Chapter 1. The Business Transformation
As we write this book in 2024, the business landscape is marked by increasing adoption of digital technologies and practices, often referred to as “business transformation” or “industrial transformation.” This transformation is not the first one. So far, the world has seen three industrial transformations, each driven by new technology: steam power (beginning in roughly the 1760s), electricity and mass production (from the mid-1800s to the 1910s), and computerization (from the late 20th century to the present). This chapter will explain what’s different about the current transformation compared to previous ones and why we believe that augmented analytics (AA) is so well positioned to solve the problems that many businesses currently face.
Augmented analytics simply means providing people with access to technology that gives them the analytical leverage they need to accomplish the business task at hand in a better way. If you’ve heard this term before, you might associate it with automated forecasting in Tableau dashboards; that’s not what we’re doing here. AA requires a much more holistic approach to really take off. We will dive deeper into defining AA in Chapter 3.
Before we do that, let’s zoom out a bit and explore the external driving forces that make using AA a necessity for staying competitive in today’s world.
Why Businesses Are Transforming
We’re currently in the computerization and information age, and many experts believe that AI will drive the next phase of this industrial transformation. So what makes this phase of business transformation more special than previous ones? We believe there are four main factors at play, which we’ll examine one by one in this section.
Factor 1: The Speed of Change
Digital transformation is happening much faster than previous transformations, requiring businesses to be agile and adapt quickly. For example, steam power took several decades to fully transform the economy. Mass production took roughly half a century. And computerization took about 20 to 30 years. In contrast, the digital transformation is happening in just a few years for some industries. To be clear, most companies and organizations are just not built for that pace; you need exceptional leadership and organizational agility to succeed in this environment.
For businesses, this rapid pace of change has led to increased pressure to keep up and stay competitive. Every bookstore around the corner suddenly has to compete with companies like Amazon; every taxi company has to compete with Uber, which offers exceptional customer service built on top of world-class data analytics. But the competition doesn’t come only from disruptive players. Even traditional businesses have started to disrupt themselves. Consider the shift toward Industry 4.0 in manufacturing, which includes adopting smart factories and Internet of Things (IoT) devices to optimize production processes and improve efficiency.
Factor 2: The Convergence of Multiple Technologies
Previous industrial transformations were driven largely by one or two key technologies, such as steam and electricity. But digital transformation involves integrating technologies like AI, cloud computing, data analytics, and IoT into manufacturing, which on their own have the potential to disrupt entire industries.
The advancements of the 2010s and 2020s in computation, networking, and storage have resulted in new technologies and services that have significantly changed the way businesses operate. This makes the current phase of digital transformation much more complex and multidimensional. It’s extremely hard to predict where the ship is heading and navigate the waters successfully. Disruption can happen practically overnight, as we saw in the consumer electronics and retail spaces, or it can take years. For example, while renewable energy started disrupting the energy sector around 20 years ago, it’s still far from being the dominant energy source in most countries.
Factor 3: The Importance of Data
Digital transformation often requires fundamental changes in business models and in the way organizations think about data. In previous industrial revolutions, data “just happened”: you knew how many cars you sold, how much material you needed, how many employees you had, and so on. You analyzed data in hindsight to improve processes. Don’t get us wrong—this alone was a big deal. It has fueled an entire business-intelligence software industry in the golden age of computerization, including database vendors and visualization tools. However, in the digital age, data has evolved from something that happens to something that must happen.
The volume of data created, captured, copied, and consumed is still growing at an unprecedented pace, according to a 2022 McKinsey report. As Figure 1-1 shows, this trend has been taking place for more than a decade and is predicted to keep up its momentum.
