GANs allow new applications to produce new samples from a previous set of samples, including completing missing information.
In the following screenshot, we depict a number of samples created with the LSGAN architecture on five scene datasets from LSUN, including a kitchen, church, dining room, and conference room:
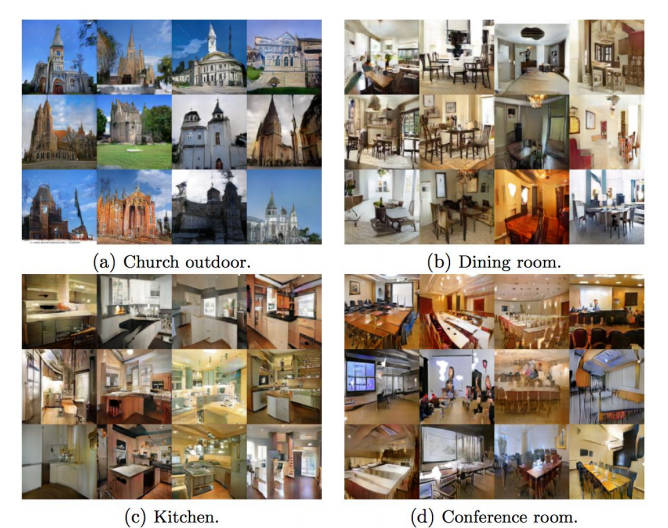
Another really interesting example is class-conditional image sampling using the Plug and Play Generative Network (PPGN) to fill in 100 x 100 missing pixels in a real 227 x 227 image.
The following screenshot compares PPGN variations and the equivalent Photoshop image completion: