Bringing AI into the enterprise
The O’Reilly Data Show Podcast: Kris Hammond on business applications of AI technologies and educating future AI specialists.
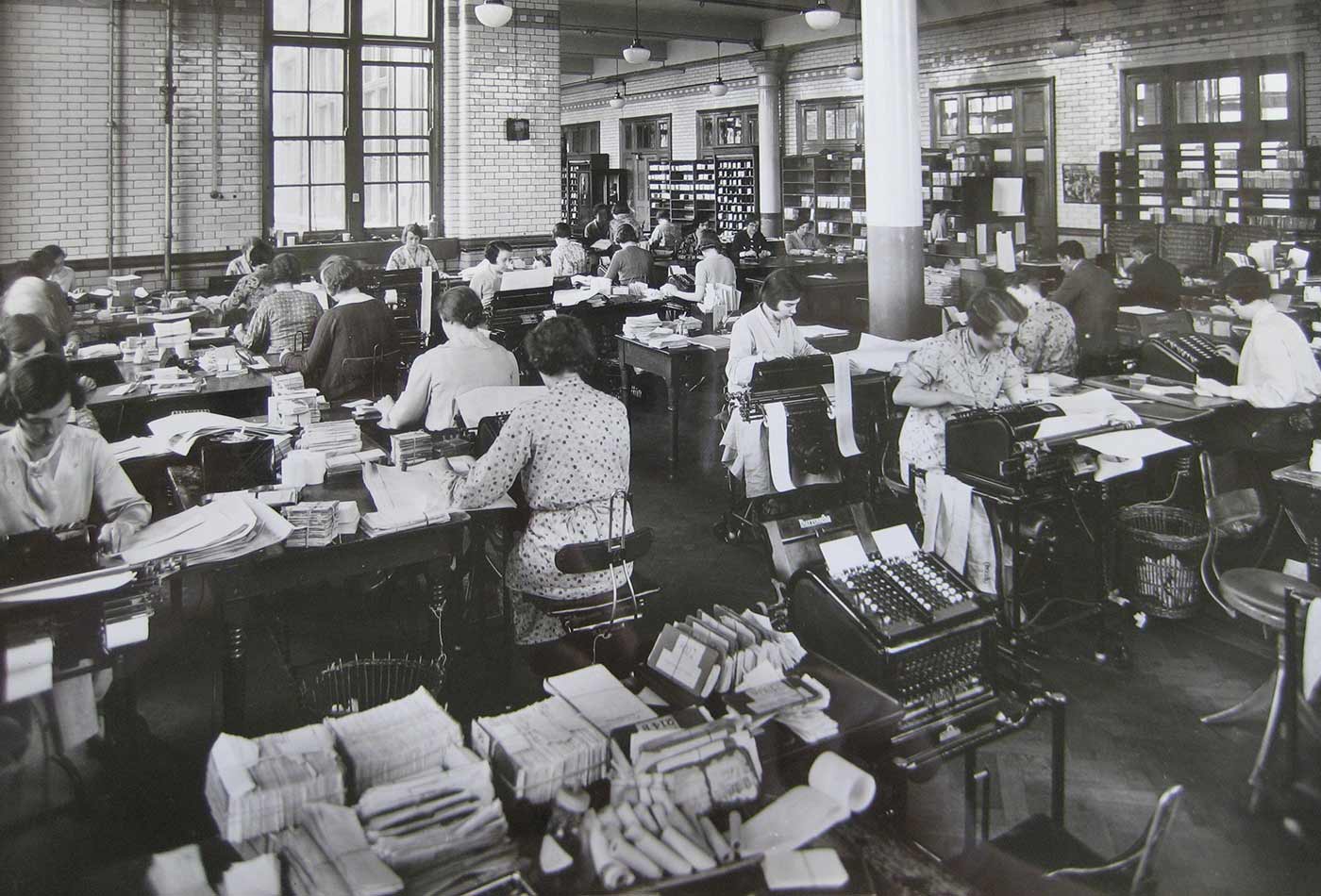
In this episode of the Data Show, I spoke with Kristian Hammond, chief scientist of Narrative Science and professor of EECS at Northwestern University. He has been at the forefront of helping companies understand the power, limitations, and disruptive potential of AI technologies and tools. In a previous post on machine learning, I listed types of uses cases (a taxonomy) for machine learning that could just as well apply to enterprise applications of AI. But how do you identify good use cases to begin with?
A good place to start for most companies is by looking for AI technologies that can help automate routine tasks, particularly low-skill tasks that occupy the time of high-skilled workers. An initial list of candidate tasks can be gathered by applying the following series of simple questions:
- Is the task data-driven?
- Do you have the data to support the automation of the task?
- Do you really need the scale that automation can provide?
We discussed other factors companies should consider when thinking through their AI strategies, education and training programs for AI specialists, and the importance of ethics and fairness in AI and data science.
Here are some highlights from our conversation:
It begins with finding use cases
I’ve been interacting more and more with the companies that are thinking about AI solutions; they often won’t have gotten to the place where they can talk about what they want to do. It’s an odd thing because there’s so much data out there and there’s so much hunger to derive something from that data. The starting point is often bringing an organization back down to, “So what do you want and need to do? What kind of decision-making do you want to support? What kinds of predictions would you like to be able to make?”
Identifying which tasks can be automated
Sometimes, you see a decision being made and, from an organizational point of view, everyone agrees that this decision is really strongly data driven. But it’s not strongly data driven. It’s data driven based upon the historical information that two or three people are using. It looks like they’re looking at data and then making a decision, but, in fact, what they’re doing is, they’re looking at data and they’re remembering one of 2,000 past examples in their heads and coming out with a decision.
… There are sets of tasks in almost any organization that nobody likes to have anything to do with. In the legal profession, there are tasks around things like discovery where you actually need to be able to look through a corpus of documents, but you need to have also some idea of the semantic relationships between words. This is totally learnable using existing technologies.
… It’s not as though tasks that can be automated don’t exist. They do, and, in fact, they not only exist, but they’re easily doable with current technologies. It’s a matter of understanding where to draw the line. It’s sometimes easy for organizations to look at the problem and sort of hallucinate that there is not a different kind of reasoning going on in the heads of the people who are solving the problem.
… You have to be willing to look at that and say, “Oh, I’m not going to replace the smartest person in the company, but, you know, I will free up the time of some of our smartest people by taking these tasks on and having the machine do them.”
Related resources:
- Here and now – Bringing AI into the enterprise: Kris Hammond’s tutorial at the 2017 AI conference in San Francisco.
- Vertical AI – Solving full stack industry problems using subject-matter expertise, unique data, and AI to deliver a product’s core value proposition: Bradford Cross at the 2017 AI conference in San Francisco.
- Demystifying the AI hype: Kathryn Hume at the 2017 AI conference in NYC.
- “6 practical guidelines for implementing conversational AI“: Susan Etlinger on how organizations can create more fluid interactions between humans and machines.
- “How companies can navigate the age of machine learning“: to become a “machine learning company,” you need tools and processes to overcome challenges in data, engineering, and models.