Chapter 7. Interacting with Explainable AI
Explanations cannot exist in a vacuum. They are consumed, used, and acted upon by ourselves, our colleagues, auditors, and the public to gain an understanding of why an AI acted the way it did. Without explainability (and interpretability), Machine Learning (ML) is a one-way street of information and predictions. We may see an ML do something astounding, such as translating a paragraph from one language to another, but it is rare for us to unequivocally trust technology.
Fundamentally, we are in a working relationship with every AI we use. Imagine machine learning as your coworker. Even if this coworker did an amazing job, we would find them difficult to work with if, when we asked them to perform a task, they went off to another room, returned with the answer, and then promptly left again, never answering our questions or responding to a thing we said! This silent coworker problem is what explainability tries to address by starting a two-way dialogue, as in Figure 7-1, between the ML system and its users. However, this dialogue is very limited given how novel explainability is, which makes your choices around how to construct that dialogue even more important.
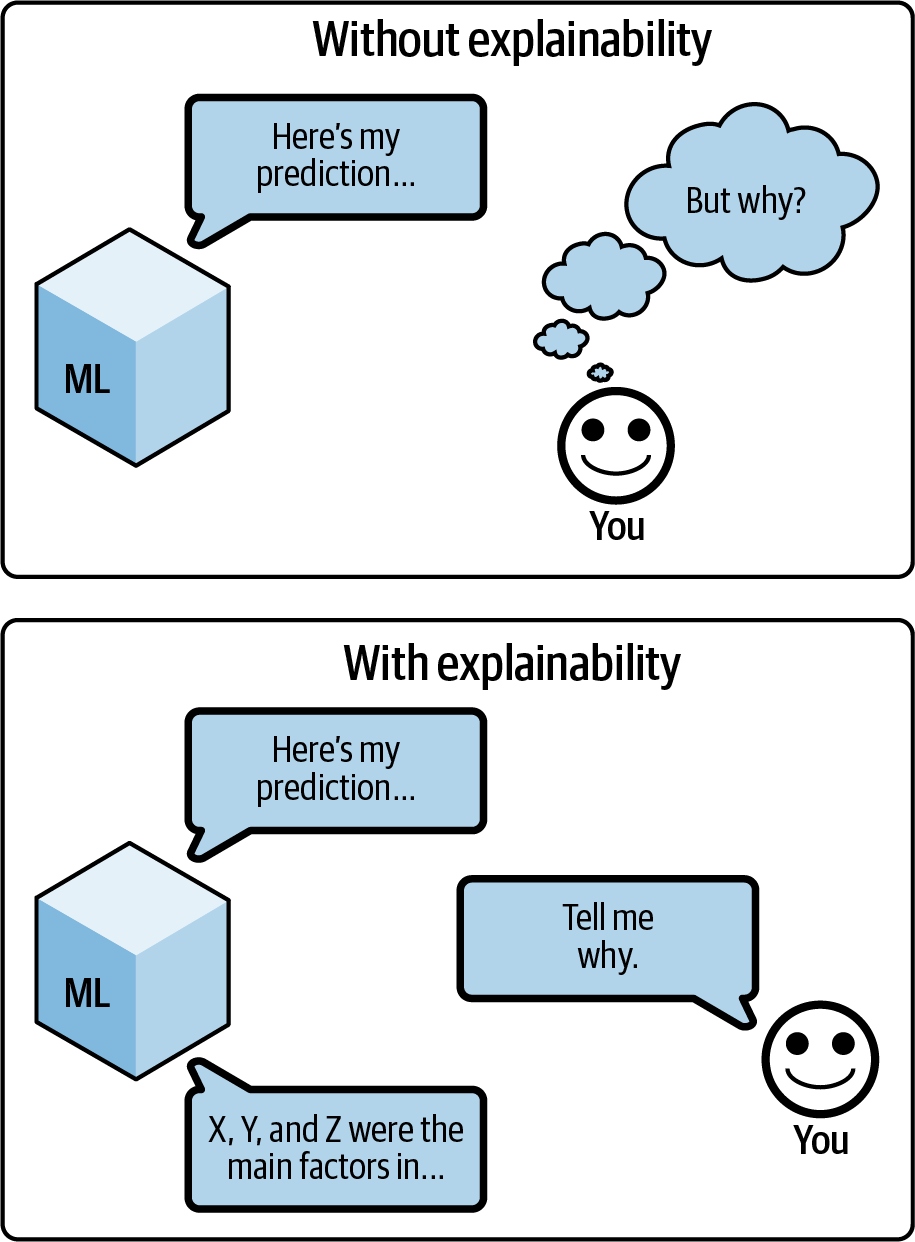
Figure 7-1. Explainability creates a dialogue between an ML and its users.
In this chapter, we will review the needs of different ML consumers and what to keep in mind when designing ...
Get Explainable AI for Practitioners now with the O’Reilly learning platform.
O’Reilly members experience books, live events, courses curated by job role, and more from O’Reilly and nearly 200 top publishers.