P-values not quite considered harmful
The crisis of reproducibility is an opportunity to get better at doing science.
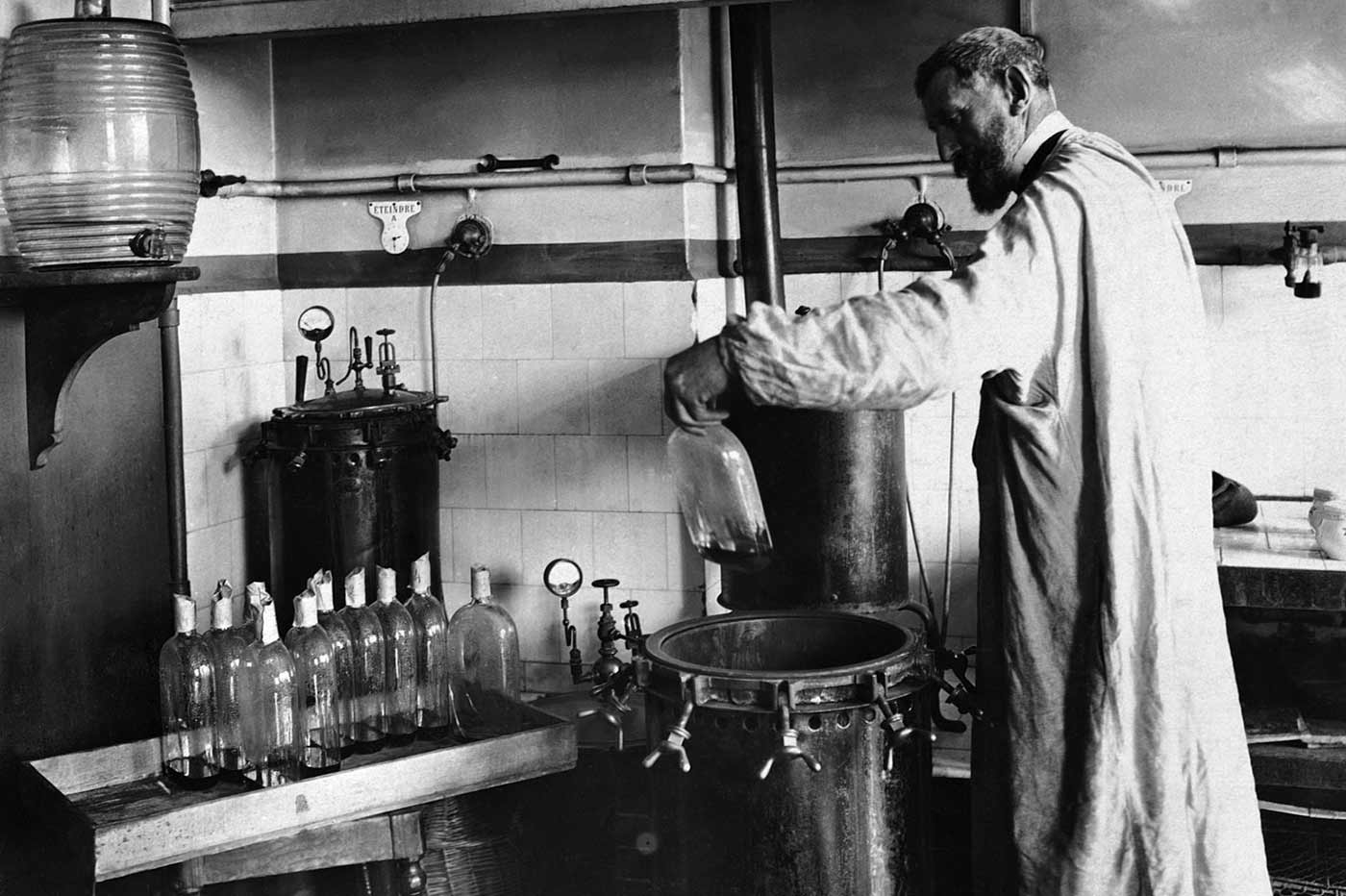
There’s been a lot of writing recently about p-values and the reproducibility of scientific results. P-values are hard to explain, even more difficult to understand, and easy to exploit in the effort to get a publishable result. There have been calls to abandon reliance on p-values, along with spirited defenses.
I’m not sympathetic to p-values, and I’m on board with anyone who wants to put the analysis of experimental results on a firmer basis. However, it’s hard for me to think that p-values are a Really Big Problem. At best, they’re a small part of a larger problem.
