Charles Givre on the impetus for training all security teams in basic data science
The O’Reilly Security Podcast: The growing role of data science in security, data literacy outside the technical realm, and practical applications of machine learning.
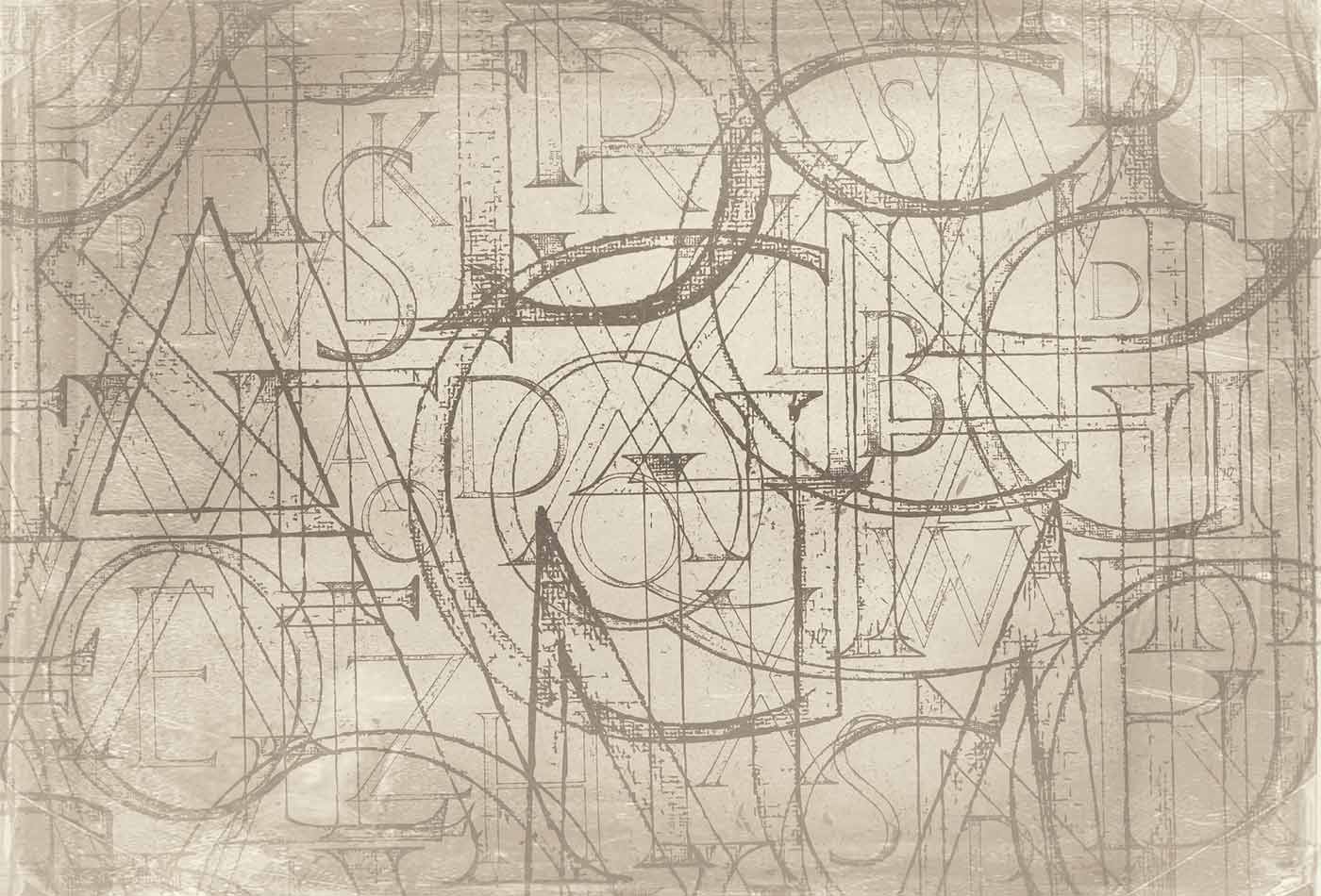
In this episode of the Security Podcast, I talk with Charles Givre, senior lead data scientist at Orbital Insight. We discuss how data science skills are increasingly important for security professionals, the critical role of data scientists in making the results of their work accessible to even nontechnical stakeholders, and using machine learning as a dynamic filter for vast amounts of data.
Here are some highlights:
Data science skills are becoming requisite for security teams
I expect to see two trends in the next few years. First, I think we’re going to see tools becoming much smarter. Not to suggest they’re not smart now, but I think we’re going to see the builders of security-related tools integrating more and more data science. We’re already seeing a lot of tools claiming they use machine learning to do anomaly detection and similar tasks. We’re going to see even more of that.
Secondly, I think rudimentary data science skills are going to become a core competency for security professionals. Considering, I expect we are going to increasingly see security jobs requiring some understanding of core data science principles like machine learning, big data, and data visualization. Of course, I still think there will be a need for data scientists. Data scientists are going to continue to do important work in security, but I also think basic data science skills are going to proliferate throughout the overall security community.
Data literacy for all
I’m hopeful we’re going to start seeing more growth in data literacy training for management and nontechnical staff, because it’s going to be increasingly important. In the years to come, management and executive-level professionals will need to understand the basics—maybe not a technical understanding, but at least a conceptual understanding of what these techniques can accomplish.
Along those lines, one of the core competencies of a data scientist is, or at least arguably should be, communication skills. I’d include data visualization in that skillset. You can use the most advanced modeling techniques and produce the most amazing results, but if you can’t communicate that in an effective manner to a stakeholder, then your work is not likely to be accepted, adopted, or trusted. As such, making results accessible is really a vital component of a data scientist’s work.
Machine learning as a dynamic filter for security data
Machine learning and deep learning have definitely become the buzzwords du jour of the security world, but they genuinely bring a lot of value to the table. In my opinion, the biggest value machine learning brings to the table is the ability to learn and identify new patterns and behaviors that represent threats. When I teach machine learning classes, one of the examples I use is domain-generating algorithm detection. You can do this with a whitelist or a blacklist, but neither one of these is going to be the most effective approach. There’s been a lot of success in using machine learning to identify this, allowing you to then mitigate the threat. A colleague of mine, Austin Taylor, gave a presentation and wrote a blog post about this as well—about how machine learning fits in the overall schema. He views data science in security as being most useful in building a very dynamic filter for your data.
If you imagine an inverted triangle, you begin examining tons and tons of data, but you can use machine learning to filter out the vast majority of it. From there, a human might still have to look at the remaining portion. By applying several layers of machine learning to that initial ingested data, you can efficiently filter out the stuff that’s not of interest.