This company uses AI to accelerate drug discovery
Atomwise applies deep learning and AI to hasten drug discoveries.
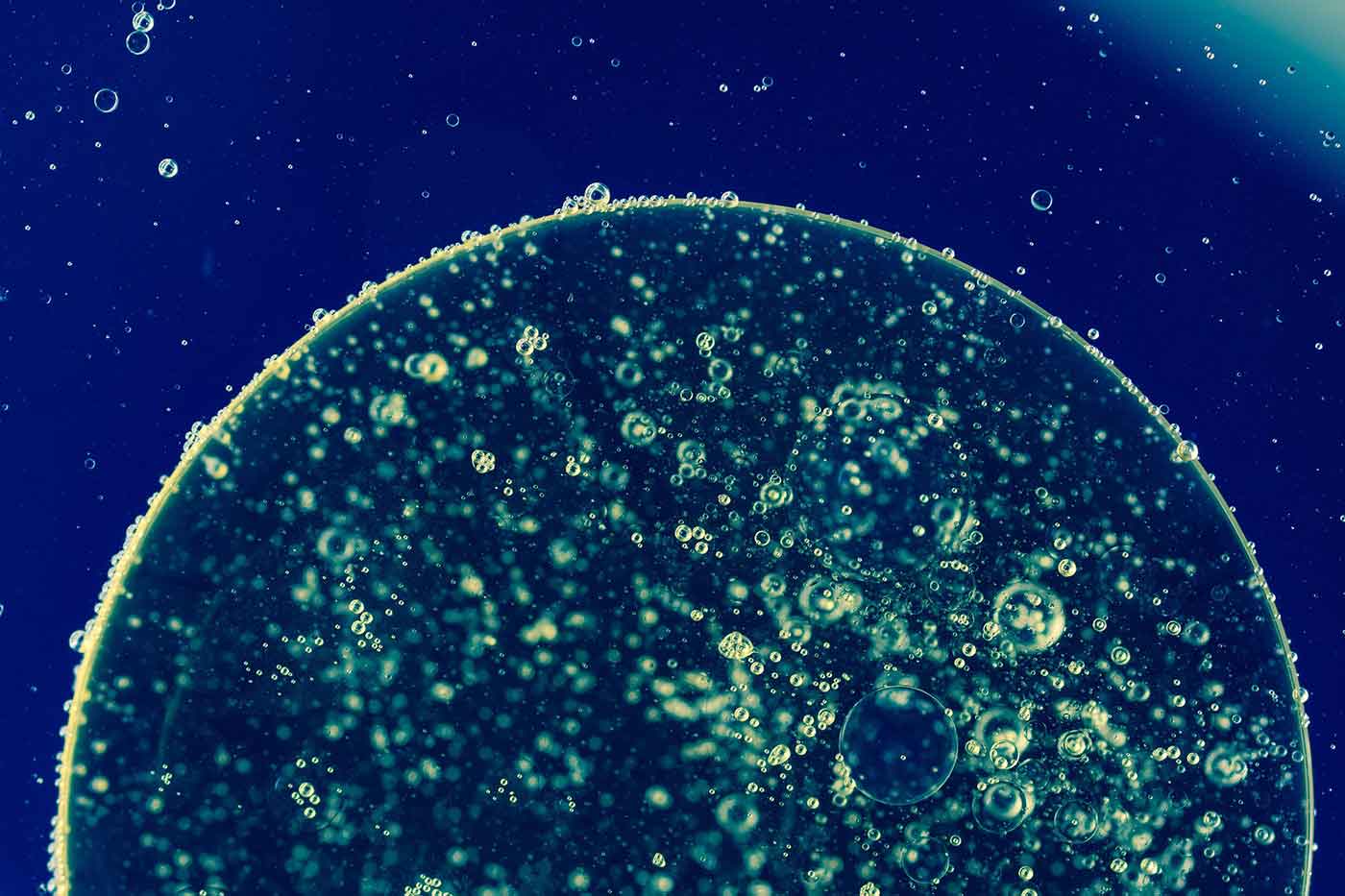
Predicting Cures
It’s hardly news that drug development is a horrendously expensive undertaking, one requiring painstaking physical synthesis and testing. That’s because the process demands a massive commitment of equipment, time, and human labor; there’s no other way, it seems, to assure newly identified molecules will properly address specific disorders without causing unacceptable side effects. Though it exists on the nano scale, the biochemical realm is vast in its granularity, and exploring and confirming its configurations is a Herculean task.
Correct that: there was no other way. In recent years, dramatic advancements in AI and machine learning have pointed to an alternative path, one that leads out of the wet lab to a virtual arena where the potential efficacy of candidate drugs can be predicted with a high degree of accuracy, greatly reducing the time necessary for the development, testing, and dissemination of new medicines.
“On average, it takes 14 years and about $2 billion to discover and refine a new drug, take it through clinical trials, and get it into pharmacies,” says Abraham Heifets, the CEO of San Francisco–based Atomwise. “And for every hundred projects that are initiated, only a few ultimately are approved, but the costs must be covered for every one of the failures. We have to do better. We have to reduce the number of flameouts.”
Atomwise uses proprietary deep-learning algorithms and supercomputers to do just that: search for potentially beneficial compounds at the rate of millions of analyses per day.
“We aren’t claiming that what we do is a substitute for clinical testing,” says Heifets. “A good analogy is aircraft design. Computer simulations will tell you relatively quickly if your idea is practical in terms of basic benchmarks, but you’re not going to just build a plane from the simulations and start flying people from coast to coast. You’re still going to test any wing you design in a wind tunnel. We see our job as winnowing out the likely failures and identifying the best candidates for testing.”
Heifets notes that two main issues must be addressed in the development of any drug: efficacy and toxicity. But the relationship between the two isn’t clear-cut. It’s a palette of many shades of gray rather than a chiaroscuro of black and white. Many chemotherapy drugs, for example, are highly toxic, but they may be effective in treating specific cancers. The challenge lies in creating compounds that produce desired results with minimal side effects.
Heifets compares proteins in the human body to machines on an assembly line. Each such “machine” will transform a given input and pass it on to another proteinaceous machine. Diseases can occur, Heifets observes, when a protein fails to perform its assigned task, or messages are somehow garbled.
“Say cell division function is expressed but not turned off,” Heifets says, “so you end up with a tumor. We see our job as identifying the machines that are running poorly, and monkey wrenching them–blocking their function. Ideally, you want a monkey wrench that fits the configuration of any given rogue protein really well so it chomps on it for a long time. But you want that wrench to work only for the target protein. You want all other proteins to remain unaffected. When they are affected, it’s expressed as ‘toxicity’ or ‘side effects.’ So the goal is to produce molecules that stick really well to the bad protein, but leave all other proteins alone.”
Coming up with candidate monkey wrenches doesn’t necessarily mean starting from scratch. Atomwise researchers spend a lot of their time trying to repurpose or reposition existing drugs, evaluating them for applications other than their original purpose.
“All medicines have side effects, but not all side effects are necessarily bad,” says Heifets. “Some are beneficial. Aspirin was originally marketed as a headache remedy, but later it was discovered it also had applications for heart disease. Viagra was first promoted as a treatment for hypertension; then it was determined it could counter sexual dysfunction. We’ve found that exploring alternative applications for existing medicines can be both productive and cost-effective.”
Atomwise’s most dramatic work to date involves Ebola.
“We got involved with an Ebola project in 2014, right when the pandemic was raging,” says Heifets. “Everybody was in emergency mode, and we coordinated with a structural virologist to identify the mechanism that Ebola uses to invade healthy cells. Once we determined that, we started thinking of ways to block entry. There was no time to launch a full discovery process—we couldn’t go through the usual 14-year cycle on this. So we started looking at promising molecules that already had human safety data that had been through Phase II or later human trials.”
Atomwise’s researchers then ran analyses and came up with a short list of molecules that looked like good Ebola-inhibiting candidates.
“We tested just the top rankings of that list,” Heifets says, “and we found two molecules that blocked Ebola entry very effectively.”
The Atomwise team then conducted a pseudovirus-based inhibition assay to determine the molecules’ efficacy in stymieing Ebola.
“That turned out very promising,” “Heifets says, “as did subsequent tests of cell metabolism to make sure the cells were staying healthy. So now we’re looking for a BSL-4 facility to run tests using the live virus and mice. Bottom line: We’ve cut that 14-year research cycle down massively. That’s incredibly heartening, and not just for Ebola. This project translates to all other infectious diseases. Just look at the list of pandemics since 2000: Ebola, SARS, Monkeypox, H1N1 influenza. These pathogens are constantly evolving, and pandemics will inevitably be part of our future. So we need better tools that will allow us to dramatically shorten the drug discovery and approval timeline.”
Heifets says algorithm-and-supercomputer–driven research will also play a crucial role in “addressing diseases that we thought we had cured but are coming back—like tuberculosis. WHO recently announced that TB has surpassed HIV as the world’s number one infectious disease. We’re in this Red Queen’s race with TB, and we need to do everything we can to improve our advantage.”
Orphan diseases will also benefit from AI and machine learning, Heifets maintains.
“There are many rare diseases that have never had effective medicines because it has been hard to justify big R&D budgets from a business standpoint,” Heifets says. “But our methods could provide medicines both quickly and relatively cheaply. And it’s here that DIY bio and advocacy groups play a particularly active role, both by bringing attention to the issues and helping with the research. We’ve partnered with some of these organizations, and they’re highly motivated. They’re doing good work, and we want to do everything we can to support them.”
Heifets takes pains to emphasize that Atomwise’s basic mission is not new. Rather, he says, the company’s successes are the fruition of decades of work by hundreds of researchers, most of whom remain unsung. The potential of computer technology in determining disease pathways and predicting effective remedies has been recognized for 40 years, says Heifets, but the machines and data that were available for most of that period weren’t up to the task.
“Now, finally, the technology is catching up with our ambitions,” he says. “But we have to remember we didn’t create this by ourselves. We’re standing on the shoulders of giants.”