A new benchmark suite for machine learning
MLPerf is a new set of benchmarks compiled by a growing list of industry and academic contributors.
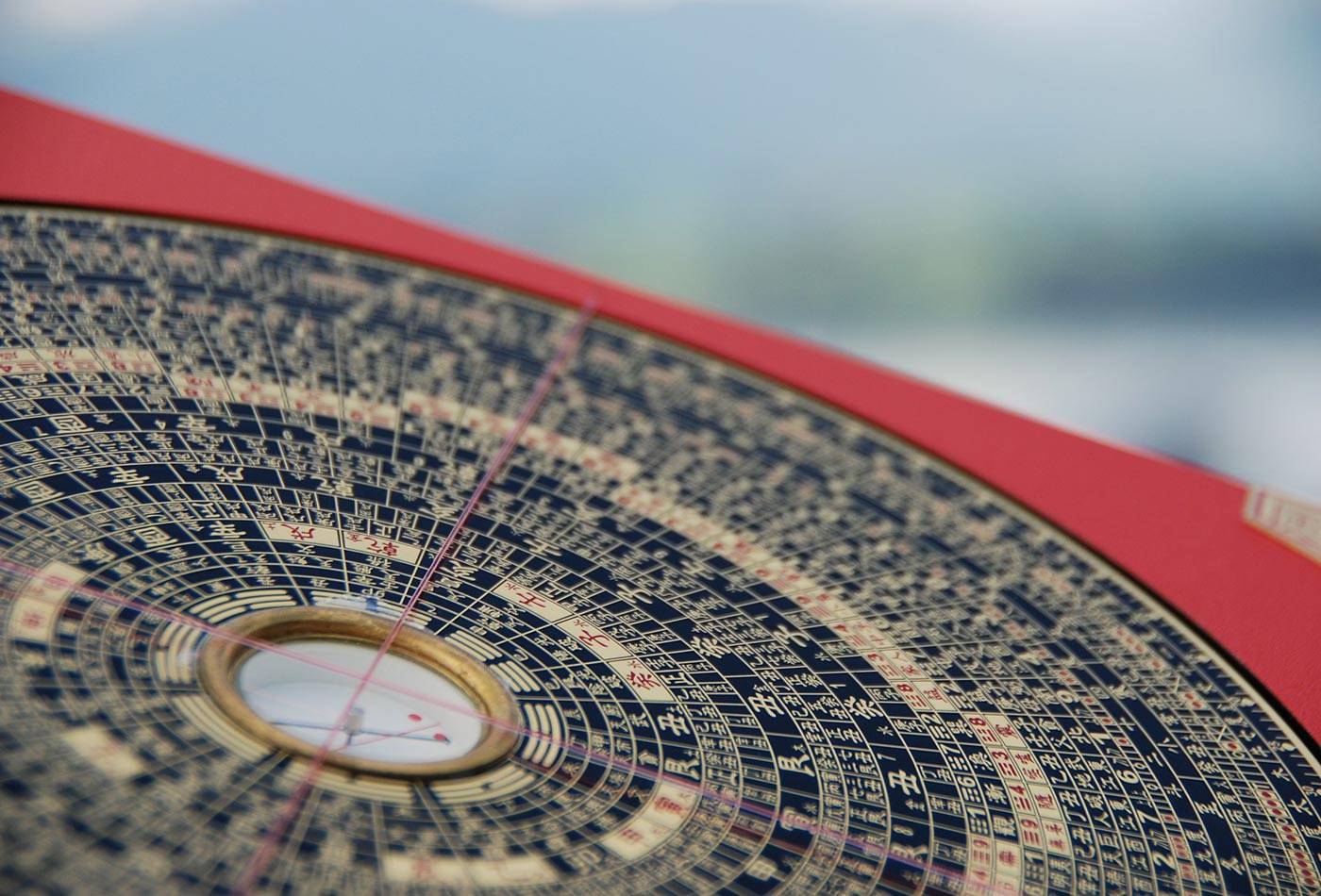
We are in an empirical era for machine learning, and it’s important to be able to identify tools that enable efficient experimentation with end-to-end machine learning pipelines. Organizations that are using and deploying machine learning are confronted with a plethora of options for training models and model inference, at the edge and on cloud services. To that end,MLPerf, a new set of benchmarks compiled by a growing list of industry and academic contributors,was recently announced at the recent Artificial Intelligence conference in NYC.
History lessons learned
2017 Turing Award winner David Patterson gives a brief overview of the 40-year history of computing benchmarks: he lists fallacies and lessons learned and he describes some previous industry cooperatives and consortiums. He closes by describing MLPerf’s primary goals
- Accelerate progress in machine learning via fair and useful measurement
- Serve both the commercial and research communities
- Enable comparison of competing systems yet encourage innovation to improve the state-of-the-art ML
- Enforce reliability to ensure reliable results
- Keep benchmarking effort affordable (so all can play)
Fathom machine learning models
Gu-Yeon Wei of Harvard University describes Fathom—a suite of eight diverse machine learning models—that attempted to serve as reference workloads for modern deep learning methods. Fathom aimed to capture the diversity of workloads that come into play when building deep learning models. Machine learning is a fast moving field, and new models arise constantly, so the eight benchmarks that were part of Fathom represented a snapshot in time. Inspired by Fathom, in early 2018, a group of industry and academic researchers drew up an initial list that became MLPerf version 0.5 benchmarks:
- Computer vision:
- Image classification
- Object detection
- Language/Audio:
- Speech recognition
- Translation
- Commerce:
- Recommendation
- Sentiment Analysis
- Action:
- Reinforcement Learning