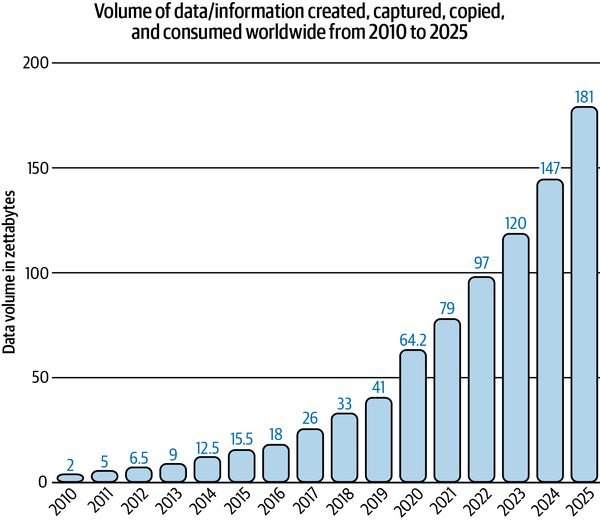
Figure 1-1. The growing amount of data worldwide (source: Statista)
Most organizations have found a way to tackle the sheer volume of data with modern technology: cheap storage, high-performance compute, and low-latency networking power have enabled a new wave of offerings that both consumers and businesses alike love and embrace, such as streaming services, social media platforms, and ecommerce sites. Adoption of these new services has not only fueled the digital ecosystem but has also literally generated the fuel to drive it: data.
Yet most of this data is not clean, tabular data that can easily be analyzed but rather unstructured data, such as text, images, and videos. Businesses generate unstructured data all over the place—for instance, through social media, customer interactions, and IoT sensors. In many cases, technology is only helpful to a certain extent; human analytical skills are required to make sense of the data. Most businesses still struggle with this growing complexity.
So companies have to think strategically about which data they need to collect, how they will collect it, and what insights they can gain from it (which, in some industries, can even happen in real time). This requires a whole new paradigm about data and often drives a need to add new roles to a company.
Some people compare data in the current economy to oil in the “old economy” as a driving resource. But we think this is actually a bad comparison. Unlike oil, data doesn’t get used up when it’s consumed. Instead, we get even more data the more we interact with it. Nor is data a scarce resource: it can be generated and collected in numerous ways. The only parallel that holds true is that both oil and data are worth much less when they are not refined. Both resources need a thorough process of cleaning, preparation, and governance before they can be used for commercial purposes. In a way, every company has to learn these skills, even if it has never worked with data before.
Factor 4: Changing Consumer Behavior and Customer Centricity
When people first saw the iPhone, they never wanted any other phone again. “Who needs a stylus?” Steve Jobs famously asked at the iconic 2007 iPhone launch keynote, pointing his finger in the air. Since then, consumers have gotten used to touch screens instead of mechanical keyboards or plastic pens. The iPhone embraced and anticipated customers’ needs, but it would never have been possible without underlying technical innovations like multitouch display, high computing power, and long battery life.
Since then, numerous other industries have had their own “iPhone moments.’’ For instance:
-
Shopify showed small shop owners that running their own ecommerce store doesn’t have to be hard or expensive.
-
Tesla showed car buyers just how well electric vehicles can perform—and how fun and effortless driving can be.
-
Amazon Prime changed the way people shop, making one-day delivery the norm and raising the bar for customer-service expectations.
-
Netflix revolutionized the way we consume video content, introducing binge-watching and personalized recommendations based on viewing history.
These are just a few examples. We aren’t endorsing the underlying brands or services—we just want to show you that digital transformation spans all industries and is often driven by the changing behaviors and expectations of consumers.
Industries Heavily Impacted by Digital Transformation
McKinsey researchers investigated Big Data’s impact on different industries in a 2011 study, which is the source for Figure 1-2. (While technology has changed a lot since then, its importance in these industries has not, and the key challenges have remained surprisingly steady.) Table 1-1 describes some example industries that have been extraordinarily affected by the current business-transformation processes, their key challenges, and how they leverage data and analytics to address these challenges.
