our most popular modules.
…Last year is about when we started building out the Spark courses, but we’ve really seen that take off in the past year. … It’s been great to see Spark evolve to the point where we’re collaborating with Databricks to do trainings and see this huge demand in industry.
Related resources: