It can also be helpful to have a steeper gradient during training; as such, we can use the tanh function instead of the Sigmoid function, as shown in the following code:
func tanh(x){ return 2 * (1 + Exp(-2*x)) - 1}
We get the following output:
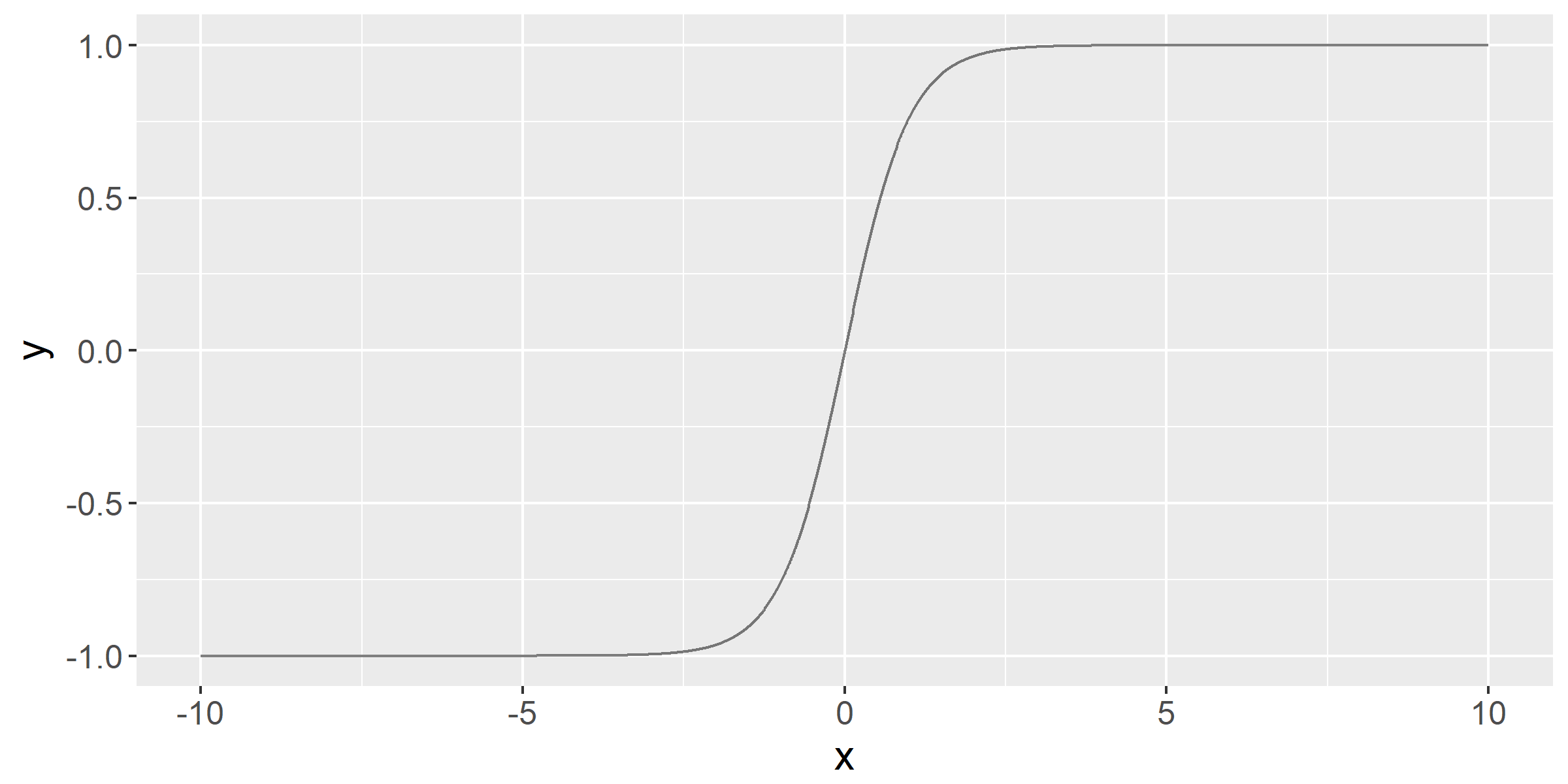
The tanh function has another useful property: its slope is much steeper than the Sigmoid function; this helps networks with tanh activation functions to descend the gradient faster when adjusting weights. The output for both functions is plotted in the following output:
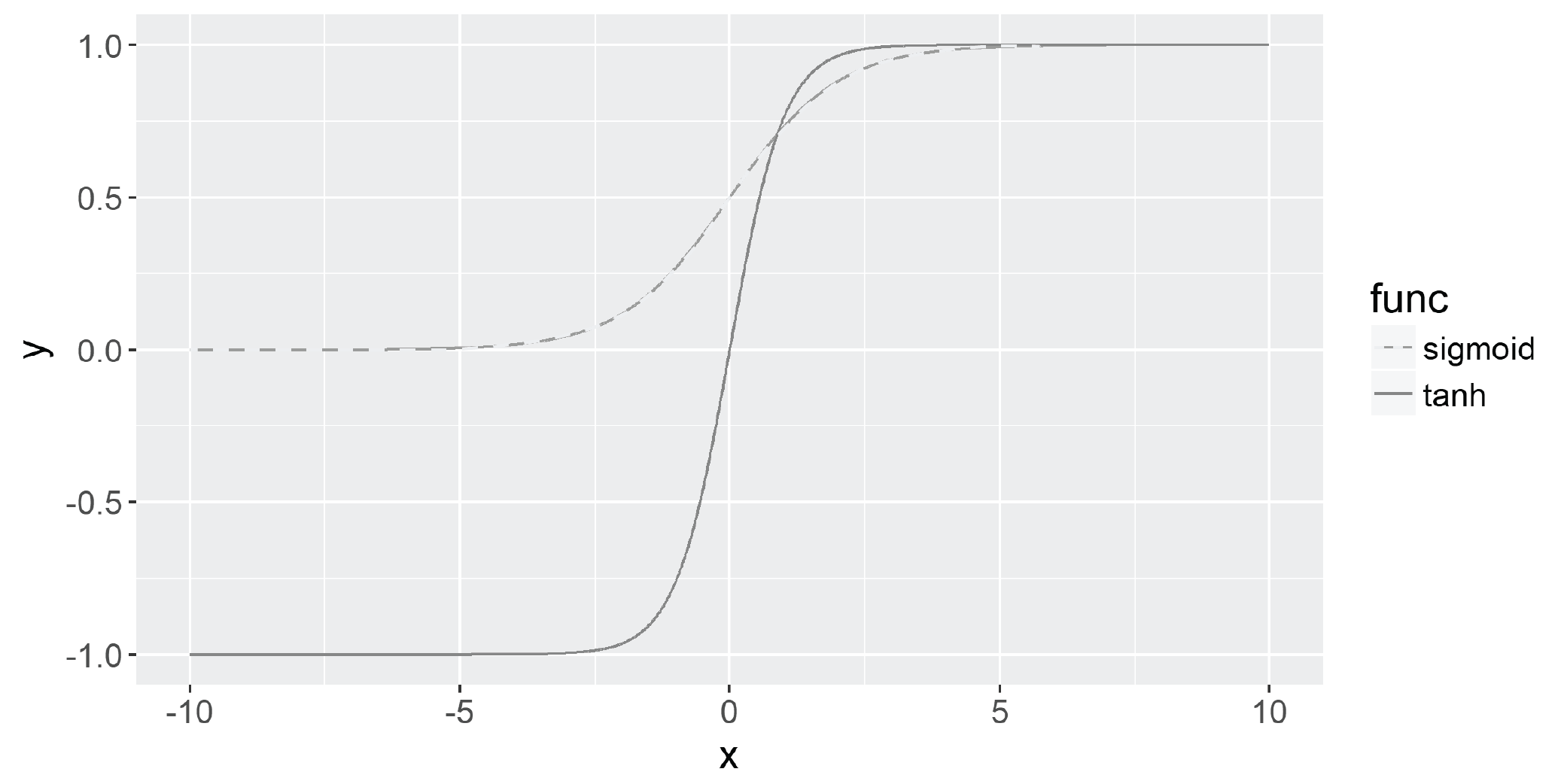