5 tips for embracing open data science in the enterprise
Transform the way you approach analytics.
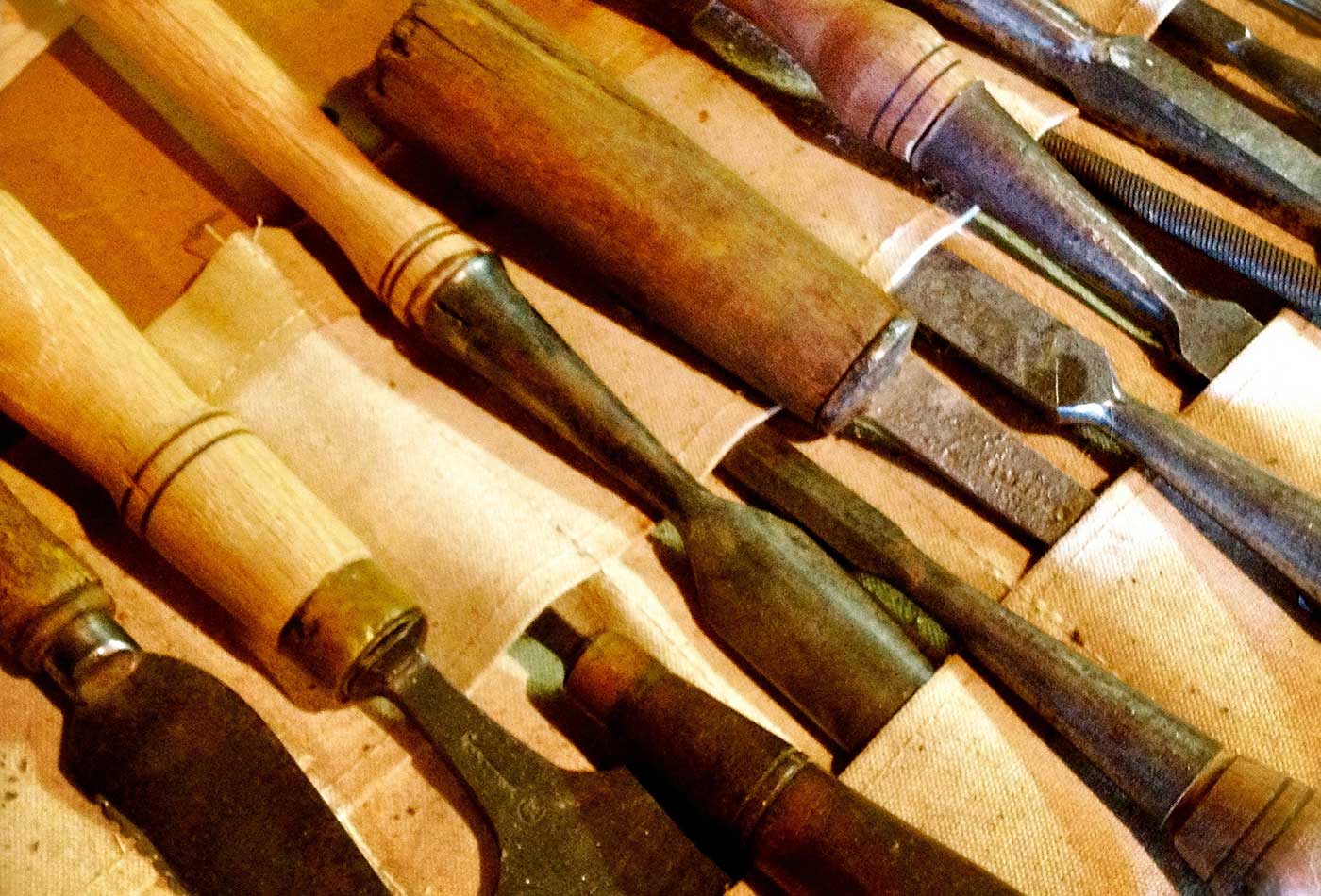
Businesses are continually seeking competitive advantage. Lately, the focus has been on leveraging data to seize opportunities, detect possible weaknesses, and triumph over competitors. Big data, in particular, offers a multitude of ways to use data to drive strategic, operational, and execution practices. And, increasingly, data science is the way to get there.
First, a definition: data science is a multidisciplinary field that combines the latest innovations in advanced analytics—including machine learning and artificial intelligence—with high-performance computing and visualizations to extract knowledge or insights from data.
The tools of data science originated in the scientific community, where researchers used them to test and verify hypotheses that include “unknown unknowns.” These tools have entered business, government, and other organizations gradually over the past 10 years as computing costs have dropped and software has grown more sophisticated.
