Making AI transparent
The O'Reilly Podcast: Andy Hickl on sources of bias in artificial intelligence—and how to address them.
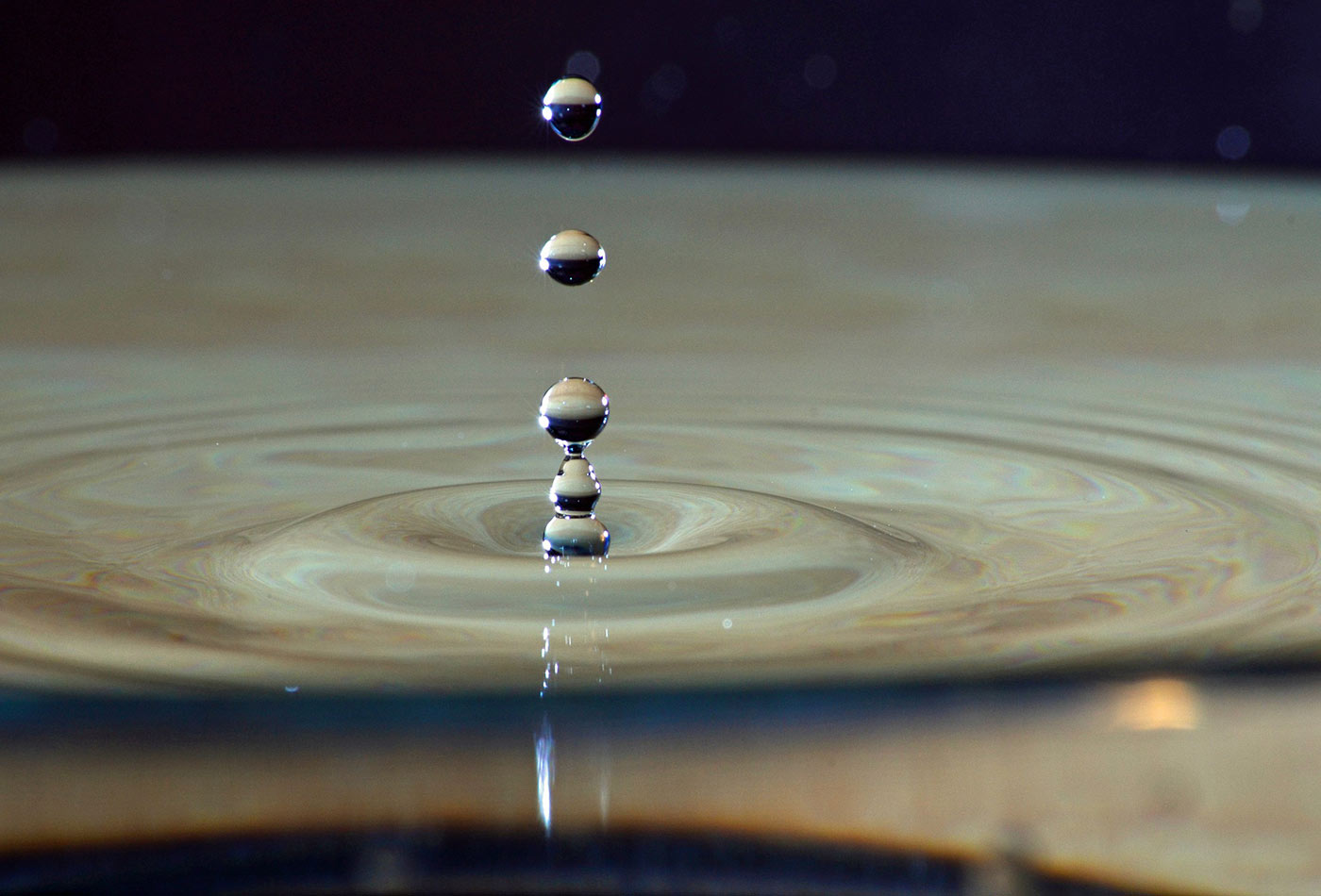
Over the next several years, artificial intelligence techniques are likely to make their way into a wide variety of critical processes, such as diagnosing illnesses and deciding whether to underwrite risky insurance policies. Ensuring that AI algorithms are acting on accurate, unbiased assumptions is essential. Executives, policymakers, and consumers will ask how these important judgments are made, but they’ll run into a problem: many AI techniques involve “black boxes” that may be highly accurate, but which aren’t interpretable by humans in intuitive ways.
In this podcast episode, I speak with Andy Hickl, chief product officer at Intel Saffron Cognitive Solutions Group. Our subject: common sources of bias in artificial intelligence—and how to identify and address them by using transparent AI methods.
AI systems automatically learn the characteristics of the data they’re trained with. In the process, they often learn patterns that aren’t the best possible representations of ground truth—either because they’re trained with an unrepresentative data sample, or because they learn to pick up higher-order signals that turn out to be false leads. And unless these models are easily interpretable by human operators, these biases may go undetected.
So, how should managers address the problem of bias in AI? One answer, says Hickl, is transparency. Models that can explain their decision-making process make it possible for human operators to detect bias and logical errors. In natural language understanding and computer vision, researchers have developed attentional and memory-based models that illustrate which elements of an image or sentence bore most heavily on the model’s judgment.
For instance, notes Hickl, a classifier trained to distinguish between photos of baseball games and photos of soccer games might identify the presence of bats and baseball caps as the most salient features in deciding that a photo shows a baseball game. Or, an AI system trained to identify modes of industrial failure could point to three different factors that make a machine likely to fail.
An AI system “can’t just say, ‘hey, trust me, I’m right. I’m a machine and I know more than you do,’” says Hickl. “It’s got to be able to establish a worldview.”
Links to research that Hickl mentions in the podcast:
- “Man is to Computer Programmer as Woman is to Homemaker? Debiasing Word Embeddings,” by Tolga Bolukbasi, Kai-Wei Chang, James Zou, Venkatesh Saligrama, and Adam Kalai
- Project Aristo at the Allen Institute for Artificial Intelligence, led by Peter Clark, aims to answer multiple-choice questions from grade-school science exams
This post and podcast is a collaboration between O’Reilly and Intel. See our statement of editorial independence