Although it’s hard to describe what a p-value is in concrete terms, it’s essentially the probability of observing your experimental result, or an even stronger result, if the “null hypothesis” is correct. The null hypothesis is something like “the result you’re trying to prove is false.” So p is something like the probability of seeing your result given random data. (Yes, statisticians are probably screaming now.) There’s a lot of subtlety wrapped into this. A p-value doesn’t tell you the probability that your result is invalid. It doesn’t tell you the probability that your result is due to random chance. It doesn’t tell you whether you’re looking at a strong effect or a weak one. Statistics aside, p certainly doesn’t tell you anything about whether you are interpreting your results correctly. And p doesn’t tell you whether your results can be attributed to experimental errors that you didn’t take into account.
Using p as the primary indicator that a result is “valid” is problematic, to say the least. That isn’t to say that p-values are useless, but with any metric, you can’t start out by pretending the metric says things that it doesn’t. However, there are bigger problems that need addressing, problems that have little to do with p-values:
- First, we don’t really understand how to describe experiments precisely. In any experiment, there’s a lot of local knowledge, lab folklore, stuff that “everyone knows” (but a lot of people don’t really know).
- Second, there’s a lot of possibly relevant data that we don’t collect: calibration data, environmental data, and so on. For example, does it matter whether test tubes are made of plastic or glass? (Yes, it can make a big difference.) Could the weather make a difference in an experimental result? Probably not, but possibly: I can imagine ambient temperature and humidity affecting the behavior of some piece of equipment.
- Third, a lot of biology is about learning good lab technique. But even among people with good technique, one person’s technique isn’t the same as someone else’s. Although it’s possible to analyze person-to-person variation statistically, it is very difficult, if not impossible, to describe in a protocol. You can’t say “stir it just the way I stir it.”
- Fourth, we’re not very good at publishing data. We’re particularly poor at publishing the data from the intermediate steps of an experiment. Such data often fails the “who would possibly want that” test, though it might be precisely what’s necessary to figure out why an experimental result can’t be duplicated: at what step is something going wrong? Even when we publish the data, we don’t have very good tools for discovering other data, comparing data sets from different experiments, or even discovering other relevant experiments.
- Fifth, we’re not good at designing experiments that are statistically effective, or that have outcomes that can easily be re-used by others.
None of these problems means that experimental results are invalid, even when they can’t be reproduced. But they do mean that experimental results are harder to reproduce and interpret than we’d like to think. What’s the hidden knowledge, the quirk of a researcher’s technique, the poorly described intermediate result that would help us to understand? We don’t know. We have trouble talking about those problems; we don’t have much language for talking about the ways in which attempts to perform the same experiment differ. But I’m willing to bet that those differences are a lot more important than whether p is 0.10, 0.05, or 0.01. P has become a scapegoat for bigger problems in experimental science. Arguing about p won’t help if the real issue is that we don’t know how to describe experiments, how to collect data, or how to publish results in ways that can be used effectively by others.
Here’s a radical proposal. Let’s consider the crisis of reproducibility as an opportunity to think about the scientific enterprise itself. In 2015, scientific research leads (ideally) to a journal article, which in turn is a ticket in the tenure lottery. If an important result can’t be verified, that’s bad: bad for the researcher, bad for the journal, bad for the funding agency. To see something different, let’s go back to the beginning of scientific publishing: the Royal Society’s Philosophical Transactions, volume 1 (1665-1666), the first scientific journal published in English. In the Epistle Dedicatory, Henry Oldenburg, the editor, writes that the purpose of the journal is “To spread abroad Encouragements, Inquiries, Directions, and Patterns, that may animate, and draw on Universal Assistances.” The scientific community is a society for mutual assistance in the discovery and propagation of knowledge. The first article, An Accompt of the Improvement of Optick Glasses, includes a confirmation of Christiaan Huygen’s observation that Saturn’s rings are a disk surrounding the planet. That is followed by a report from Robert Hooke about observing a “small Spot” (probably not the Great Red Spot) on the planet Jupiter. Next is The Motion of the late Comet Praedicted. This piece is a request “either to confirm the Hypothesis, upon which the Author had beforehand calculated the way of this Star, or to undeceive him, if he be in a mistake.” The next few issues contain a several responses and arguments to this article. All in all, the Transactions aren’t presentations of results so much as requests for verification, or confirmations of previous observations. They are parts of a much larger conversation between enthusiasts.
Seeing publications as explicit requests for verification changes the “crisis of reproducibility” completely. It’s normal to present a result that can’t be verified; that’s just part of the discussion. If a result can’t be verified, we can either reject the result, or we can figure out how to verify it. (A later article in the Transactions presents a very dubious process for killing rattlesnakes; I doubt this was ever verified.) We won’t verify results by statistical sleight-of-hand, but by better descriptions of experimental methods, better data collection, and standardized experimental techniques. To facilitate this, we’ll need to get better at publishing and sharing all the data and protocols that led up to the result, not just the key pieces. And that will require rethinking what “publication” means, how candidates are evaluated for research positions and tenure, and how experiments are funded.
That’s the challenge that reproducibility presents. The crisis isn’t really a crisis at all; it’s an opportunity to get better at doing science.