It’s time for data scientists to collaborate with researchers in other disciplines
The O’Reilly Data Show Podcast: Forough Poursabzi Sangdeh on the interdisciplinary nature of interpretable and interactive machine learning.
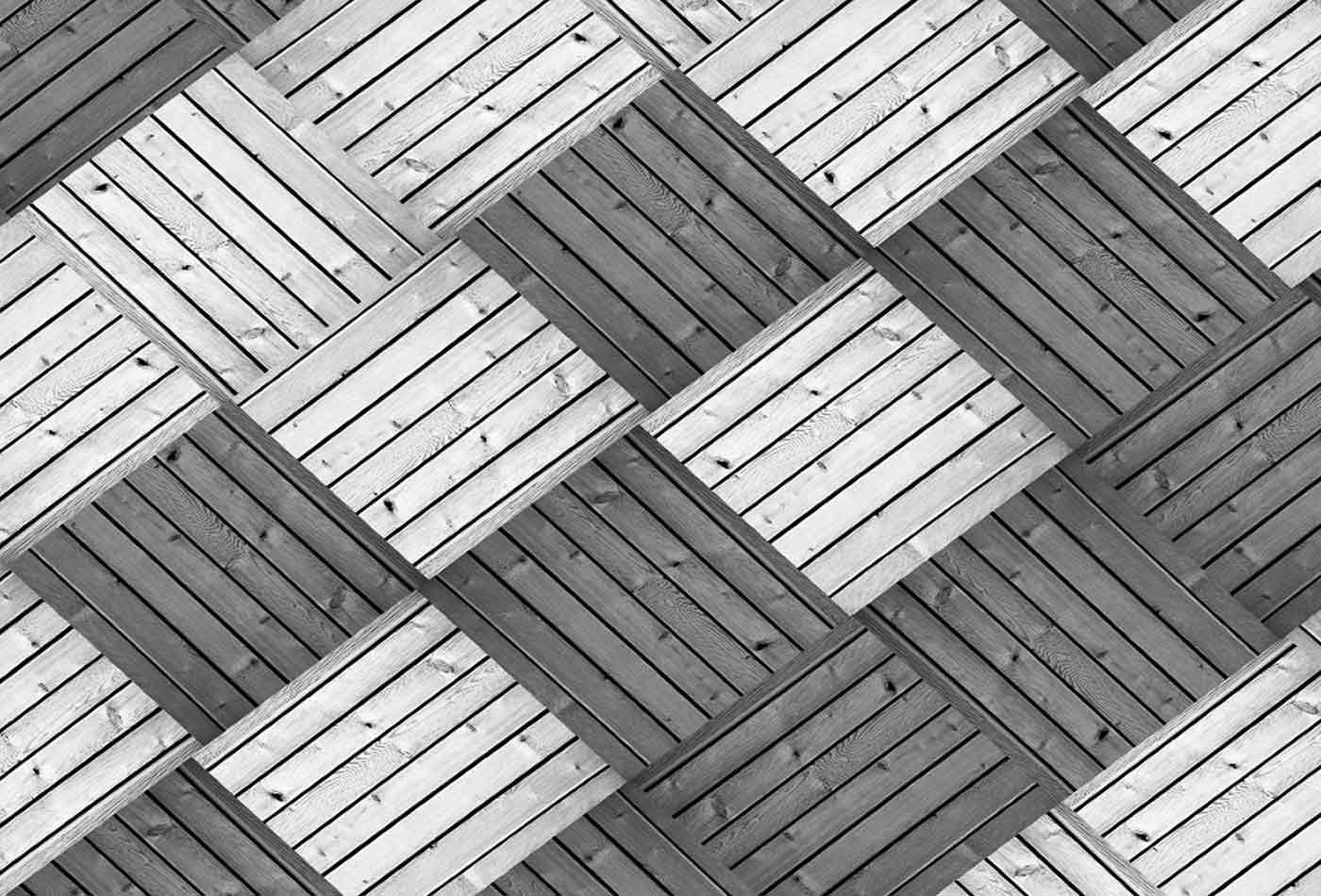
In this episode of the Data Show, I spoke with Forough Poursabzi-Sangdeh, a postdoctoral researcher at Microsoft Research New York City. Poursabzi works in the interdisciplinary area of interpretable and interactive machine learning. As models and algorithms become more widespread, many important considerations are becoming active research areas: fairness and bias, safety and reliability, security and privacy, and Poursabzi’s area of focus—explainability and interpretability.
We had a great conversation spanning many topics, including:
- Current best practices and state-of-the-art methods used to explain or interpret deep learning—or, more generally, machine learning models.
- The limitations of current model interpretability methods.
- The lack of clear/standard metrics for comparing different approaches used for model interpretability
- Many current AI and machine learning applications augment humans, and, thus, Poursabzi believes it’s important for data scientists to work closely with researchers in other disciplines.
- The importance of using human subjects in model interpretability studies.
Related resources:
- “Local Interpretable Model-Agnostic Explanations (LIME): An Introduction”
- “Interpreting predictive models with Skater: Unboxing model opacity”
- Jacob Ward on “How social science research can inform the design of AI systems”
- Sharad Goel and Sam Corbett-Davies on “Why it’s hard to design fair machine learning models”
- “Managing risk in machine learning”: considerations for a world where ML models are becoming mission critical
- Francesca Lazzeri and Jaya Mathew on “Lessons learned while helping enterprises adopt machine learning”
- Jerry Overton on “Teaching and implementing data science and AI in the enterprise”