In the next experiment, we evaluate the effectiveness of the NS optimization method in a more complex task. In this task, we try to evolve a maze solving agent that can find a path through a maze with a complex configuration.
For this experiment, we use the hard-to-solve maze configuration introduced in the previous chapter. Such an approach allows us to compare results obtained with the NS optimization method against the results obtained with the goal-oriented optimization method used in the previous chapter. The maze configuration is as follows:
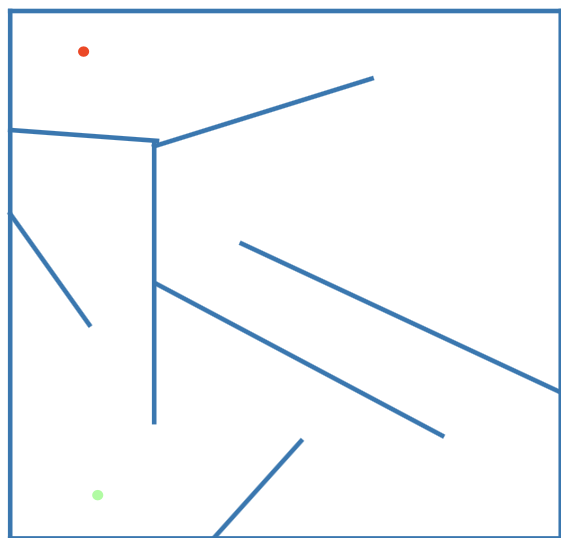