The curse of dimensionality is a common problem where the number of necessary data points is exponentially increased when the dimension is increased. Let's say we have two datasets: one in a one-dimensional space and one in a three-dimensional space. If we want to achieve sufficient accuracy with 10 data points in the one-dimensional space, then we need to collect around 1,000 bits of data. The following diagram illustrates a situation where the curse of dimensionality can happen:
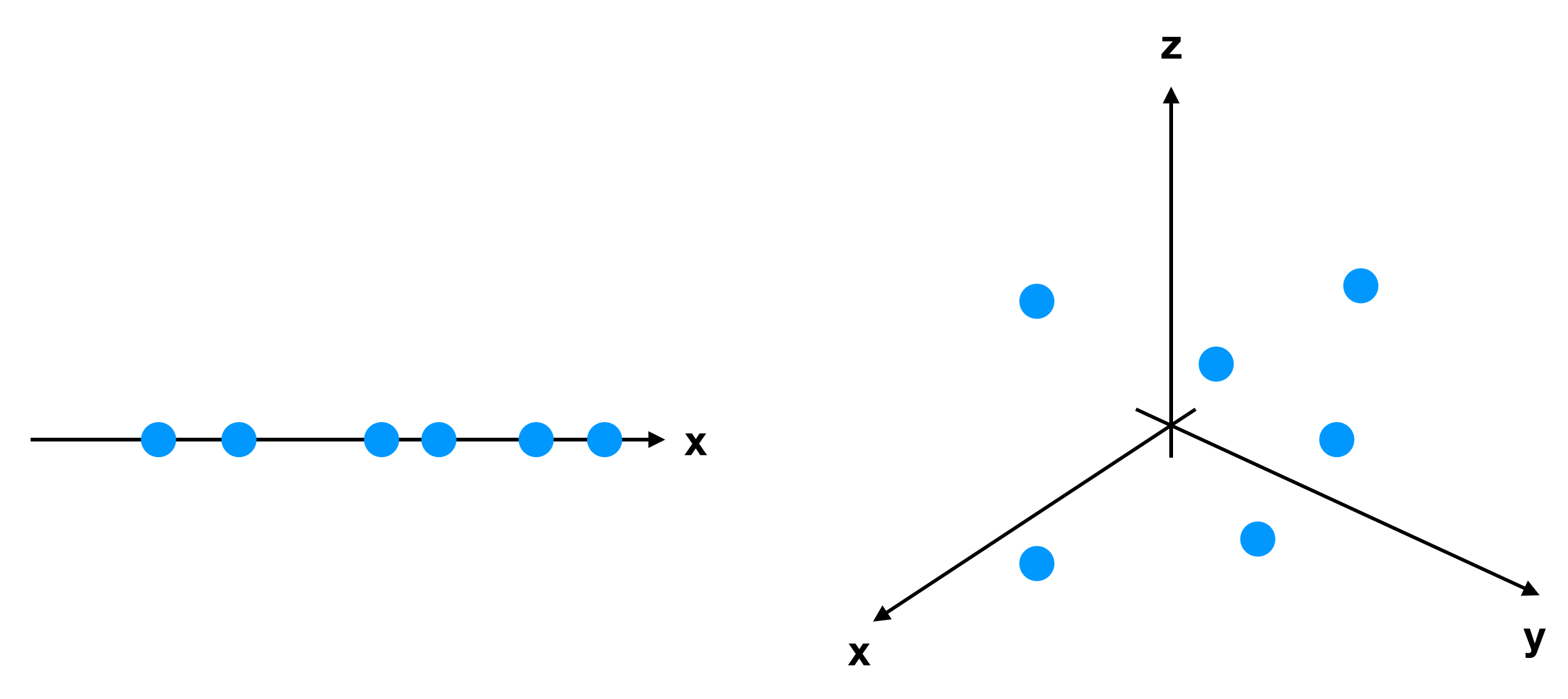
In the left low-dimensional space, the data points cover the one-dimensional space pretty well. However, the same number of data points show ...