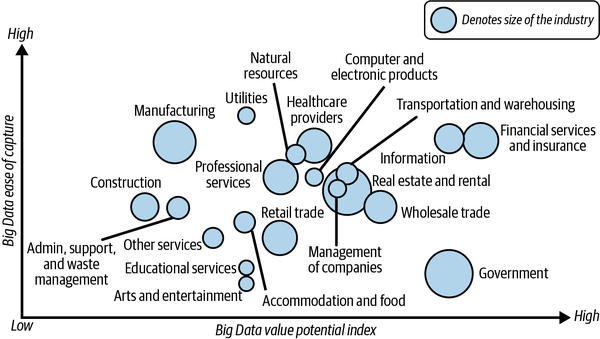
Figure 1-2. Industries heavily impacted by big data and analytics (adapted from McKinsey)
Industry | Why affected | Key challenges | Use case examples |
---|---|---|---|
Agriculture | Meeting growing food demand while addressing sustainability concerns |
|
|
Commercial insurance | Powerful dynamics can be observed on the insurance market. New technologies need to be handled with new technical products and analytics solutions. | Handling industrial Internet of Things (IIoT) demand, B2B processes, data-driven underwriting, and pricing |
|
Finance |
|
|
|
Healthcare |
|
|
|
Information technology | IT plays a critical role in driving digital transformation across all industries. |
|
|
Manufacturing |
|
|
|
Transportation |
|
|
|
Utilities |
|
|
|
The Consequences for Your Business
In the past, transformations primarily centered on optimizing processes and cutting costs. For example, lean manufacturing and Six Sigma, which were all about improving efficiency and reducing waste, were popular trends during the last industrial revolution. These frameworks were mainly driven from an internal perspective: what can we do better and cheaper? No one was asking about the “what” and the “why” of customers’ needs.
Today, however, companies need to be laser-focused on customers and their needs, which involves understanding their journey and creating a seamless experience for them. Why? Because it’s possible. Thanks to digital technology, companies can easily collect large amounts of data about their customers and analyze it to get insights into their behaviors, needs, and preferences. As a result, customer-centricity has become an essential component of staying competitive in today’s market. As we will explain in Chapter 2, analytics is an excellent way to support organizations with managing this transformation—and at the same time is the biggest challenge in itself.
According to a recent Gartner study, fewer than half of data and analytics leaders reported that their team is actually providing value to their organization. The core problems cited by respondents were that analytics has hit a dead end and that making data-driven decisions often turns out to be “too complicated” or “too technical” for the average employee.
Innovation has consistently shown us that the way to spur mass adoption of any technology is by making it simpler and easier to use. Think back to the early days of computing. The very idea of operating a computer was daunting to most ordinary people. Computers were reserved for technically inclined professionals who could navigate through intricate programming commands. Then came the graphical user interface, the desktop, and—perhaps most pivotal of all—the mouse. These seemingly simple inventions transformed computing from a specialist’s arena into everyone’s playground. People who were once intimidated by lines of code could now point, click, and drag. These innovations bridged the massive gap between complex technology and the average user.
Similarly, mobile phones used to be for calling and texting. But the introduction of user-friendly touch screens, intuitive interfaces, and app ecosystems transformed them into ubiquitous, indispensable tools for everything from photography to navigation to banking. These shifts in technology demonstrate a fundamental principle: to achieve widespread adoption, technology needs to be intuitive and easy, and it needs to cater to the user’s natural inclinations and behaviors.
The analytics world is no different. People who use analytics but who are not data professionals form the majority of the workforce: about 80%. These individuals might never be interested in diving deep into complex data sets, sifting through raw data, or understanding intricate analytics tools. Instead of making them come to the world of data, we need to bring data to them.
There’s No Analytics Transformation Without Augmented Analytics
Your authors have a strong opinion: analytics that don’t leverage modern techniques like AI and automation will not suffice for running a profitable business. Why? Because you won’t be able to scale out analytics to the full workforce of the organization.
Consider the three big ideas of Big Data: volume, velocity, and variety. Traditional analytics mainly tackled volume. With modern tech and affordable storage, we can now also handle massive amounts of data quickly and inexpensively, solving velocity. What businesses are really still struggling with is the third V: variety. The challenge of getting insights from complex data is only getting harder.
Imagine a medium-sized company that gathers a tremendous volume of data from numerous sources: internal systems, customer interactions, social media, third-party providers, and more. Each data source has its own structure, format, and level of reliability, and there’s a myriad of data types: structured data such as sales figures, semistructured data like customer feedback, and unstructured data like social media comments.
This complexity is compounded when the company tries to draw insights from its cross-functional data. Say the marketing team wants to understand the impact of a recent promotional campaign on sales. This requires integrating and analyzing data from marketing and sales systems and, potentially, external data, such as market trends and competitor activity, within a certain time frame.
