Chapter 9. Fairness in the Age of Algorithms
Anna Jacobson
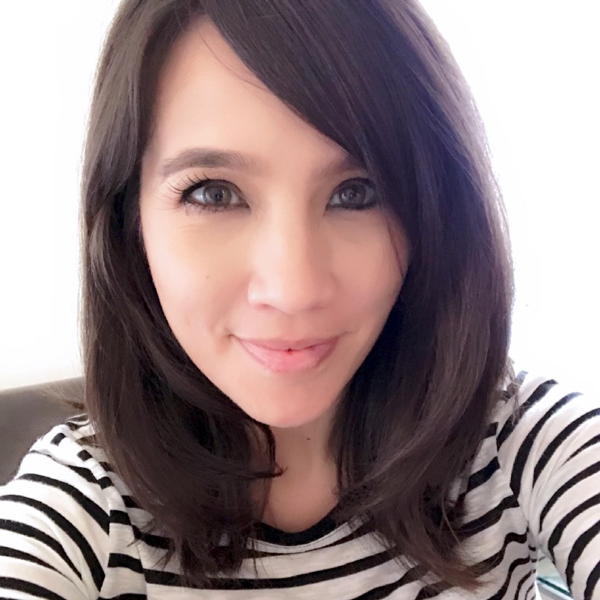
Of all the exciting work taking place in the field of data science, the machine learning algorithm (MLA) is one of the advancements that has garnered the most popular attention—and to many, it is the area of data science that holds the most promise for the future. However, as with all powerful technologies, MLAs also carry the risk of becoming destructive forces in the world.
Early applications of MLAs included email spam filtering, image recognition, and recommender systems for entertainment. In these low-stakes settings, the cost of any errors is low, usually a minor inconvenience at worst. However, the cost of errors in MLAs has dramatically increased as they have begun to be applied to human beings, such as in predictive policing. Despite the apparently objective process of training MLAs, it sometimes results in algorithms that, while computationally correct, produce outputs that are biased and unjust from a human perspective. And in high-stakes settings, MLAs that produce unfair results can do enormous damage.
Fairness is an elusive concept. In the practice of machine learning, the quality of an algorithm is judged based on its accuracy (the percentage of correct results), its precision (the ability not to label as positive a sample that is negative), or its recall ...
Get 97 Things About Ethics Everyone in Data Science Should Know now with the O’Reilly learning platform.
O’Reilly members experience books, live events, courses curated by job role, and more from O’Reilly and nearly 200 top publishers.