Simon Moss on using artificial intelligence to fight financial crimes
Innovations that increase detection of, and response to, criminal attacks of financial systems.
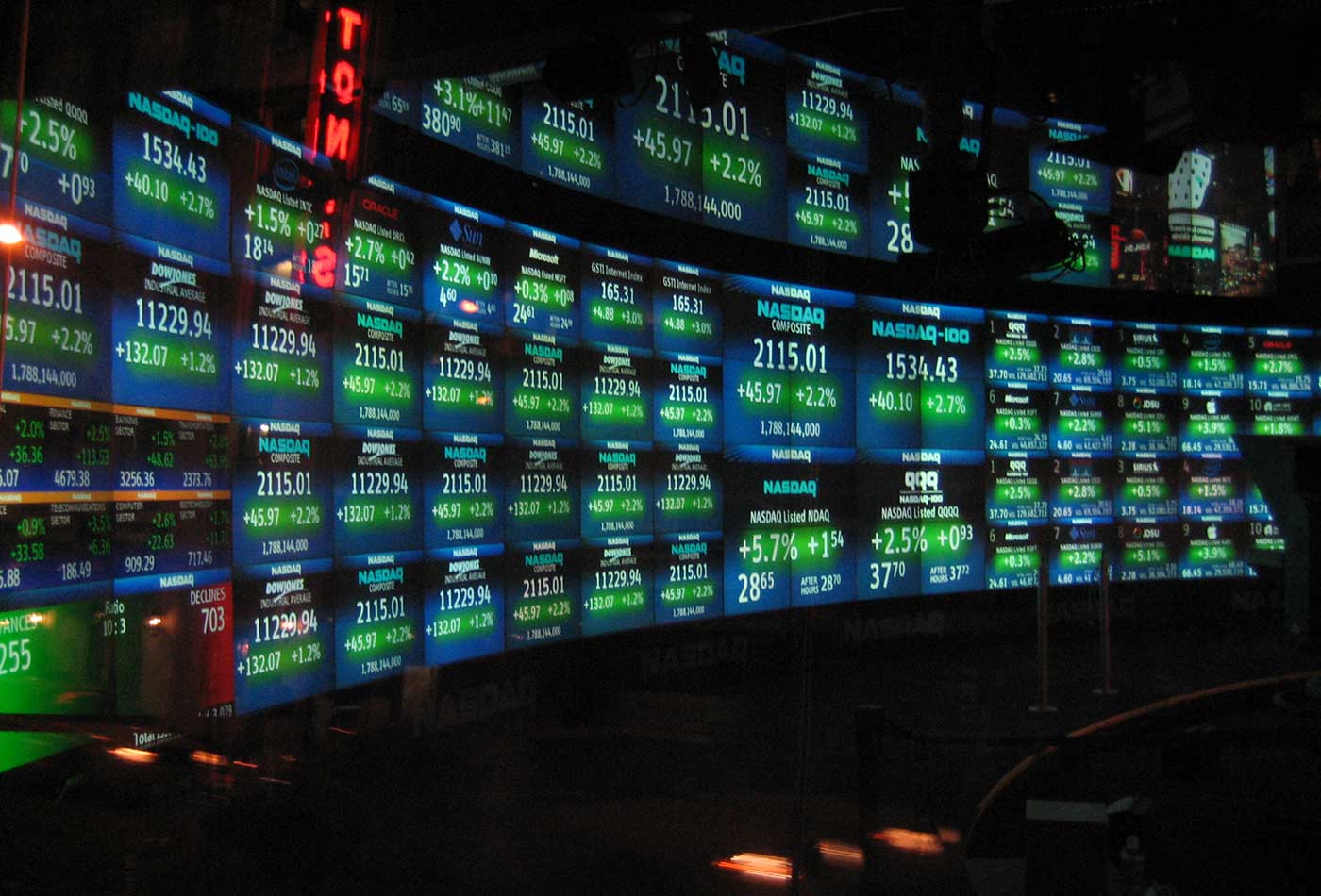
In this episode of the O’Reilly Podcast, I talk with Simon Moss, vice president of industry consulting and solutions, Americas, at Teradata. We discuss how machine learning and deep learning techniques are being used to fight financial crimes, such as credit card fraud, identity theft, health care fraud, and money laundering.
Discussion points:
- Moss says AI techniques are “a new set of weapons” against perpetrators of financial crime. “If we use them right, we can finally at least slow down the constant tide of financial crime.”
- AI can be more effective than traditional methods of combatting identity fraud, health care fraud, and money laundering, because for those issues, he explains, “you’re not looking for a needle in a haystack. You’re looking for a needle in a stack of needles. You are trying to find individuals whose nefarious activity is disguised and hidden in pure normality, by completely innocuous activity.”
- Moss compares the use of machine learning techniques to a rules engine: “a rules engine looks for behaviors that have already happened, whereas machine learning is trying to connect different bread crumbs. It’s running multiple scenarios at the same time to try to look at the problem from multiple different angles.”
- Machine learning can add efficiency to the detection process: “It can take multiple data sources, map the data to a case, analyze it, and then in seconds, make a decision on whether it’s a false positive, whether it’s normal business activity, whether it’s something that needs further investigation, or whether it is outright criminality,” Moss says.
Other links:
- Think Big Analytics, the consulting division of Teradata
- Moss’ recent LinkedIn article on the fight against money laundering
This post is a collaboration between Teradata and O’Reilly. See our statement of editorial independence.