Getting actionable insights here is beyond the capabilities of traditional analytics. You can’t figure all of this out in a manual Excel spreadsheet or a static, overloaded business intelligence (BI) dashboard. The company’s data is a good representative of today’s data: messy, contradictory, and difficult to make sense of.
While technical challenges factor in, the difficulty of making sense of it all is due above all to a lack of analytics maturity. Only a few “data experts” handle these topics—and, in some organizations, the “data experts” are just the best Excel users.
A Data-Driven Culture
Having the best tools and technologies is great, but without the right mindset, they’re of little use. A data-driven culture is an organizational culture in which data isn’t just appreciated—it’s required. It’s a culture where questions like “What does the data say?” are standard. For analytics to truly make an impact, organizations need to foster a mindset that’s curious, analytical, and always hungry for insights.
Most companies still lack this kind of data culture, though. Indeed, most still struggle with basic data literacy, which Gartner defines as the ability to read, interpret, understand, and communicate data effectively. According to a 2020 Qlik study, “Only about 24% of business decision-makers, from junior managers to the C-suite, feel fully confident in their ability to read, work with, analyze and communicate with that data.” That means that most workers do not yet have sufficient skills to analyze and interpret data.
Fortunately, with the right culture and maturity, organizations can tap the full potential of data to guide decisions and strategy.
The “People Problem” and the Limits of Upskilling
During the first three industrial revolutions, employers had the advantage, with a lot of control over their employees and little competition. Taylorism could never have happened in a world where employees could easily find alternative work. Today, though, the leading economies (the United States, Europe, Japan, and so on) have a completely different problem: a “people problem.” They face an aging population and a shrinking workforce. So what does that mean for digital transformation? It’s simple: you can’t just replace your workforce. Even if your business had the economic power and local labor laws allowed it, there wouldn’t be enough human capital available to close the gap.
It’s vital to leverage the workforce you have, including people’s unique skillsets and contributions. But because a lot of these workers don’t have the necessary digital or data-literacy skills, it’s essential for every business to create a culture of learning and upskilling. We’re not talking about a three-day workshop here: getting people ready for the digital age and staying competitive require hard technical skills as well as collaboration, adaptability, and a continuous-learning mindset.
Upskilling the workforce is therefore probably the largest cultural change that every major business will go through in the 2020s and 2030s. Gartner Research predicts that this cultural shift will make the biggest difference in whether digital transformation efforts succeed or fail.
But here’s the hitch: upskilling your workforce probably won’t solve your problem. In fact, we believe that most businesses will never succeed in upskilling all their workers, especially with regard to data analytics. It’s time to accept that, just as not everyone wants to or can be a programmer, not everyone wants to or can be a data person. Instead, we need to empower them with technology, so they can do their jobs better and in a data-driven way. But how is that possible? Some organizations create separate analytics teams, essentially spinning up an internal consulting firm. But this leads to further separating concerns rather than democratizing the company’s data capabilities.
The solution is to meet people where they are. Expecting universal advanced analytics expertise across all roles is unrealistic. Instead, give employees tools and interfaces that augment their existing data skills and capabilities. This pragmatic approach recognizes the limits of mass upskilling while still enabling broader data usage.
AA aims to bring analytics to the people, rather than forcing them to become analysts. Just as the mouse made computers user-friendly, AA aims to make data analytics accessible, intuitive, and (most important) actionable for the majority. This is the transition from a data-driven to an insight-driven approach.
Conclusion
You’ve seen how and why business transformation is happening, and you know that leveraging data and insights at scale is a cornerstone of managing this transformation and staying competitive in global markets.
Scaling analytics across an organization requires more than just upskilling employees and investing in new tools. It requires a paradigm shift in how we approach analytics. Just as the mouse bridged the gap between users and computers, AA can bridge the analytics adoption gap, helping organizations to make better, more informed decisions at scale.
Before we dive deeper into the solution—augmented analytics—let’s make sure you understand the need for analytics and the analytics adoption gap. We’ll see you in the next chapter!
Get Augmented Analytics now with the O’Reilly learning platform.
O’Reilly members experience books, live events, courses curated by job role, and more from O’Reilly and nearly 200 top publishers.