Associative memory AI aids in the battle against financial crime
The O’Reilly Media Podcast: Gayle Sheppard, Saffron AI Group at Intel, and David Thomas, Bank of New Zealand.
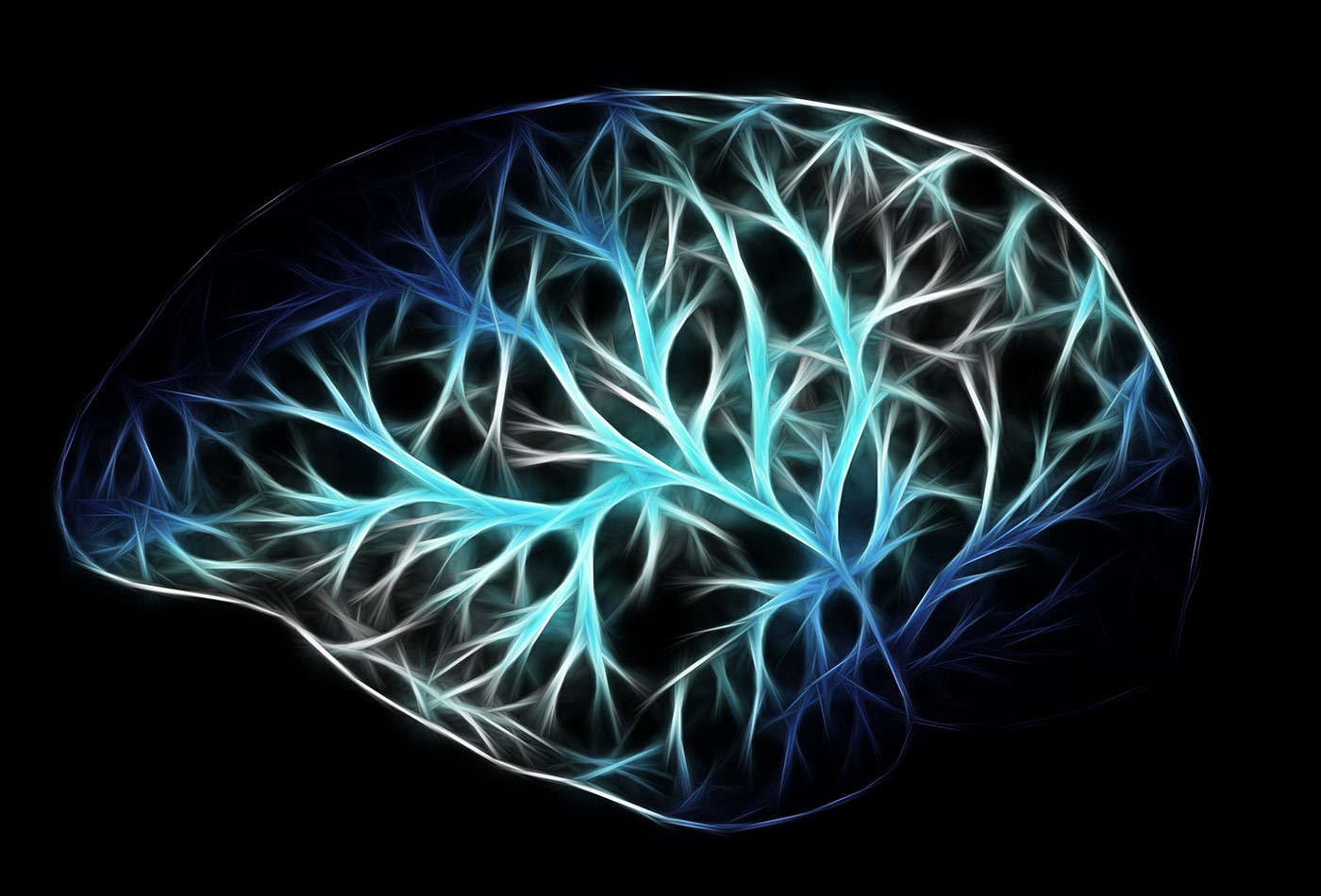
In this episode of the O’Reilly Media Podcast, I spoke with Gayle Sheppard, vice president and general manager of Saffron AI Group at Intel, and David Thomas, chief analytics officer for Bank of New Zealand (BNZ). Our conversations centered around the utility of artificial intelligence in the financial services industry.