But proprietary tools and technologies have proved to be inadequate to support the speed and innovation happening in the data science world. Enter the open source community.
Open source communities want to break free from the shackles of proprietary tools and embrace a more open and collaborative work style that reflects the way they work—with teams distributed all over the world. These communities are not just creating new tools; they’re calling on enterprises to use the right tools for the problems at hand.
Open data science is revolutionary. It transforms the way organizations approach analytics. With open data science, you can boost the productivity of your data team, enhance efficiencies by moving to a self-service data model, and overcome organizational and technical barriers to making the most of your big data.
Here are five things you can do to embrace open data science:
- Wholeheartedly adopt open source. Traditional commercial data science tools evolve slowly. Although stable and predictable, many of them have been architected around 1980s-style client-server models that don’t scale to internet-oriented deployments with web-accessible interfaces. On the other hand, the open data science ecosystem is founded on concepts of standards, openness, web accessibility, and web-scale-oriented distributed computing. And, open data science tools are created by a global community of analysts, engineers, statisticians, and computer scientists who have hands-on experience in the field.
This global community includes millions of users and developers who rapidly iterate the design and implementation of the most exciting algorithms, visualization strategies, and data processing routines available today. These pieces can be scaled and deployed efficiently and economically to a wide range of systems.
By enthusiastically adopting—and contributing to—this community, your chances of having successful deployments multiplies exponentially.
- Build a data science team with diverse skills. Successful projects start with gathering together the right people and organizing them in a way that makes operational sense. Open data science is no different, but the diverse range of skills required might surprise you. True, data science inherently rests on mathematics and computer science. A strong statistical background has traditionally been assumed necessary for one to work in data science. However, these magical “data scientist” unicorns are very difficult to find. Moreover, open data science is a practical real-world discipline that requires a team that includes business analysts, data scientists, developers, data engineers, and devops engineers.
It also requires new organizational structures—centers of excellence, lab teams or emerging technology teams are a way to dedicate personnel to jump-start the changes. These groups are typically charged with actively seeking out new open data science technologies and determining the fit and value to the organization. This facilitates adoption of open data science and bridges the gap between traditional IT and lines of business. Additionally, roles may shift—from statistician to data scientist, and from database administrator to data engineer—and new roles, such as computational scientist, will emerge. It pays to be flexible and to welcome diversity.
- Secure executive sponsorship. This might sound like your standard IT-projects-need-executive-sponsorship spiel. But keep in mind that we’re talking about making room in the enterprise IT landscape for an emerging world where open data science connects with new and existing data to inform everything from ordinary day-to-day to critically important strategic business decisions. Also, open data science introduces new and different types of risks into the organization that can be mitigated by appropriate executive sponsorship.
- Prepare for dynamic spending. With traditional analytics software, when you purchase a platform or system, all your spending decisions are made upfront. You are effectively wedded to that decision for some time. And then you get what you get. This static investment is quite different than the dynamic investments that are made with open data science.
In the open data science world, you’ll have the advantage of moving faster and getting things up and running more quickly, as the open source software is freely available for people to download and start using right away. No need to wait for corporate purchasing cycles. Neither do you have to wait for the long upgrade cycles of commercial software, as the brightest minds around the world are continuously contributing to open source software innovation, and their efforts are made instantly available. That’s a definite plus. Less up-front big planning and big budgeting is needed. But you do have to continually make new choices and new investments, as your needs—and the technology—evolve. This requires making some organizational process changes in budgeting and procurement.
- Put robust governance frameworks in place. Open data science doesn’t exist in a vacuum. You will still need to exercise control over creating, sharing, and deploying data science assets in your organization. The user permissions you establish for your data science assets must integrate with a wide variety of enterprise authentication systems, such as LDAP, Active Directory, and Kerberos, to track all open data science activities. This includes access to specific versions of open source libraries and packages, plus specific versions of the data science assets created by your team. Additionally, you need to build a full provenance of the data science assets (for example, data, models, and apps) to achieve the transparency often demanded by regulators or compliance review boards.
The pace of business today demands responsive data science collaboration from empowered teams with a deep understanding of the business that can quickly deliver value. They also require the right open data science tools—and increasingly, that’s a wide array of programming languages, analytic techniques, analytic libraries, visualizations, and computing infrastructure.
Open data science is truly revolutionary, and has the chance to change business decision-making as we know it.
This post is part of a collaboration between O’Reilly and Continuum Analytics. View our statement of editorial independence.