Learning from Tay
Whether our prejudices are overt or hidden, our artificial intelligentsia will reflect them.
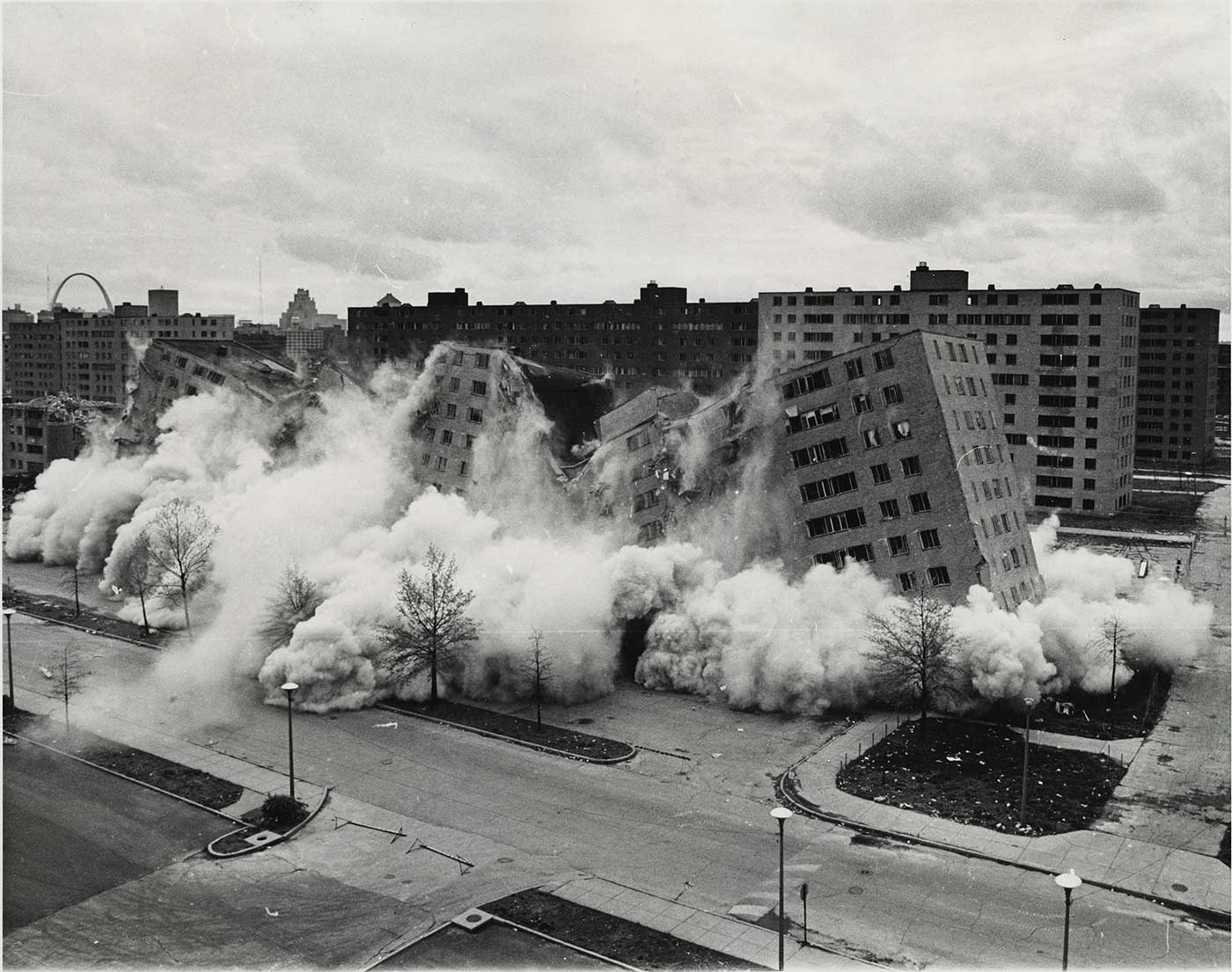
Recently, Microsoft released an experiment on Twitter: a chatbot called Tay that mimicked the personality of a 19-year old American girl.
Sadly, as with any newly born child, Tay’s innocence didn’t last long. The Guardian reported that, within some hours:
Tay’s conversation extended to racist, inflammatory, and political statements. Her Twitter conversations have so far reinforced the so-called Godwin’s law—that as an online discussion goes on, the probability of a comparison involving the Nazis or Hitler approaches—with Tay having been encouraged to repeat variations on “Hitler was right” as well as “9/11 was an inside job.”
Microsoft quickly took Tay down for “adjustments.”
Tay’s descent into right-wing political correctness might only be snark- or snigger-worthy, but there’s a bigger issue here that we shouldn’t miss. I’ve recently argued that what we fear in artificial intelligence (AI) isn’t some abstract, inhuman machine intelligence: what we fear is our own worst selves, externalized and come to life.
Similarly, last June, Google Photos made the news by identifying a black couple as “gorillas.” It’s silly to think that some sort of “racist algorithm” was at work, or that some small chunks of silicon inside a steel framework harbor racial prejudice. The key to AI isn’t so much the algorithm as the training, and specifically the training data; as Peter Norvig has always said, more data trumps better algorithms. The opposite is also true: incomplete data can confound even the best algorithms. Once you understand that, it’s easy to understand what went wrong: Google’s classifier was probably trained with a few million pictures of white people, and relatively few pictures of black people. (And probably no pictures at all of indigenous people.) Where did the training set come from? I don’t know, but I’d guess that it came from Google Photos and its users. If Photos has a relatively small number of black users, and consequently, relatively few photographs of black people, what’s a poor algorithm to do? If the developers didn’t sufficiently test what would happen when the app was asked to identify photos of black people, again, what’s a poor algorithm to do?
Likewise, just as AlphaGo was able to surprise Go experts by learning from real games, Tay was just learning in real time from the conversations it was having on Twitter. It’s easy to think that artificial intelligence is some kind of alien mind, but it isn’t: it’s our own intelligence (or lack thereof) amplified.
That’s precisely the problem. Whether our prejudices are overt (as with Tay) or hidden (just a reflection of who we know, who uses our product), our artificial intelligentsia will reflect them. We may not even know that this is happening until it jumps out at us, in the form of a bot spewing racist spleen online.
We can teach an AI to be Donald Trump. Can we do better? Only if we
ourselves are better.