Associative memory AI: Enabling machines to fight financial crime with human-like reasoning
According to Sheppard, associative memory AI technologies are best thought of as reasoning systems that combine the memory-based learning seen in humans—recognizing patterns, spotting anomalies, and detecting new features almost instantly—with data. Compared to traditional machine learning methods, Sheppard says, associative memory AI unifies multiple data sources—both structured and unstructured—without relying on pre-defined models. Associative memory AI reasons on that data to deliver insights quickly, accurately, and with less training data—in some cases, using as little as 20% of the available training set. Furthermore, transparency is built into the fabric of associative memory AI, so one can more easily explain the system’s path to insight.
Applications of associative memory AI in the enterprise are varied. “Our strategy is to build comprehensive decision systems for financial services, supply chain management, and manufacturing and defense. … These systems combine what we think are the best of learning approaches, such as deep learning, traditional statistical machine learning, associative learning, and others. Our goal is to deliver a sum that is much greater than its individual parts.” Intel has developed a sharp focus on the financial services industry, with its October launch of the Intel Saffron Anti-Money Laundering (AML) Advisor. Sheppard described four challenges and opportunities that Intel sees in the financial services industry:
- Financial institutions—specifically banks and insurers—collect data at massive scale, and this data is expected to double every two years. Human and machine-generated data is growing 10 times faster than traditional business data.
- Structured, transactional data has dominated their systems, but almost everything in banking is customer-pattern based, or unstructured. Excluding this data from analysis significantly reduces the relevance of its outcomes.
- Models become stale quickly, but criminals are constantly evolving. It can take up to nine months to update statistical models, from design to test, for banking customers. That’s before these new models can even be put into production. “The fastest time for the industry to deploy simple model changes is considered five to six months. That’s a lot of time for crime to run rampant for these institutions,” said Sheppard.
- Financial organizations can store data on several hundred systems of record. How can customers efficiently access and analyze this data that’s spread across multiple locations? It’s incredibly time consuming and expensive to move that data, particularly in an industry governed by tight regulations.
If associative memory AI can address these four key challenges, then where does that leave investigators, analysts, and auditors—the human decision-makers? As Sheppard explains, AI technologies go to work for the human element. They find similarity/anomaly/novelty patterns, make recommendations and predictions, and provide explanations behind their insights in order to make the human decision better, faster, and more confident. “This full investigative analytic capability that we can provide for humans plus the evidence to explain why we believe a certain course of action should be taken is a terrific productivity aid to the human investigator.”
Taking banking into the future with lean analytics and AI
Thomas extended the narrative by talking about the evolving business model for financial services, how banks are navigating increasingly strict regulations, and how BNZ is leveraging data and modern tools to engage with its customers. We discussed the opportunities for AI in this new landscape, and how BNZ is using natural language processing, cognitive computing, and neural networks to empower their customers to meet their financial goals. Thomas noted that Intel has been instrumental in not only configuring BNZ’s architecture, but also in thinking about their customer strategy. BNZ is participating in Intel Saffron’s Early Adopter Program, which was recently launched with the goal of targeting institutions that aim to lead the pack on innovation in financial services.
Below are some highlights from our conversation:
Insights from unstructured data
I think the use of unstructured data is increasing at quite a pace. Historically, we have very structured data, but we’ve now set up our Cloudera Hadoop-based data lake, are starting to use natural language processing more and more, and are utilizing bots to understand what our customers are saying. The insight you can get from what customers have actually said as opposed to what they’ve said in research or in a survey, just takes it to a whole new level of getting closer to the customer.
Proven approaches
In terms of the tools, probably the things we’ve tried the most that we’ve really appreciated are: we have changed some operating models (we are using lean analytics, which is a lot of hypothesis-driven work, utilizing data scientists but combining with customer experience teams), taking off bite-sized customer problems or customer opportunities, and completing three-week sprints. That’s been really successful for us. If you can do a three-week sprint and come out with eight or nine prototype interventions that have been tested with customer data, you can move the organization at quite a pace. We also have started developing bots and playing with those on both the sales and service side, and we’re investing heavily in our digital teams, supported by analytics and thinking quite a lot about the global evolution toward digital banks, and how you blend that with the human interactions as well.
Navigating the pace of change
The first thing we had to do, particularly working with the board, is define what AI is. … And we did need to present it as almost a progression and broken into chunks—natural language processing, cognitive computing, neural networks, and machine learning. … The biggest lesson we’ve certainly had as an organization is that information or data insights or whatever variation you call it, has got to be an ongoing build. All of our strategies look like staircases. We’re very, very mindful that pace of change is substantial and we need partners to stay up with that change, but we need to be constantly moving forward.
This post is a collaboration between O’Reilly and Intel Saffron. See our statement of editorial independence.