Part III. Transform
The second part of the book was a deep dive into data visualization. In this part of the book, you’ll learn about the most important types of variables that you’ll encounter inside a data frame and learn the tools you can use to work with them.
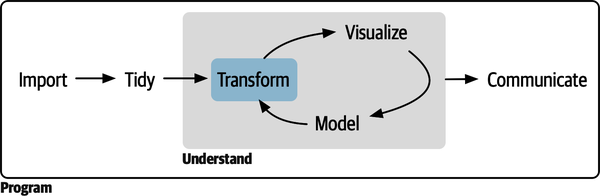
Figure III-1. The options for data transformation depend heavily on the type of data involved, the subject of this part of the book.
You can read these chapters as you need them; they’re designed to be largely standalone so that they can be read out of order.
Chapter 12 teaches you about logical vectors. These are the simplest types of vectors, but they are extremely powerful. You’ll learn how to create them with numeric comparisons, how to combine them with Boolean algebra, how to use them in summaries, and how to use them for condition transformations.
Chapter 13 dives into tools for vectors of numbers, the powerhouse of data science. You’ll learn more about counting and a bunch of important transformation and summary functions.
Chapter 14 gives you the tools to work with strings: you’ll slice them, you’ll dice them, and you’ll stick them back together again. This chapter mostly focuses on the stringr package, but you’ll also learn some more tidyr functions devoted to extracting data from character strings.
Chapter 15 introduces you to regular expressions, a powerful tool for manipulating strings. This chapter will ...
Get R for Data Science, 2nd Edition now with the O’Reilly learning platform.
O’Reilly members experience books, live events, courses curated by job role, and more from O’Reilly and nearly 200 top publishers.