Aman Naimat on building a knowlege graph of the entire business world
The O'Reilly Radar Podcast: The maturity of AI in enterprise, bridging the AI gaps, and what the U.S. can do with $4 trillion.
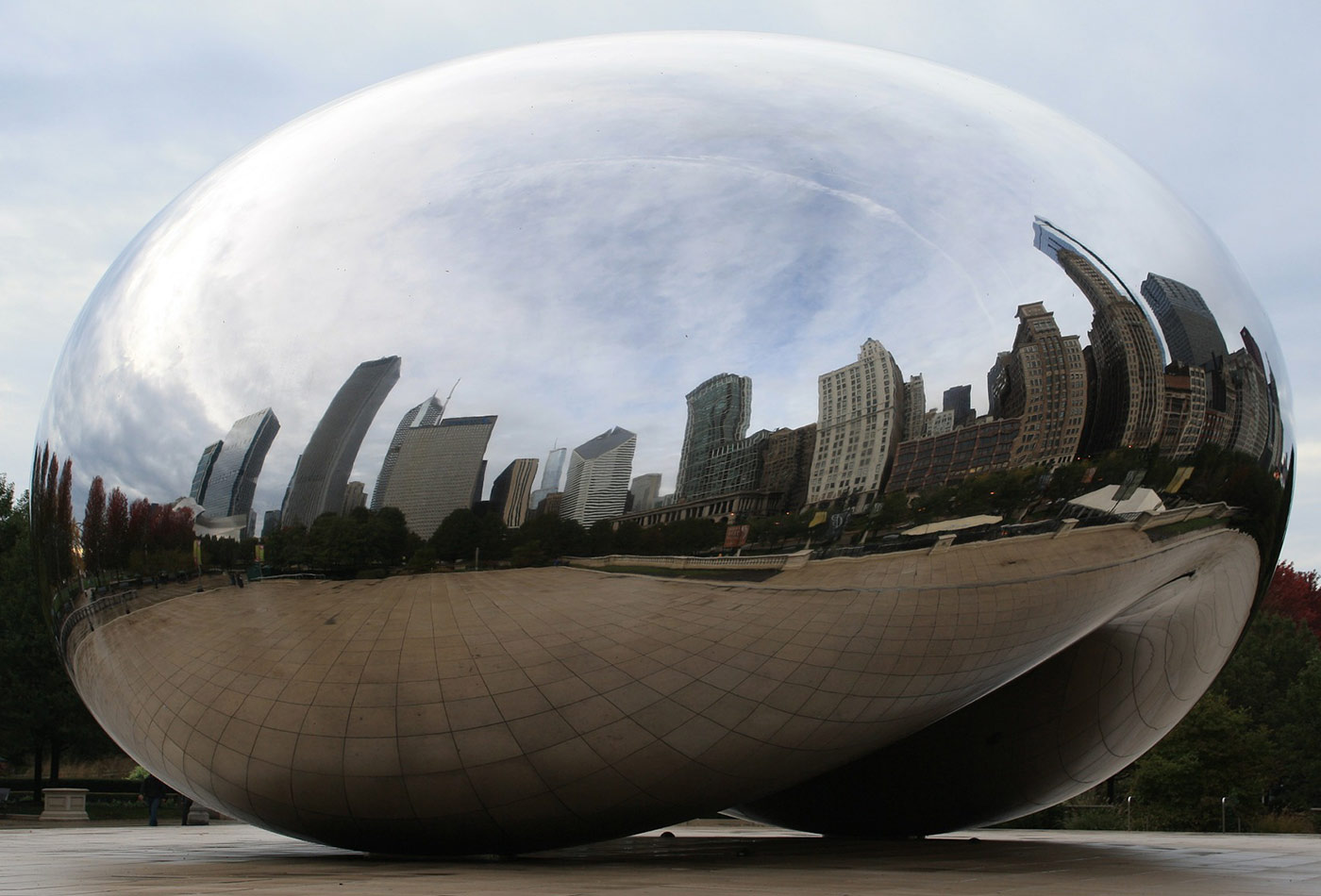
This week, I sit down with Aman Naimat, senior vice president of technology at Demandbase, and co-founder and CTO of Spiderbook. We talk about his project to build a knowledge graph of the entire business world using natural language processing and deep learning. We also talk about the role AI is playing in those companies today and what’s going to drive AI adoption in the future.
Here are a few highlights:
Surveying AI adoption
We were studying businesses for the purpose of helping sales people talk accounts, and we realized we could use our technology to study entire markets. So, we decided to study entire markets of how companies are adopting AI or big data. Really, the way it works is, we built a knowledge graph of how businesses interact with each other, their behavioral signals, who’s doing business with whom, who are their partners, customers, suppliers? Who are the influencers, the decision-makers? Who’s buying what product?
In essence we have built a universal database, if I may, or a knowledge graph, of the entire business world. We use natural language processing and deep learning—the short answer for what data sets we look at is everything. We are now reading the entire business internet, completely unstructured data, from SCC filings to financial regulatory filings to Tweets to every blog post, every job post, every conference visit, every power point, every video. So, it’s really pretty comprehensive. We also have a lot of proprietary data around the business world, as to who’s reading or viewing what ad, and we triangulate all of that in this graph and do machine learning on top to classify maturity levels of each company out of the 500,000 into how mature they are in AI. How many people do they have working, what are they doing with it, what are the use cases, how much money are they spending. That’s how we built the study.
Bridging the AI gap between academia and enterprise
What will drive adoption in AI, I think, is also investment. The current landscape, according to our study, which was the first data-driven study of the market, was that only a few companies are really investing in it. There’s some interest in other places, but companies like Google—the CEO recently came out and said that AI is really how the company will be framed going forward. So, we need more investments, more venture capital investments, more government investments, and that’s not just in starting startups, but putting together data sets that data scientists could consume. Public data sets is a huge gap in the market between what is available in academia and what companies like us at Demandbase have—we have a ton of data, proprietary data. So, to be able to have such data available in open source…that could spark new types of use cases.
Can we build an AI-based representative democracy?
Another use case: the largest set of spend in the world is actually the United States government—$4 trillion; it’s a huge market. So, how do you allocate those resources? Is it possible that we can build systems that, in essence, become some sort of an AI-based representative democracy where we can optimize the preferences of individual citizens?
Today, most citizens are completely unaware of what’s happening at their local government level or state level. If I ask you who’s your state senator, you probably don’t know. Nobody actually does, yet the state level pretty much has the biggest impact on our lives. They control education, roads, environment, and they have some of the largest budgets— health care. There’s suddenly areas where we can try to understand individual preferences automatically, and there’s a lot of data—for each bill that is passed, there are thousands and thousands of pages of feedback, text, that AI can process and understand. So, obviously some of this is really far out, but that doesn’t mean we can’t do something today.